Adjustable adaboost classifier and pyramid features for image-based cervical cancer diagnosis
IEEE International Symposium on Biomedical Imaging(2015)
摘要
Cervical cancer is the third most common type of cancer in women worldwide. Most death cases of cervical cancer occur in less developed areas of the world. In this work, we develop an automated and low-cost method that is applicable in those low-resource regions. First, we propose a more distinctive multi-feature descriptor for encoding the cervical image information by enhancing an existing descriptor with the pyramid histogram of local binary pattern (PLBP) feature. Second, we apply the AdaBoost algorithm to perform feature selection, and train a binary classifier to differentiate high-risk patient visits from low-risk patient visits. Our AdaBoost classifier can be adjusted to achieve high specificity, which is necessary for use in clinical practice. Experiments on both balanced and imbalanced datasets are conducted to evaluate the effectiveness of our method. Our method is shown to achieve better performance than existing image-based CIN classification systems and also outperform human interpretations on various screening tests.
更多查看译文
关键词
Cervical Cancer Screening, Computer Aided Diagnosis, Image Classification, AdaBoost, Pyramid Histograms, Local Binary Patterns
AI 理解论文
溯源树
样例
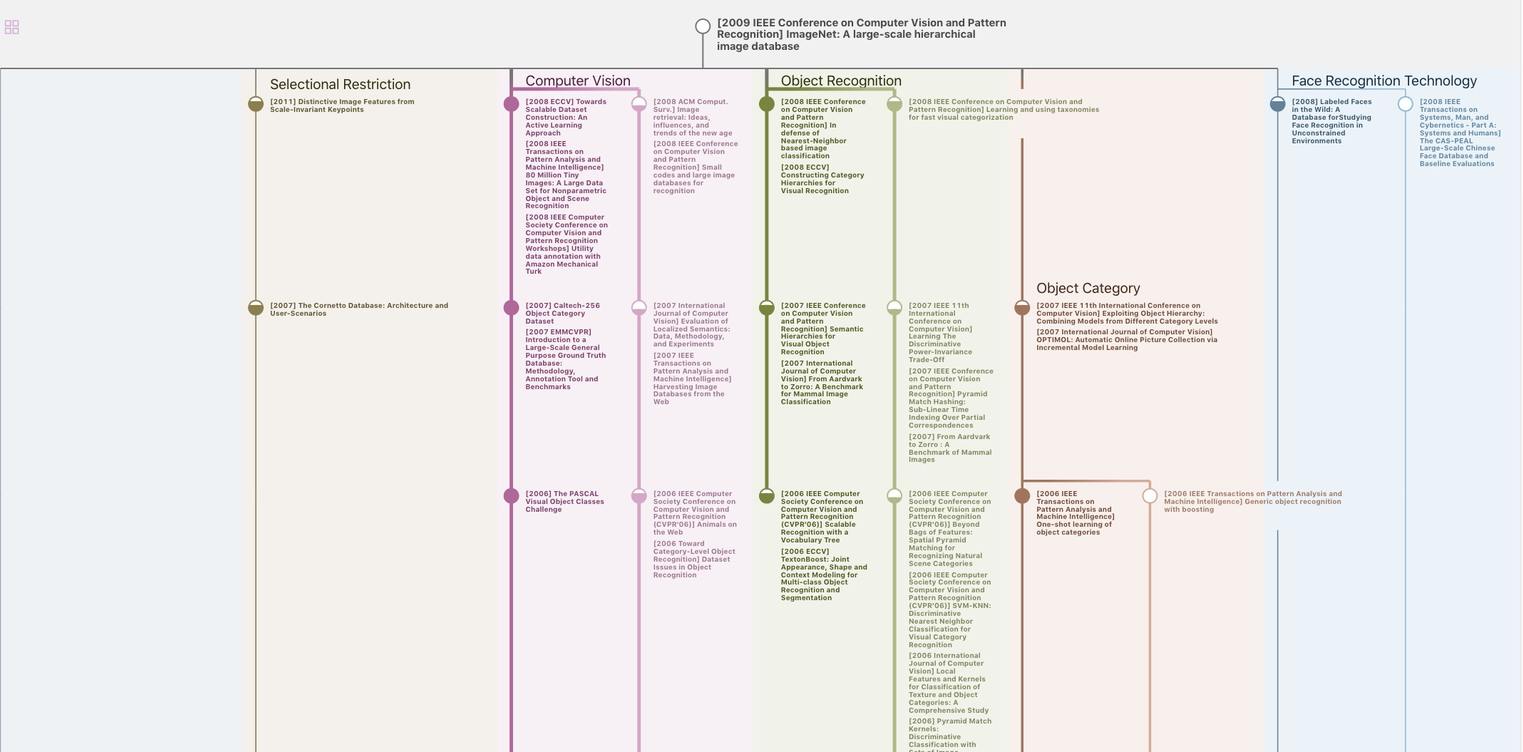
生成溯源树,研究论文发展脉络
Chat Paper
正在生成论文摘要