A dynamic neural field model of self-regulated eye movements during category learning.
CogSci(2015)
摘要
Computational models of category learning and attention have historically focused on capturing trial and experiment level interactions between attention and decision. However, evidence has been accumulating that suggests that the moment-to-moment attentional dynamics of an individual affects both their immediate decision-making processes as well as their overall learning performance. To extend the scope of these formal theories requires a modeling approach that can index fine-grained decision-making at millisecond time scales. Here we implement a model of eye movements during category learning using concepts from Dynamic Neural Field Theory research. Our model uses a combination of timing signals, spatial competition and Hebbian association to simultaneously account for a number of foundational attentional efficiency results from eye tracking and category learning. We report the results of fitting this model to accuracy, fixation probabilities, fixation counts and fixation duration data in 42 subjects from a standard category learning
更多查看译文
AI 理解论文
溯源树
样例
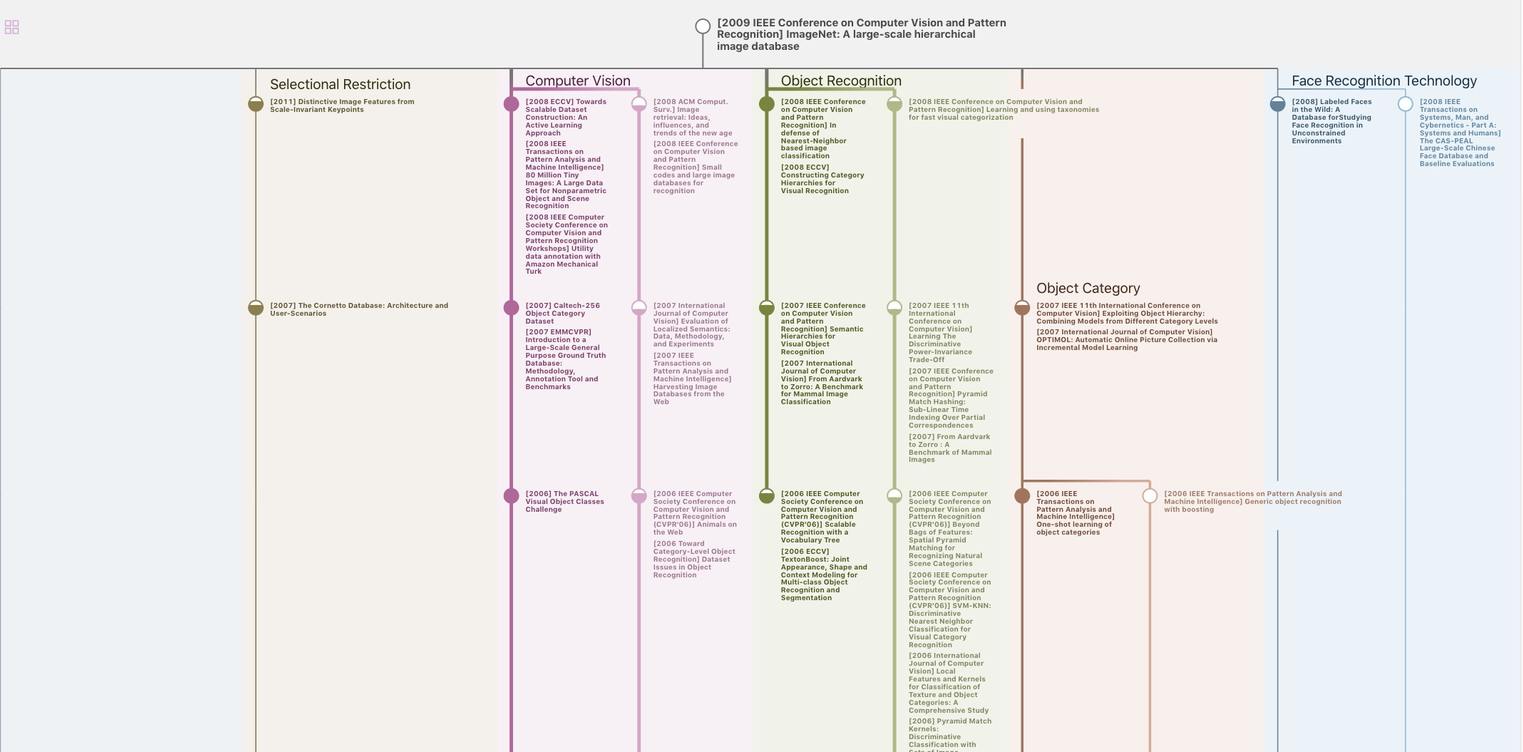
生成溯源树,研究论文发展脉络
Chat Paper
正在生成论文摘要