Re-Active Learning: Active Learning With Relabeling
AAAI'16: Proceedings of the Thirtieth AAAI Conference on Artificial Intelligence(2016)
摘要
Active learning seeks to train the best classifier at the lowest annotation cost by intelligently picking the best examples to label. Traditional algorithms assume there is a single annotator and disregard the possibility of requesting additional independent annotations for a previously labeled example. However, relabeling examples is important, because all annotators make mistakes - especially crowdsourced workers, who have become a common source of training data. This paper seeks to understand the difference in marginal value between decreasing the noise of the training set via relabeling and increasing the size and diversity of the (noisier) training set by labeling new examples. We use the term re-active learning to denote this generalization of active learning. We show how traditional active learning methods perform poorly at re-active learning, present new algorithms designed for this important problem, formally characterize their behavior, and empirically show that our methods effectively make this tradeoff.
更多查看译文
AI 理解论文
溯源树
样例
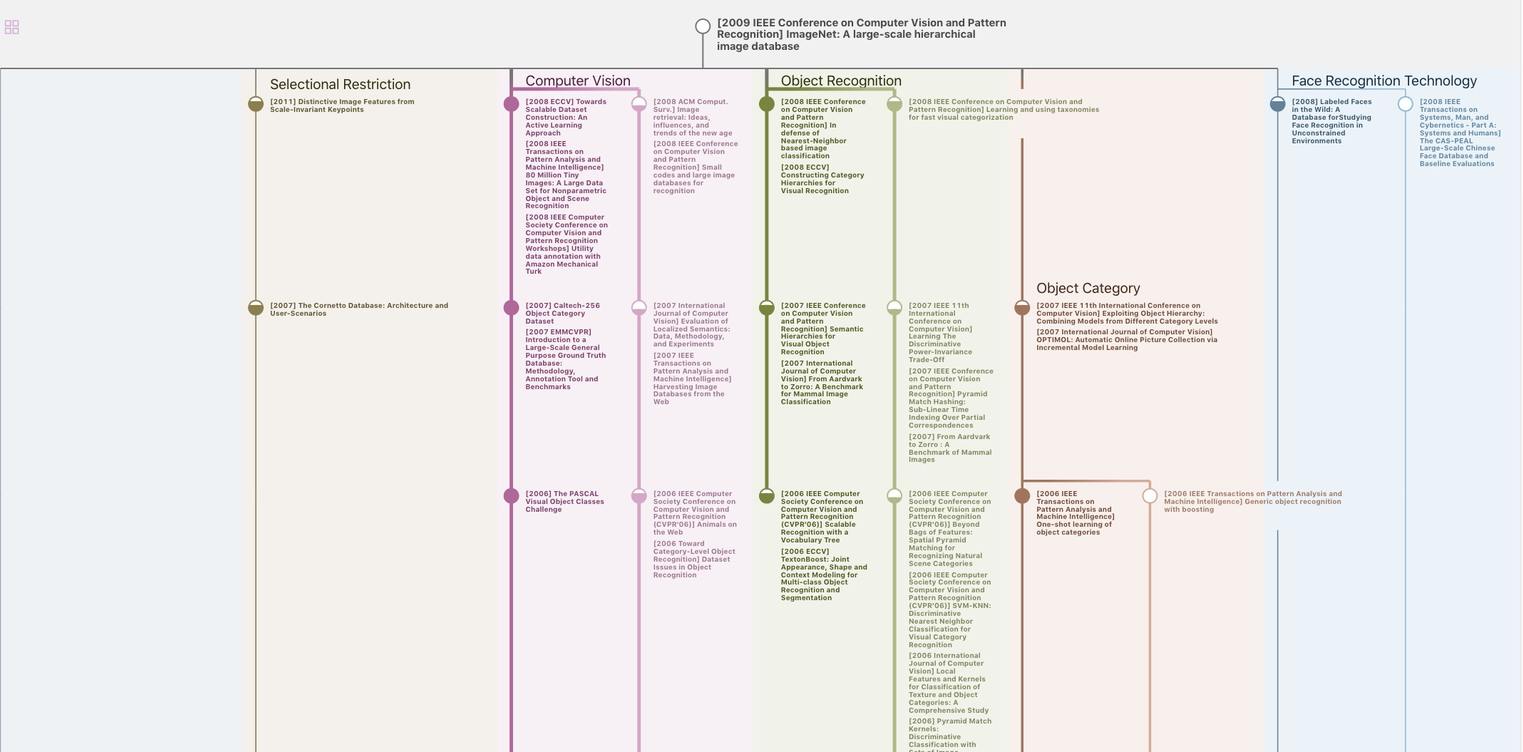
生成溯源树,研究论文发展脉络
Chat Paper
正在生成论文摘要