Hyperspectral Image Analysis Based on Quad Tree Decomposition
INTERNATIONAL JOINT CONFERENCE SOCO'14-CISIS'14-ICEUTE'14(2014)
摘要
Hyperspectral image analysis is among one of the current trends in computer vision and machine learning. Due to the high dimensionality, large number of classes, presence of noise and complex structure, this is not a trivial task. There exists a need for more precise and computationally efficient algorithms for hyperspectral image segmentation and classification. In this paper, we introduce a novel algorithm for segmentation of hyperspectral images and selecting valuable pixels for classifier training procedure. Our approach is based on Quad Tree Decomposition method, which detects homogeneous region in the input image. This allows to precisely detect groups with similar structure and recognize different possible classes on the image. We discuss the computational complexity of our algorithm and show that it can be easily used in real-life applications. Further, this algorithm is extended by active learning approach, which allows to select a single representative pixel for each detected homogeneous region. With this, the classifier is trained on a significantly reduced dataset without sacrificing its quality. We examine the correlation between the number of folds taken by our segmentation algorithm and used classifiers. We show, that the segmentation procedure can be stopped earlier without drop of the accuracy.
更多查看译文
关键词
machine learning,pattern recognition,hyperspectral image,image segmentation
AI 理解论文
溯源树
样例
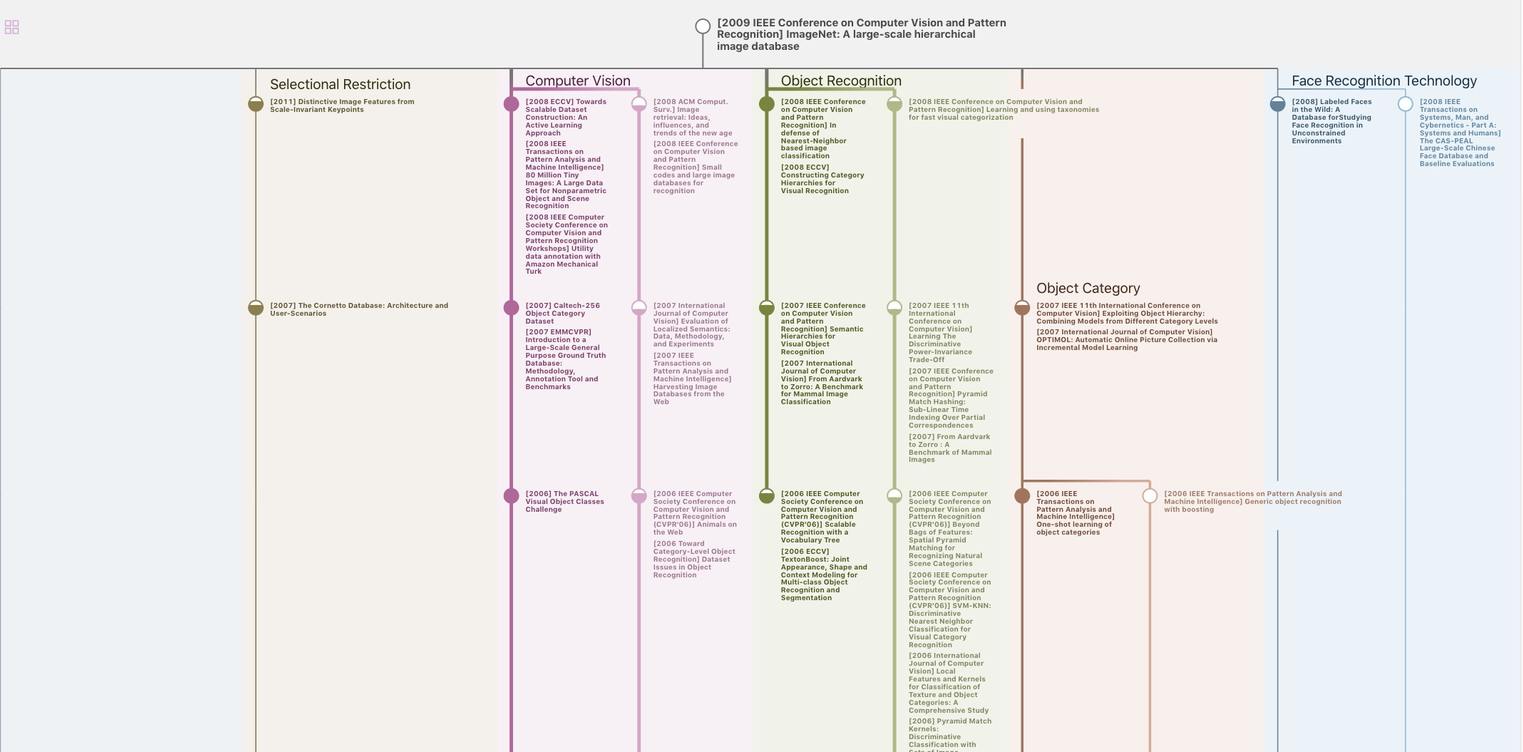
生成溯源树,研究论文发展脉络
Chat Paper
正在生成论文摘要