LYAM++ results for OAEI 2015.
OM(2016)
摘要
The paper presents a novel technique for aligning cross-lingual ontologies that does not rely on machine translation, but uses the large multilingual semantic network BabelNet as a source of background knowledge. In addition, our approach applies a novel orchestration of the components of the matching workflow. We present our results on the evaluation challenge Multifarm. 1 Presentation of the System In spite of the considerable advance that has been made in the field of on-tology matching recently, many questions remain open [1]. The current work addresses the challenge of using background knowledge with a focus on aligning cross-lingual ontologies, i.e., ontologies defined in different natural languages [2]. Indeed, considering multilingual and cross-lingual information is becoming more and more important, in view particularly of the growing number of web content-creating non-English users and the clear demand of cross-language in-teroperability. In the context of the web of data, it is important to propose procedures for linking vocabularies across natural languages, in order to foster the creation of a veritable global information network. The use of different natural languages in the concepts and relations labeling process is becoming an important source of ontology heterogeneity. The methods that have been proposed to deal with it most commonly rely on automatic translation of labels to a single target language [3] or apply machine learning techniques [2]. However, machine translation tolerates low precision levels and machine learning methods require large training corpus that is rarely available in an ontology matching scenario. An inherent problem of translation is that there is often a lack of exact one-to-one correspondence between the terms in different natural languages. 1.1 State, Purpose, General Statement We present LYAM++ (Yet Another Matcher-Light)[4], a fully automatic cross-lingual ontology matching system that does not rely on machine translation. Instead, we make use of the openly available general-purpose multilingual semantic network BabelNet 1 in order to recreate the missing semantic context 1 http://babelnet.org/
更多查看译文
AI 理解论文
溯源树
样例
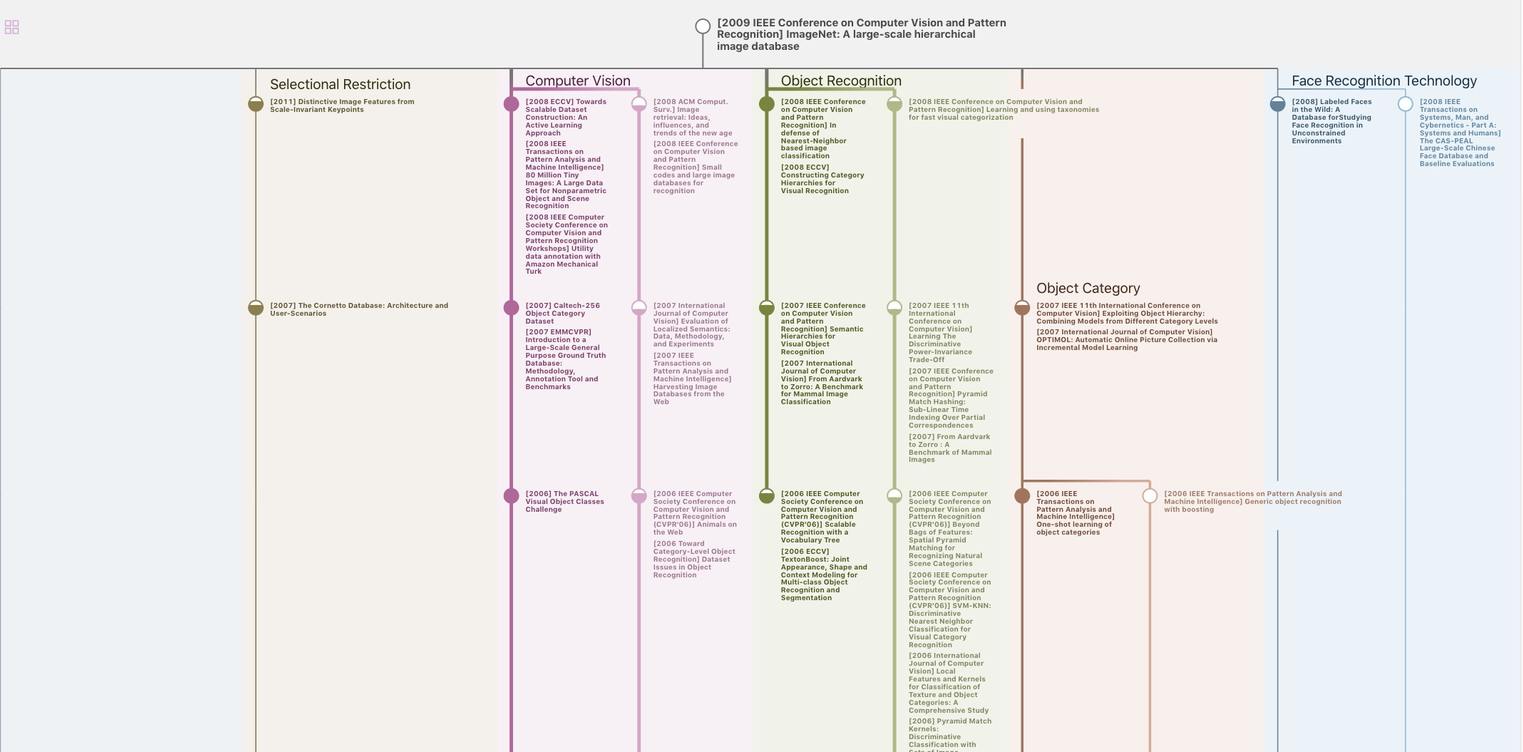
生成溯源树,研究论文发展脉络
Chat Paper
正在生成论文摘要