Double nuclear norm-based robust principal component analysis for image disocclusion and object detection.
Neurocomputing(2016)
摘要
Robust principal component analysis (RPCA) is usually used to remove structural error from low rank matrix. It assumes that the real data matrix has low rank and the error matrix is sparse. A new emerging method named double nuclear norm-based matrix decomposition (DNMD), which uses a unified low-rank assumption to characterize the real image data and continuous occlusions, is also applied to recover images and to model background. This paper presents a method called double nuclear norm-based robust principal component analysis (DNRPCA) for dealing with the occluded image. It not only assumes that the real data matrix has low rank, but also supposes the error matrix is sparse in vector space and each error image is a low-rank matrix, which can not only cope with the structural error but also the sparse error compared to DNMD. Experiments on removing occlusions from face images and object detection from monitoring videos demonstrate the advantages of our method compared to the state-of-the-art approaches.
更多查看译文
关键词
Low rank,Nuclear norm,Robust principal component analysis,Image disocclusion,Background modelling
AI 理解论文
溯源树
样例
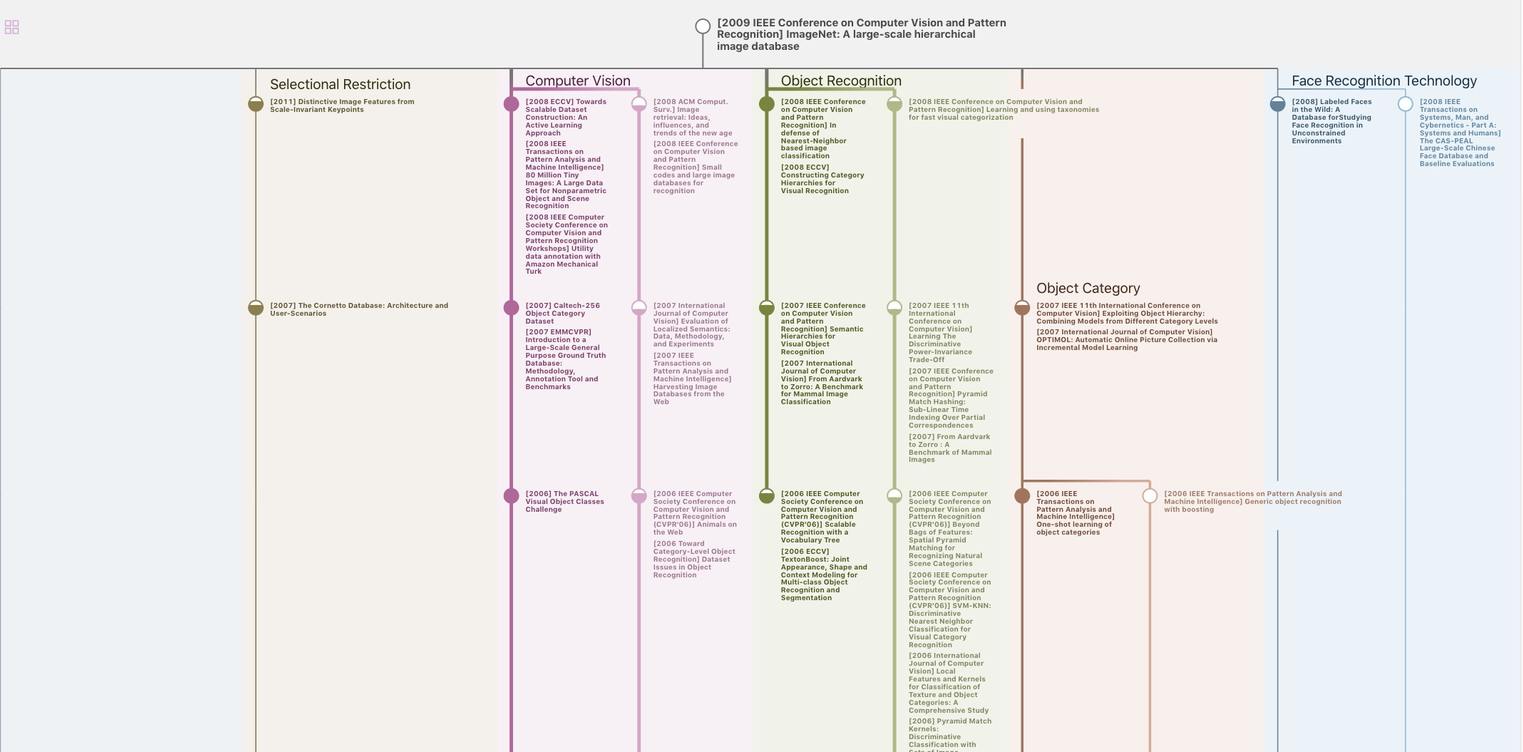
生成溯源树,研究论文发展脉络
Chat Paper
正在生成论文摘要