Classification And Reconstruction Of Compressed Gmm Signals With Side Information
2015 IEEE International Symposium on Information Theory (ISIT)(2015)
摘要
This paper offers a characterization of performance limits for classification and reconstruction of high-dimensional signals from noisy compressive measurements, in the presence of side information. We assume the signal of interest and the side information signal are drawn from a correlated mixture of distributions/components, where each component associated with a specific class label follows a Gaussian mixture model (GMM).We provide sharp sufficient and/or necessary conditions for the phase transition of the misclassification probability and the reconstruction error in the low-noise regime. These conditions, which are reminiscent of the well-known Slepian-Wolf and Wyner-Ziv conditions, are a function of the number of measurements taken from the signal of interest, the number of measurements taken from the side information signal, and the geometry of these signals and their interplay.
更多查看译文
关键词
Classification,reconstruction,Gaussian mixture models,side information
AI 理解论文
溯源树
样例
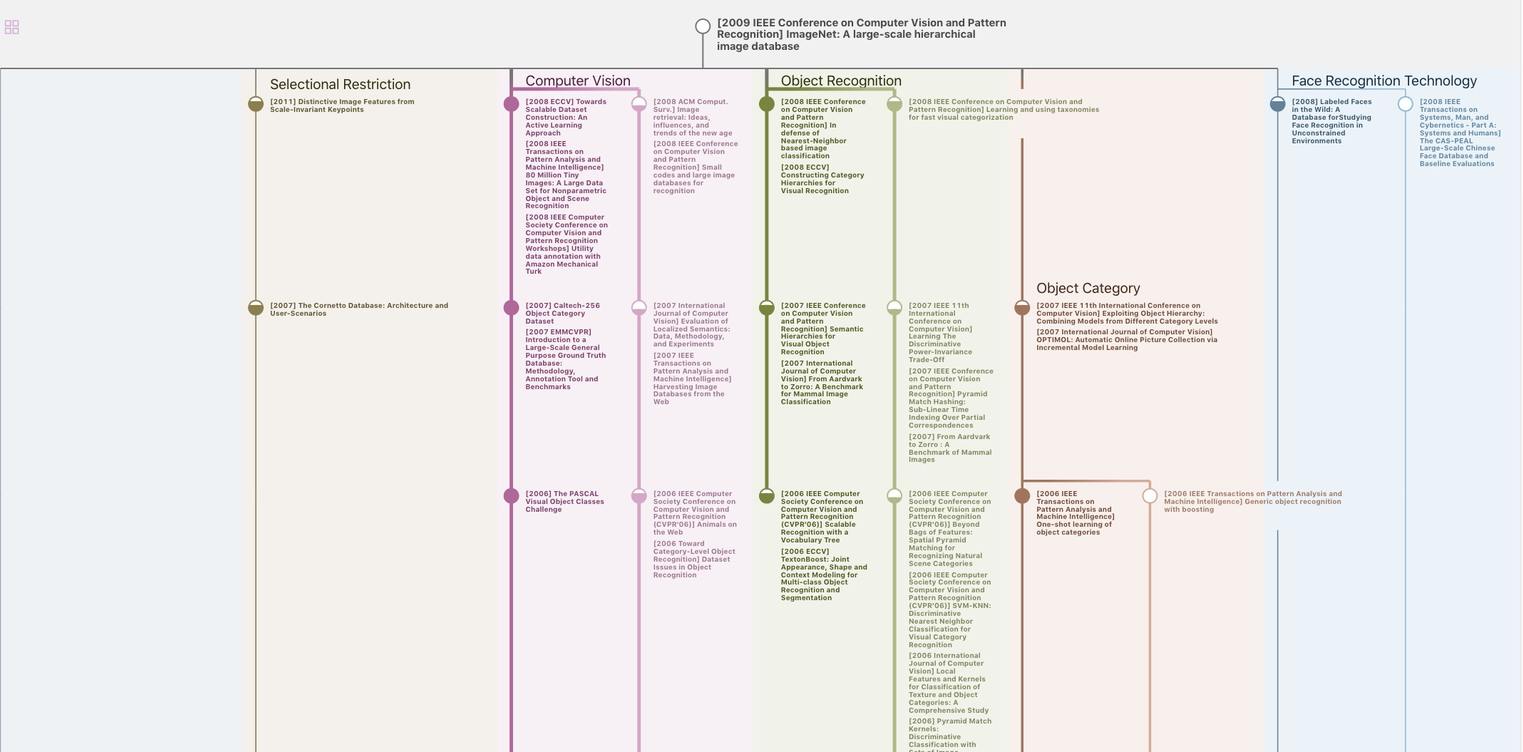
生成溯源树,研究论文发展脉络
Chat Paper
正在生成论文摘要