Random Walk with Restart on Large Graphs Using Block Elimination.
ACM Trans. Database Syst.(2016)
摘要
Given a large graph, how can we calculate the relevance between nodes fast and accurately? Random walk with restart (RWR) provides a good measure for this purpose and has been applied to diverse data mining applications including ranking, community detection, link prediction, and anomaly detection. Since calculating RWR from scratch takes a long time, various preprocessing methods, most of which are related to inverting adjacency matrices, have been proposed to speed up the calculation. However, these methods do not scale to large graphs because they usually produce large dense matrices that do not fit into memory. In addition, the existing methods are inappropriate when graphs dynamically change because the expensive preprocessing task needs to be computed repeatedly.
In this article, we propose Bear, a fast, scalable, and accurate method for computing RWR on large graphs. Bear has two versions: a preprocessing method BearS for static graphs and an incremental update method BearD for dynamic graphs. BearS consists of the preprocessing step and the query step. In the preprocessing step, BearS reorders the adjacency matrix of a given graph so that it contains a large and easy-to-invert submatrix, and precomputes several matrices including the Schur complement of the submatrix. In the query step, BearS quickly computes the RWR scores for a given query node using a block elimination approach with the matrices computed in the preprocessing step. For dynamic graphs, BearD efficiently updates the changed parts in the preprocessed matrices of BearS based on the observation that only small parts of the preprocessed matrices change when few edges are inserted or deleted. Through extensive experiments, we show that BearS significantly outperforms other state-of-the-art methods in terms of preprocessing and query speed, space efficiency, and accuracy. We also show that BearD quickly updates the preprocessed matrices and immediately computes queries when the graph changes.
更多查看译文
关键词
Proximity, random walk with restart, ranking in graph, relevance score
AI 理解论文
溯源树
样例
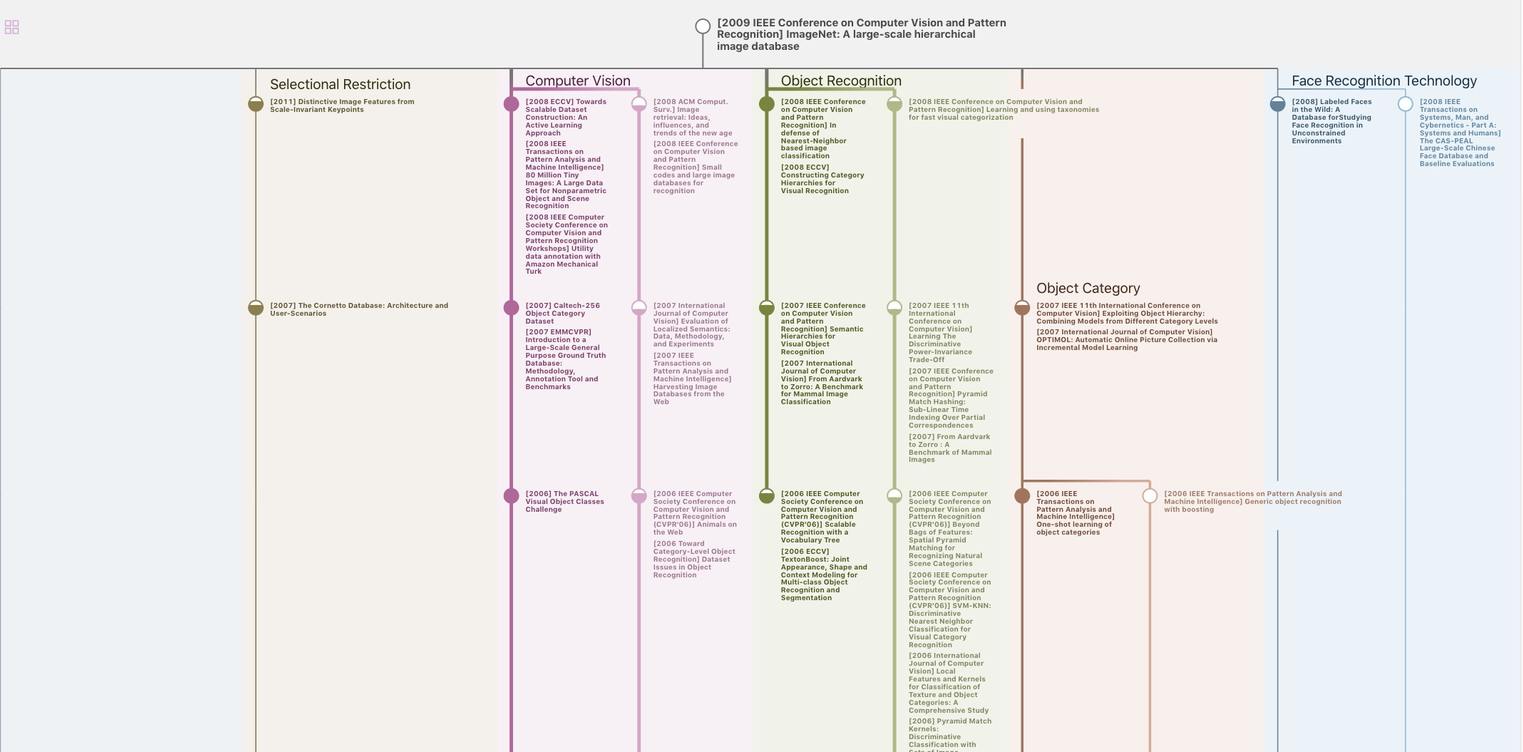
生成溯源树,研究论文发展脉络
Chat Paper
正在生成论文摘要