Topic Modeling in Semantic Space with Keywords.
CIKM'15: 24th ACM International Conference on Information and Knowledge Management Melbourne Australia October, 2015(2015)
摘要
A common and convenient approach for user to describe his information need is to provide a set of keywords. Therefore, the technique to understand the need becomes crucial. In this paper, for the information need about a topic or category, we propose a novel method called TDCS(Topic Distilling with Compressive Sensing) for explicit and accurate modeling the topic implied by several keywords. The task is transformed as a topic reconstruction problem in the semantic space with a reasonable intuition that the topic is sparse in the semantic space. The latent semantic space could be mined from documents via unsupervised methods, e.g. LSI. Compressive sensing is leveraged to obtain a sparse representation from only a few keywords. In order to make the distilled topic more robust, an iterative learning approach is adopted. The experiment results show the effectiveness of our method. Moreover, with only a few semantic concepts remained for the topic, our method is efficient for subsequent text mining tasks.
更多查看译文
AI 理解论文
溯源树
样例
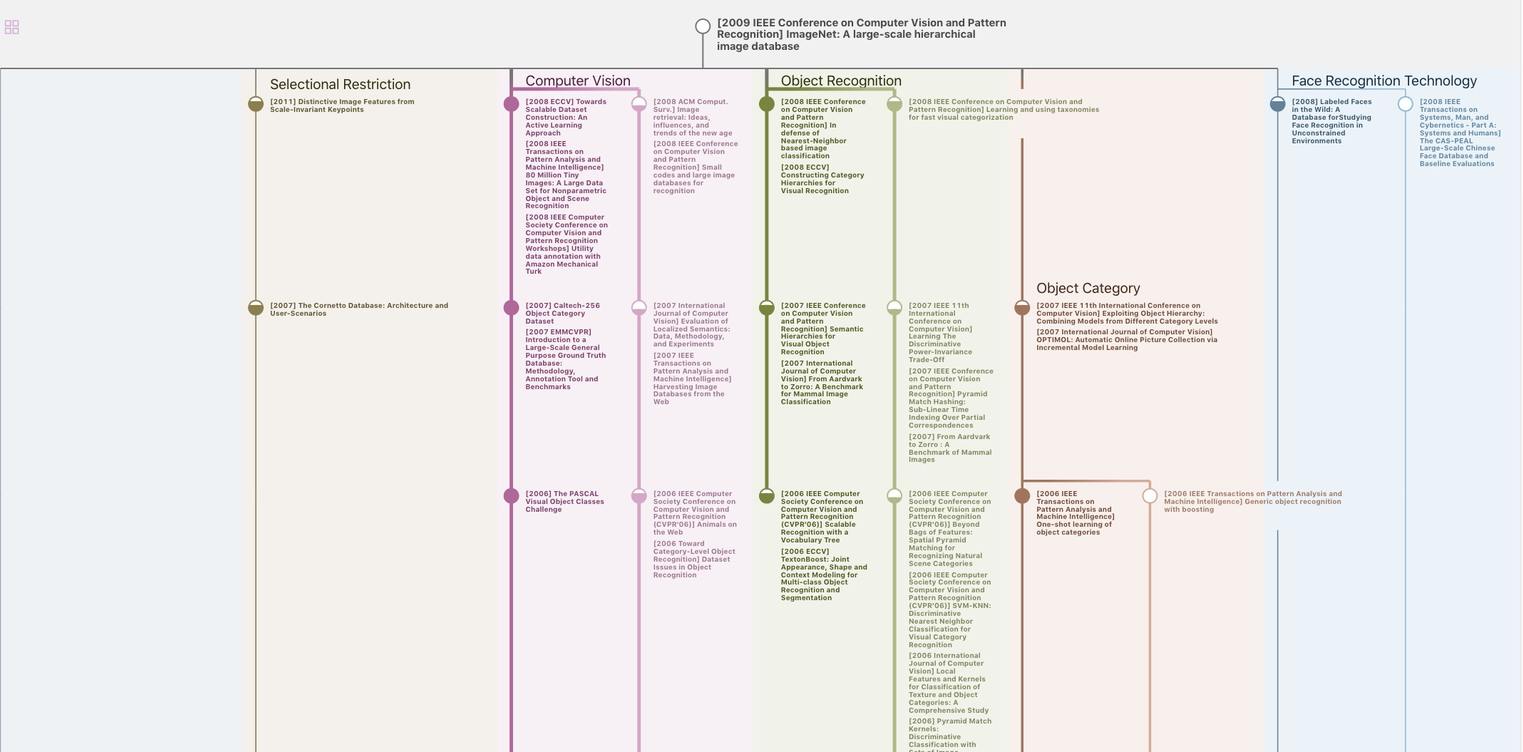
生成溯源树,研究论文发展脉络
Chat Paper
正在生成论文摘要