A Semi-Supervised Learning Approach To Why-Question Answering
AAAI'16: Proceedings of the Thirtieth AAAI Conference on Artificial Intelligence(2016)
摘要
We propose a semi-supervised learning method for improving why-question answering (why-QA). The key of our method is to generate training data (question-answer pairs) from causal relations in texts such as "[Tsunamis are generated](effect) because [the ocean's water mass is displaced by an earthquake](cause)." A naive method for the generation would be to make a question-answer pair by simply converting the effect part of the causal relations into a why-question, like " Why are tsunamis generated?" from the above example, and using the source text of the causal relations as an answer. However, in our preliminary experiments, this naive method actually failed to improve the why-QA performance. The main reason was that the machine-generated questions were often incomprehensible like " Why does (it) happen?", and that the system suffered from overfitting to the results of our automatic causality recognizer. Hence, we developed a novel method that effectively filters out incomprehensible questions and retrieves from texts answers that are likely to be paraphrases of a given causal relation. Through a series of experiments, we showed that our approach significantly improved the precision of the top answer by 8% over the current state-of-the-art system for Japanese why-QA.
更多查看译文
AI 理解论文
溯源树
样例
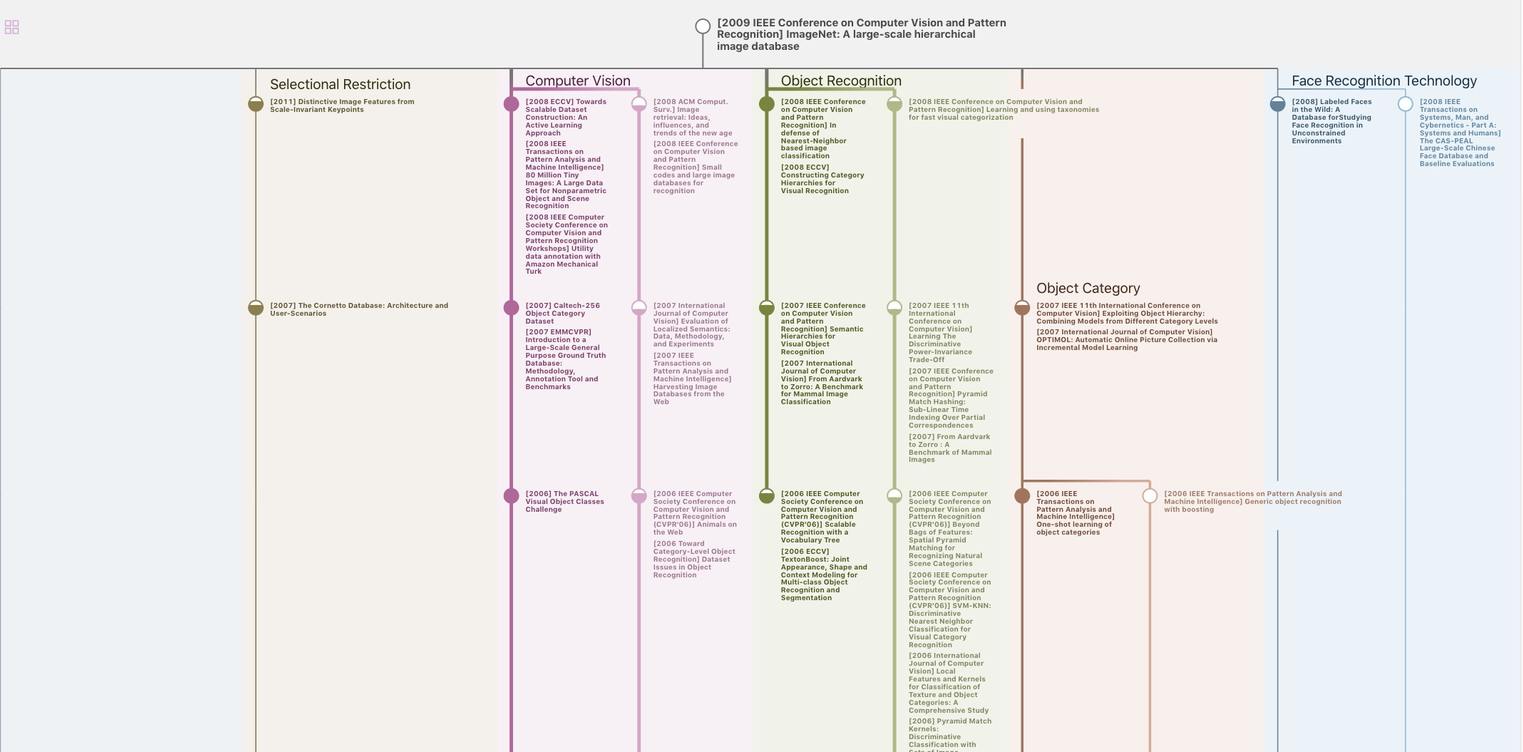
生成溯源树,研究论文发展脉络
Chat Paper
正在生成论文摘要