Parallel Importance Sampling In Conditional Linear Gaussian Networks
Lecture Notes in Computer Science(2015)
摘要
In this paper we analyse the problem of probabilistic inference in CLG networks when evidence comes in streams. In such situations, fast and scalable algorithms, able to provide accurate responses in a short time are required. We consider the instantiation of variational inference and importance sampling, two well known tools for probabilistic inference, to the CLG case. The experimental results over synthetic networks show how a parallel version importance sampling, and more precisely evidence weighting, is a promising scheme, as it is accurate and scales up with respect to available computing resources.
更多查看译文
关键词
Importance sampling,Variational message passing,Conditional linear Gaussian networks,Hybrid Bayesian networks
AI 理解论文
溯源树
样例
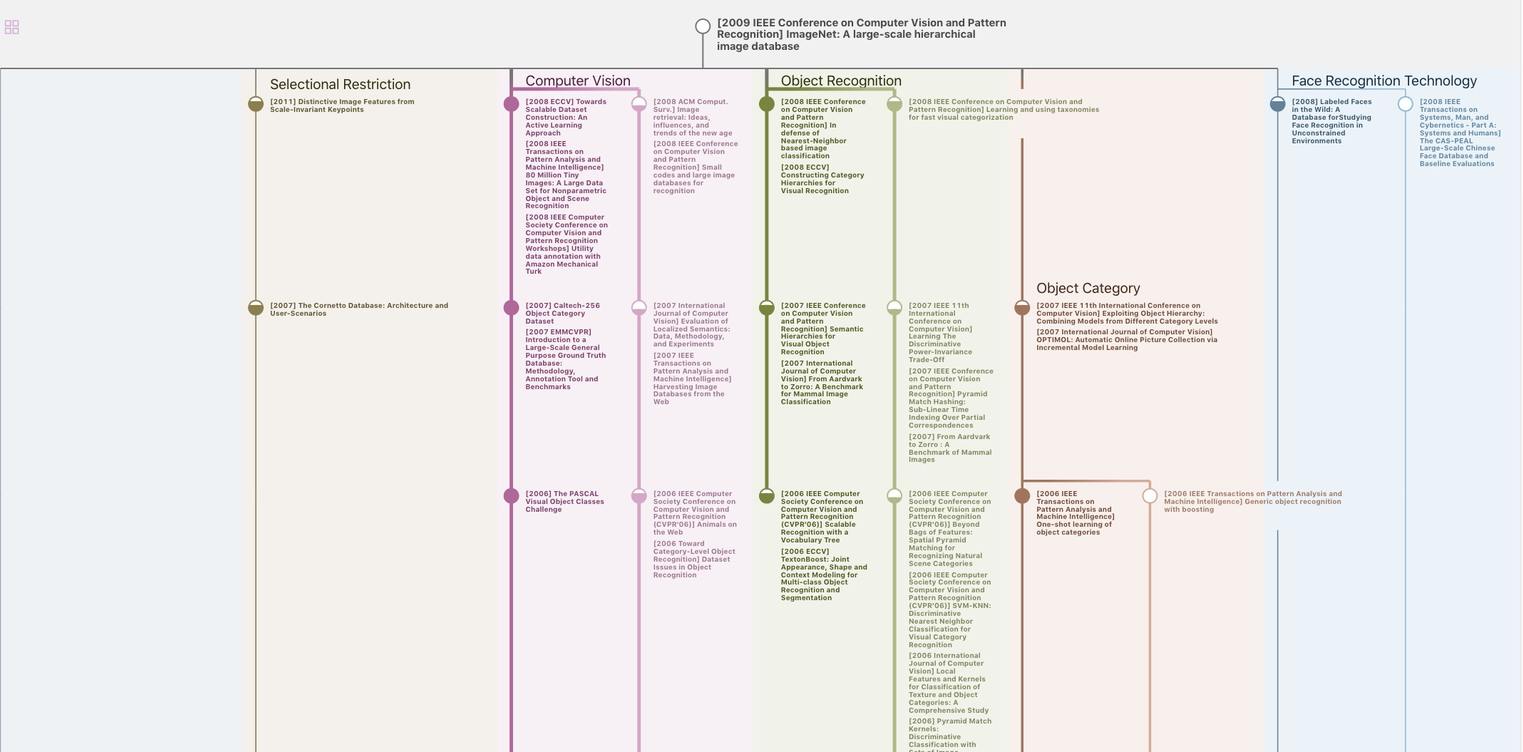
生成溯源树,研究论文发展脉络
Chat Paper
正在生成论文摘要