Bayesian Optimization With Exponential Convergence
NIPS'15 Proceedings of the 28th International Conference on Neural Information Processing Systems(2015)
摘要
This paper presents a Bayesian optimization method with exponential convergence without the need of auxiliary optimization and without the delta-cover sampling. Most Bayesian optimization methods require auxiliary optimization: an additional non-convex global optimization problem, which can be time-consuming and hard to implement in practice. Also, the existing Bayesian optimization method with exponential convergence [1] requires access to the delta-cover sampling, which was considered to be impractical [1, 2]. Our approach eliminates both requirements and achieves an exponential convergence rate.
更多查看译文
AI 理解论文
溯源树
样例
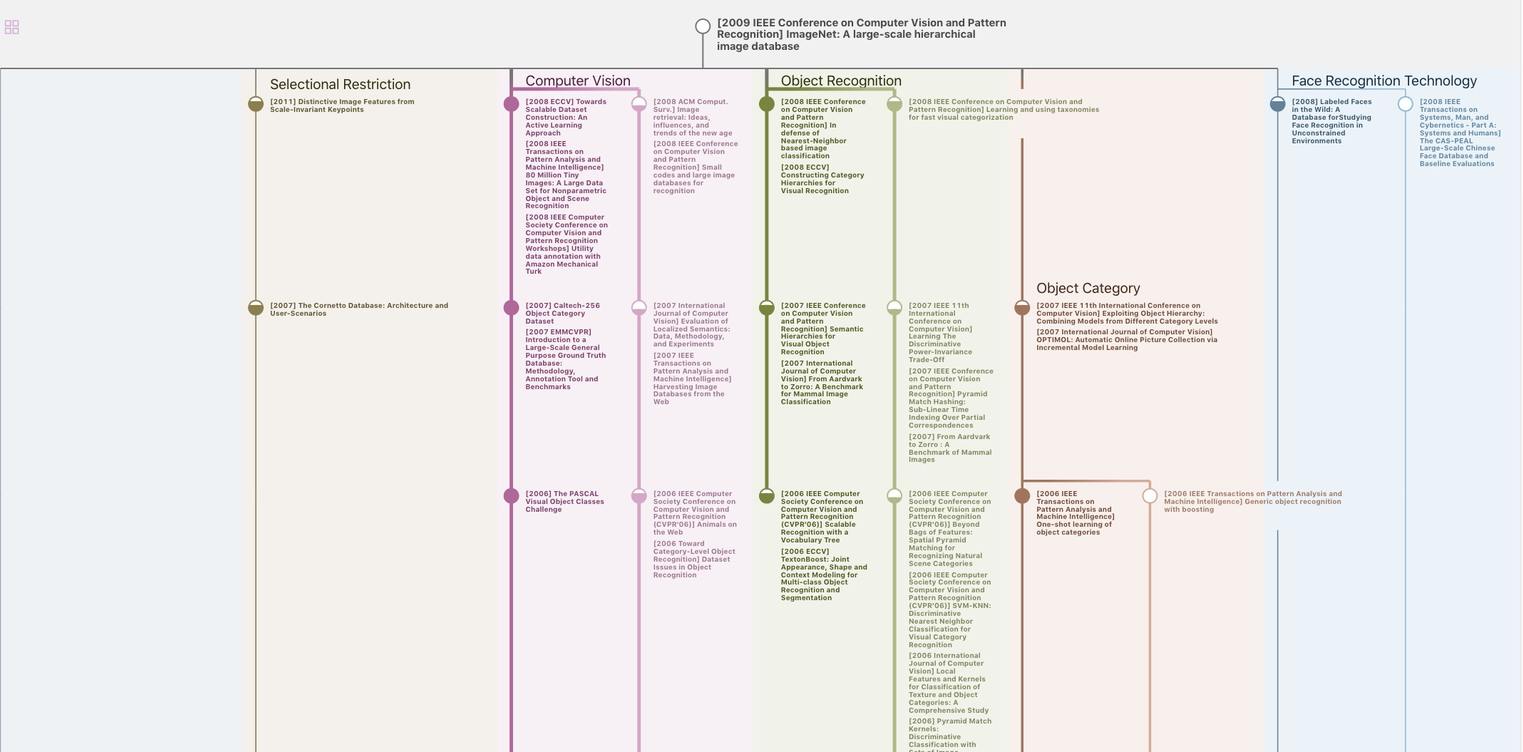
生成溯源树,研究论文发展脉络
Chat Paper
正在生成论文摘要