A Kernel-Based Clustering Method for Gene Selection with Gene Expression Data.
Journal of Biomedical Informatics(2016)
摘要
Gene selection is important for cancer classification based on gene expression data, because of high dimensionality and small sample size. In this paper, we present a new gene selection method based on clustering, in which dissimilarity measures are obtained through kernel functions. It searches for best weights of genes iteratively at the same time to optimize the clustering objective function. Adaptive distance is used in the process, which is suitable to learn the weights of genes during the clustering process, improving the performance of the algorithm. The proposed algorithm is simple and does not require any modification or parameter optimization for each dataset. We tested it on eight publicly available datasets, using two classifiers(support vector machine, k-nearest neighbor), compared with other six competitive feature selectors. The results show that the proposed algorithm is capable of achieving better accuracies and may be an efficient tool for finding possible biomarkers from gene expression data.
更多查看译文
关键词
Adaptive distance,Cancer classification,Gene expression data,Gene selection,Kernel-based clustering
AI 理解论文
溯源树
样例
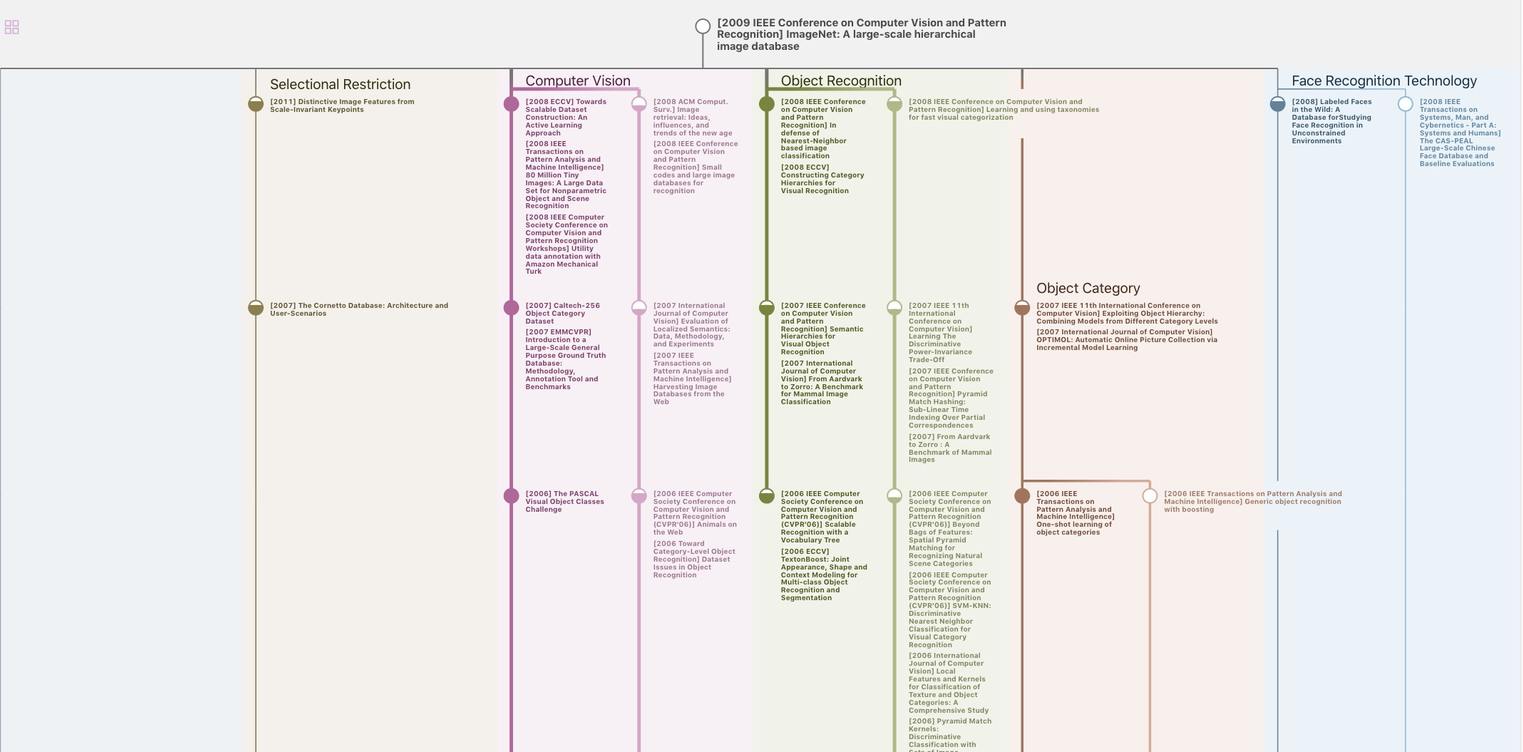
生成溯源树,研究论文发展脉络
Chat Paper
正在生成论文摘要