A privacy protection procedure for large scale individual level data
2015 IEEE International Conference on Intelligence and Security Informatics (ISI)(2015)
摘要
We present a transformation procedure for large scale individual level data that produces output data in which no linear combinations of the resulting attributes can yield the original sensitive attributes from the transformed data. In doing this, our procedure eliminates all linear information regarding a sensitive attribute from the input data. The algorithm combines principal components analysis of the data set with orthogonal projection onto the subspace containing the sensitive attribute(s). The algorithm presented is motivated by applications where there is a need to drastically `sanitize' a data set of all information relating to sensitive attribute(s) before analysis of the data using a data mining algorithm. Sensitive attribute removal (sanitization) is often needed to prevent disparate impact and discrimination on the basis of race, gender, and sexual orientation in high stakes contexts such as determination of access to loans, credit, employment, and insurance. We show through experiments that our proposed algorithm outperforms other privacy preserving techniques by more than 20 percent in lowering the ability to reconstruct sensitive attributes from large scale data.
更多查看译文
关键词
privacy preserving,data mining,orthogonal projection,PCA
AI 理解论文
溯源树
样例
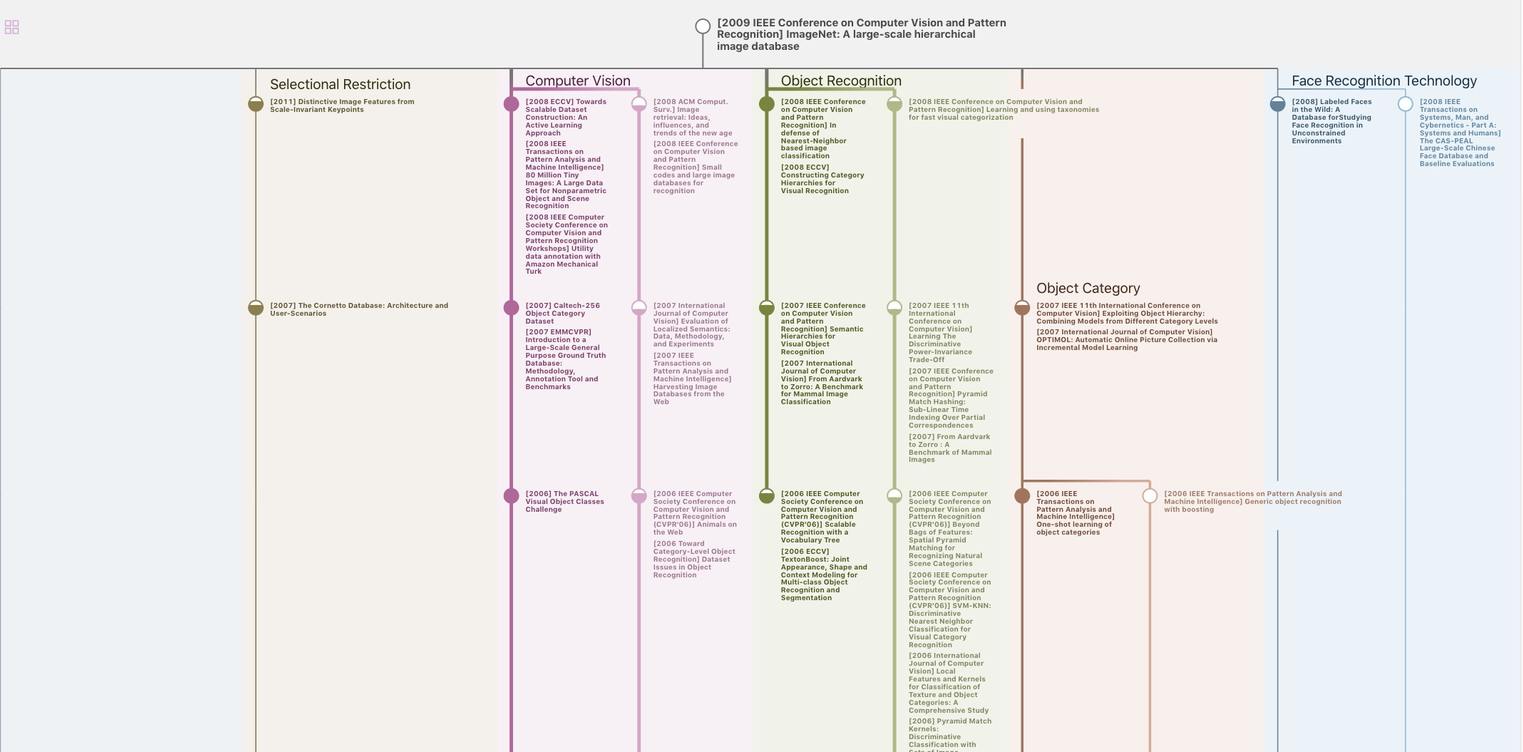
生成溯源树,研究论文发展脉络
Chat Paper
正在生成论文摘要