Semi-Automated Exploration of Data Warehouses.
CIKM'15: 24th ACM International Conference on Information and Knowledge Management Melbourne Australia October, 2015(2015)
摘要
Exploratory data analysis tries to discover novel dependencies and unexpected patterns in large databases. Traditionally, this process is manual and hypothesis-driven. However, analysts can come short of patience and imagination. In this paper, we introduce Claude, a hypothesis generator for data warehouses. Claude follows a 2-step approach: (1) It detects interesting views, by exploiting non-linear statistical dependencies between the dimensions and the measure. (2) To explain its findings, it detects local patterns in these views and describes them with SQL queries. Technically, we derive a model of interestingness from fundamental information theory. To exploit this model, we present aggressive approximations and heuristics, allowing Claude to be fast and more accurate than state-of-art view selection algorithms.
更多查看译文
AI 理解论文
溯源树
样例
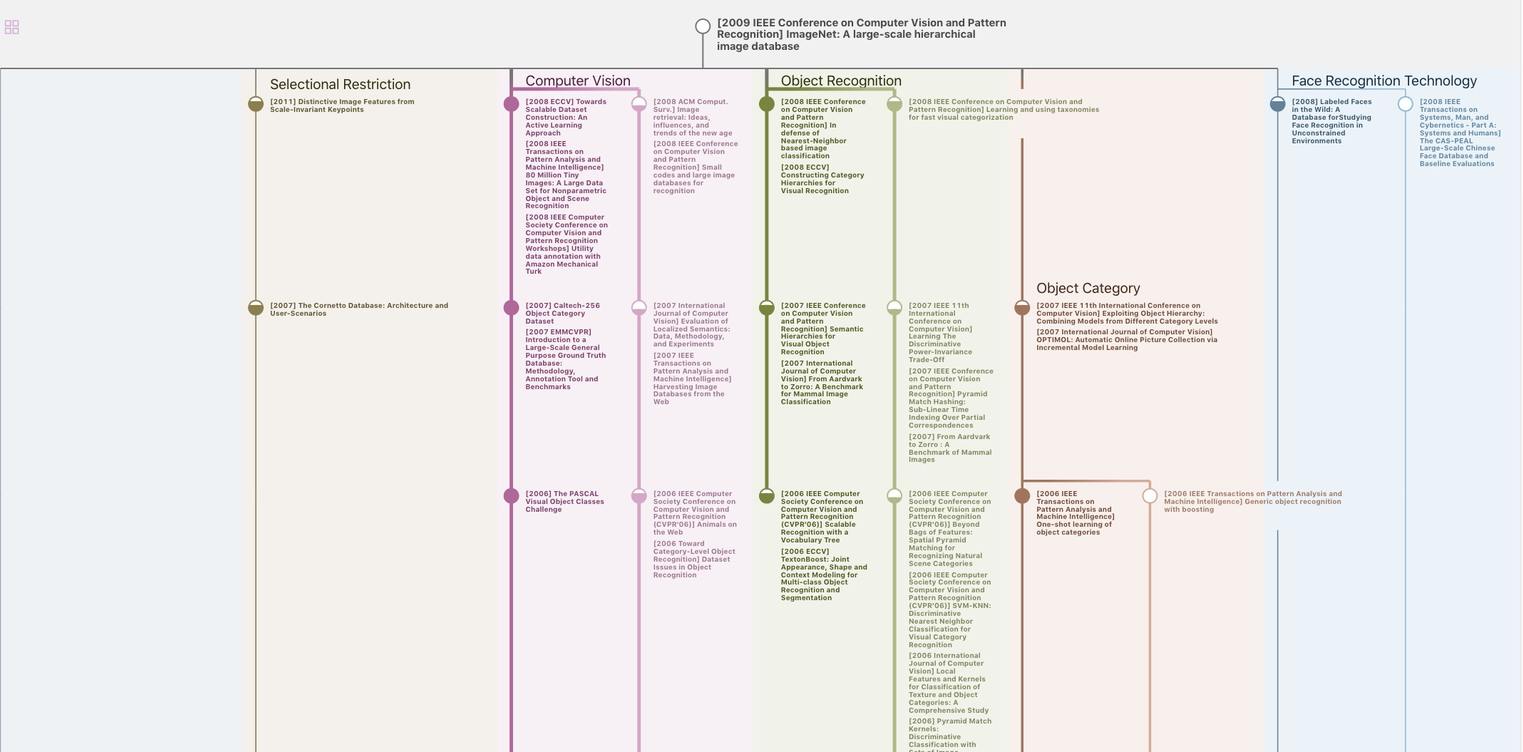
生成溯源树,研究论文发展脉络
Chat Paper
正在生成论文摘要