Spherical Random Features For Polynomial Kernels
NIPS'15: Proceedings of the 28th International Conference on Neural Information Processing Systems - Volume 2(2015)
摘要
Compact explicit feature maps provide a practical framework to scale kernel methods to large-scale learning, but deriving such maps for many types of kernels remains a challenging open problem. Among the commonly used kernels for nonlinear classification are polynomial kernels, for which low approximation error has thus far necessitated explicit feature maps of large dimensionality, especially for higher-order polynomials. Meanwhile, because polynomial kernels are unbounded, they are frequently applied to data that has been normalized to unit l(2) norm. The question we address in this work is: if we know a priori that data is normalized, can we devise a more compact map? We show that a putative affirmative answer to this question based on Random Fourier Features is impossible in this setting, and introduce a new approximation paradigm, Spherical Random Fourier (SRF) features, which circumvents these issues and delivers a compact approximation to polynomial kernels for data on the unit sphere. Compared to prior work, SRF features are less rank-deficient, more compact, and achieve better kernel approximation, especially for higher-order polynomials. The resulting predictions have lower variance and typically yield better classification accuracy.
更多查看译文
AI 理解论文
溯源树
样例
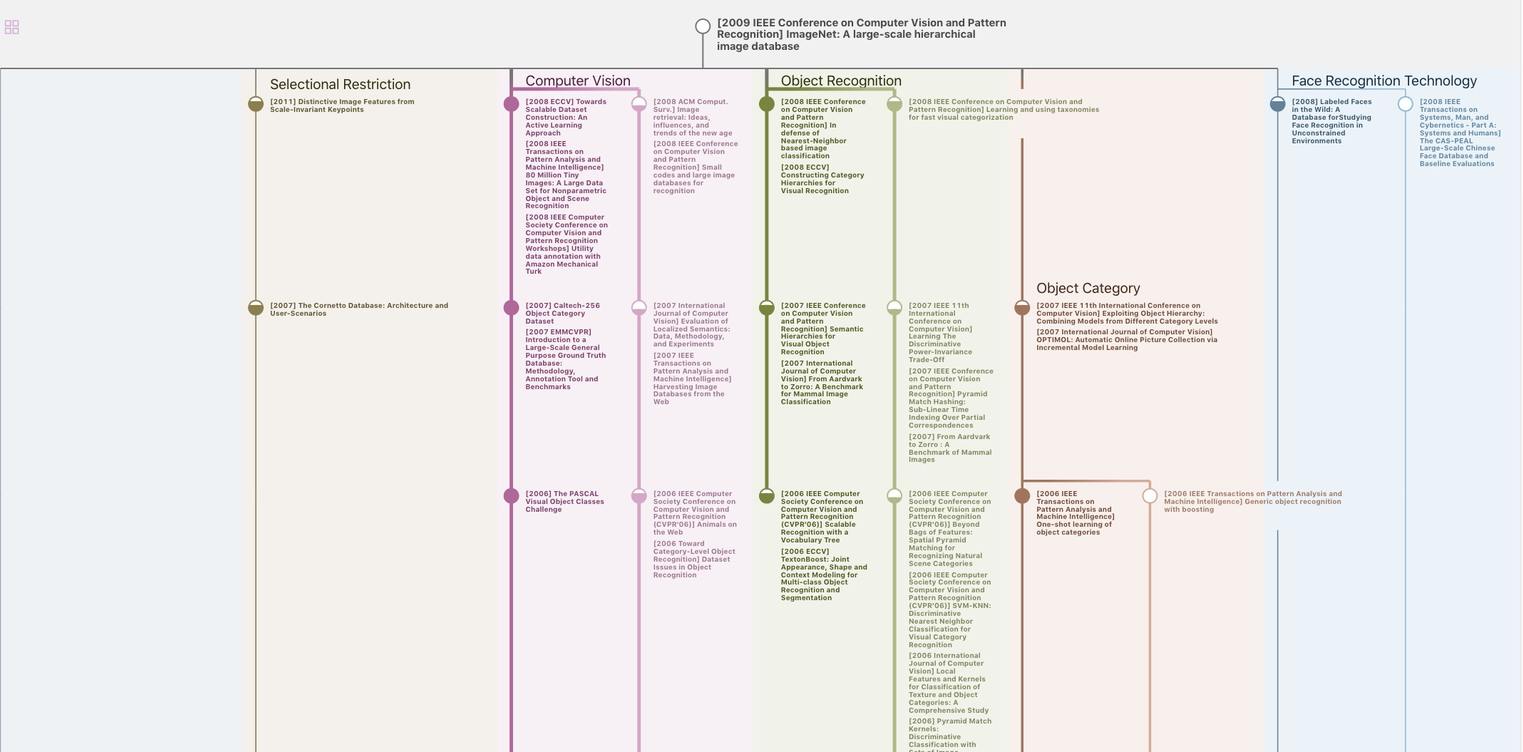
生成溯源树,研究论文发展脉络
Chat Paper
正在生成论文摘要