An Empirical Evaluation of Capture-Recapture Estimators in Software Inspection
ASWEC(2015)
摘要
Context: Capture-recapture approach has been adopted in software inspection for decades for estimating remaining defects and supporting post-inspection decisions. A number of capture-recapture models and estimators have been borrowed from other disciplines (e.g., Biology and social sciences) and applied in software inspections. These models and estimators were created with different assumptions and the violation to these assumptions may lead to invalid application. Most of the reported empirical evaluations of the capture-recapture models and estimators used relatively small data sets (i.e., Small number of inspectors and/or defects), which leads varying conclusions. Objective: The research reported in this paper aims to better understand this approach for software inspection, and the efficacy of the models and estimators in particular, with large data sets. Method: We carried out an empirical study that evaluated several typical capture-recapture estimators (i.e., M0-ML, Mt-ML, Mt-CH, Mh-CH and Mh-JK). The study employed 57 student inspectors in reviewing software requirement specification and formed 1000 virtual teams at each team size in the post statistic testing. Results: The data analysis indicates that: 1) M0-ML and Mt-ML could not generate accurate estimates even with many inspectors. 2) All estimators suffer high level of failure rate with few inspectors. 3) For a small team, estimator Mh-CH performs the best, while with many inspectors, Mt-CH and Mh-JK perform better regarding the accuracy and the failure rate. Conclusion: Our work reveals that no estimator was superior to others under all situations. With an increased team size, estimates generated by estimator Mt-CH and Mh-JK turn to be more accurate than others. While, M0-ML and Mt-ML are able to offer reference value to support post-inspection because of their convergent underestimates.
更多查看译文
关键词
capture-recapture,estimation,inspection
AI 理解论文
溯源树
样例
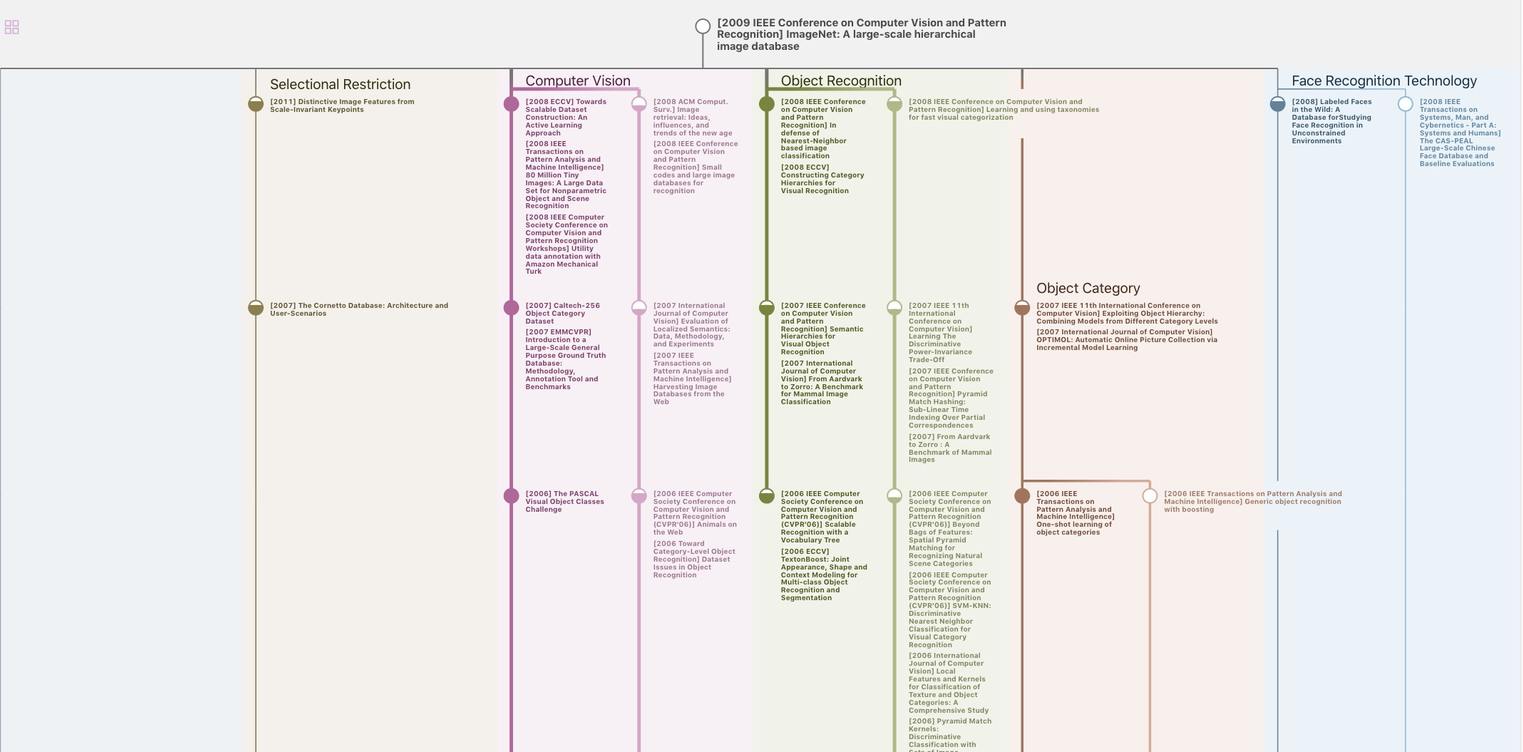
生成溯源树,研究论文发展脉络
Chat Paper
正在生成论文摘要