Complex Variants of GLVQ Based on Wirtinger's Calculus.
ADVANCES IN SELF-ORGANIZING MAPS AND LEARNING VECTOR QUANTIZATION, WSOM 2016(2016)
摘要
This paper addresses the application of gradient descent based machine learning methods to complex-valued data. In particular, the focus is on classification using Learning Vector Quantization and extensions thereof. In order to apply gradient-based methods to complex-valued data we use the mathematical formalism of Wirtinger's calculus to describe the derivatives of the involved dissimilarity measures, which are functions of complex-valued variables. We present a number of examples for those dissimilarity measures, including several complex-valued kernels, together with the derivatives required for the learning procedure. The resulting algorithms are tested on a data set for image recognition using Zernike moments as complex-valued shape descriptors.
更多查看译文
关键词
Generalized Learning Vector Quantization (GLVQ), Complex-valued Kernel, Zernike Moments, Complex Independent Component Analysis, Exponential Kernel
AI 理解论文
溯源树
样例
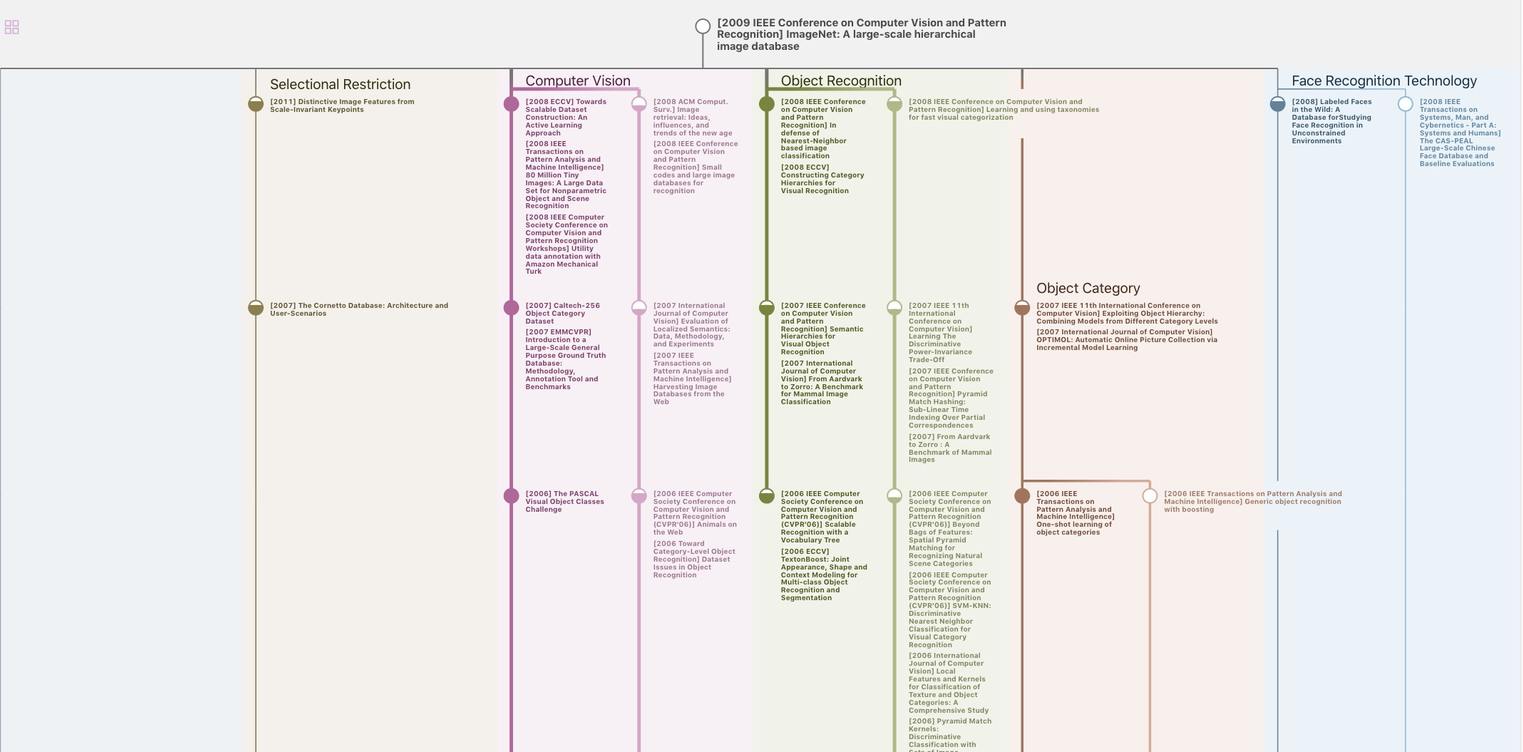
生成溯源树,研究论文发展脉络
Chat Paper
正在生成论文摘要