An Experimental Study On Combining The Auto-Context Model With Corrective Learning For Canine Leg Muscle Segmentation
2015 IEEE 12th International Symposium on Biomedical Imaging (ISBI)(2015)
摘要
Corrective learning is a technique that applies classification methods for automatically detecting and correcting systematic segmentation errors produced by existing segmentation methods with respect to some gold standard (manual) segmentation. To allow corrective learning more effectively correct errors that require non-local contextual information to capture, we extend the corrective learning technique by combining it with auto-context learning and conduct experimental study to verify its effectiveness. In our experiment, we take multi-atlas joint label fusion as the host segmentation method, for which we apply our corrective learning technique to improve, and apply it on a canine leg muscle segmentation application. We show that the auto-context enhanced corrective learning produces prominent improvement over the original corrective learning method.
更多查看译文
关键词
corrective learning,auto-context learning,multi-atlas segmentation,joint label fusion
AI 理解论文
溯源树
样例
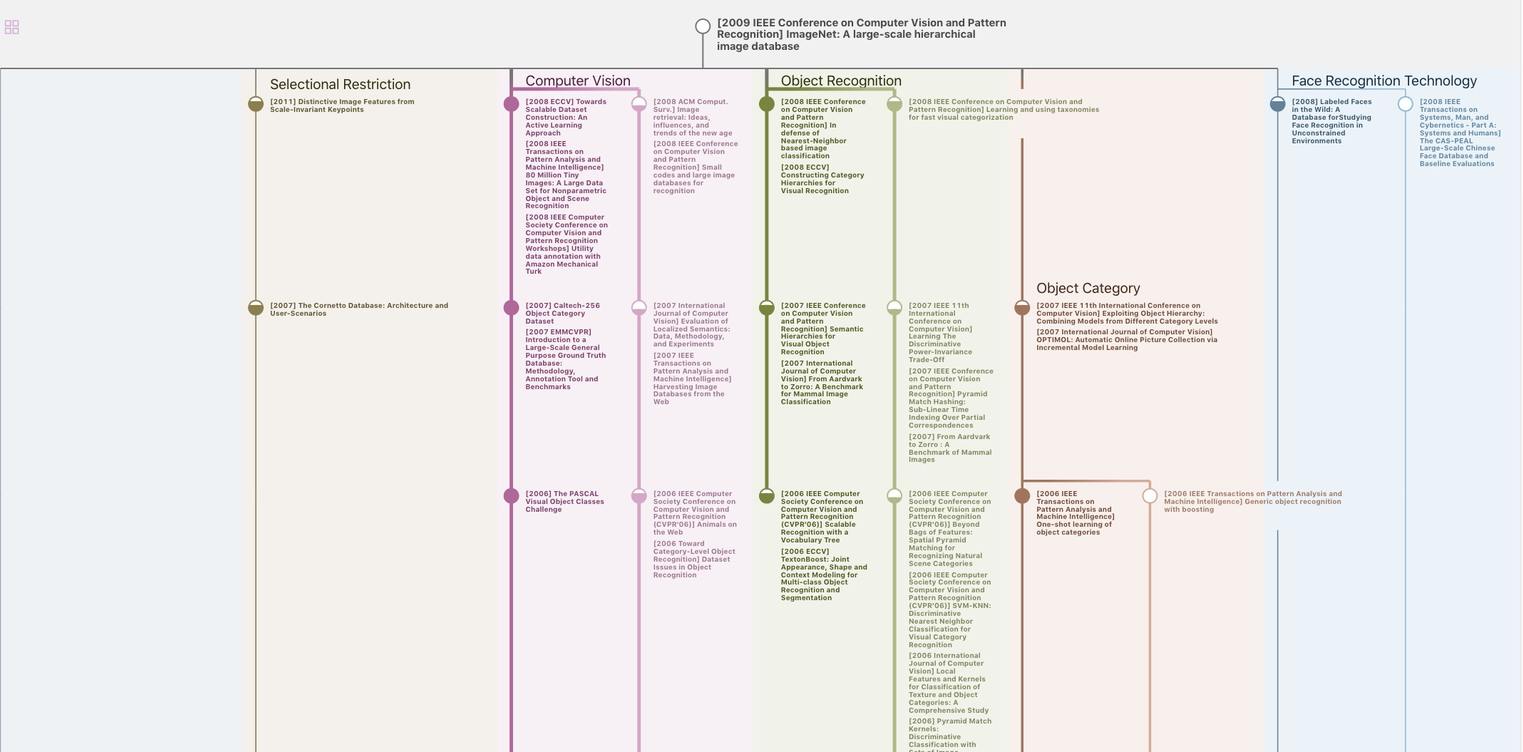
生成溯源树,研究论文发展脉络
Chat Paper
正在生成论文摘要