On Top-k Selection in Multi-Armed Bandits and Hidden Bipartite Graphs
Annual Conference on Neural Information Processing Systems(2015)
摘要
This paper discusses how to efficiently choose from n unknown distributions the k ones whose means are the greatest by a certain metric, up to a small relative error. We study the topic under two standard settings—multi-armed bandits and hidden bipartite graphs—which differ in the nature of the input distributions. In the former setting, each distribution can be sampled (in the i.i.d. manner) an arbitrary number of times, whereas in the latter, each distribution is defined on a population of a finite size m (and hence, is fully revealed after m samples). For both settings, we prove lower bounds on the total number of samples needed, and propose optimal algorithms whose sample complexities match those lower bounds.
更多查看译文
AI 理解论文
溯源树
样例
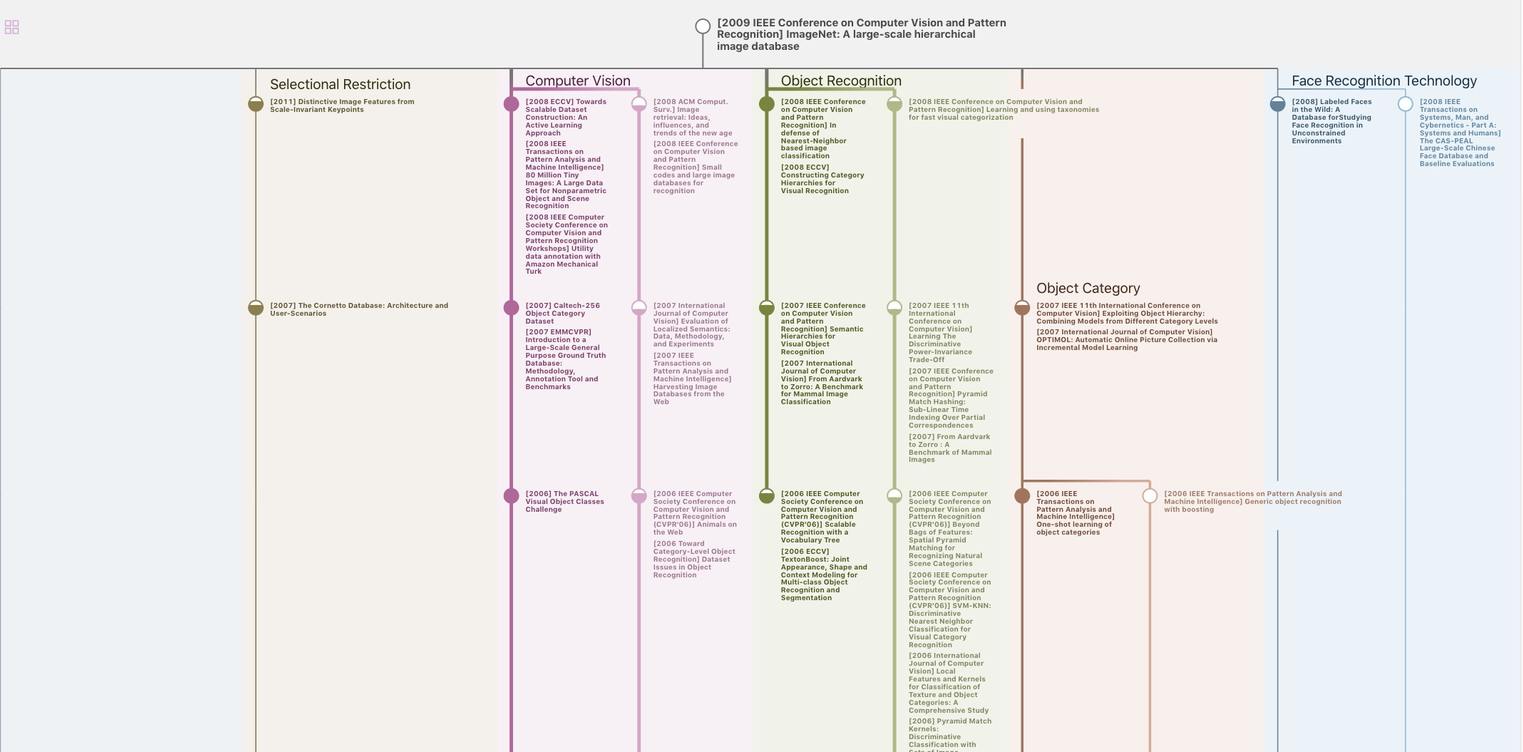
生成溯源树,研究论文发展脉络
Chat Paper
正在生成论文摘要