gSparsify: Graph Motif Based Sparsification for Graph Clustering
ACM International Conference on Information and Knowledge Management(2015)
摘要
Graph clustering is a fundamental problem that partitions vertices of a graph into clusters with an objective to optimize the intuitive notions of intra-cluster density and intercluster sparsity. In many real-world applications, however, the sheer sizes and inherent complexity of graphs may render existing graph clustering methods inefficient or incapable of yielding quality graph clusters. In this paper, we propose gSparsify, a graph sparsification method, to preferentially retain a small subset of edges from a graph which are more likely to be within clusters, while eliminating others with less or no structure correlation to clusters. The resultant simplified graph is succinct in size with core cluster structures well preserved, thus enabling faster graph clustering without a compromise to clustering quality. We consider a quantitative approach to modeling the evidence that edges within densely knitted clusters are frequently involved in small-size graph motifs, which are adopted as prime features to differentiate edges with varied cluster significance. Path-based indexes and path-join algorithms are further designed to compute graph-motif based cluster significance of edges for graph sparsification. We perform experimental studies in real-world graphs, and results demonstrate that gSparsify can bring significant speedup to existing graph clustering methods with an improvement to graph clustering quality.
更多查看译文
AI 理解论文
溯源树
样例
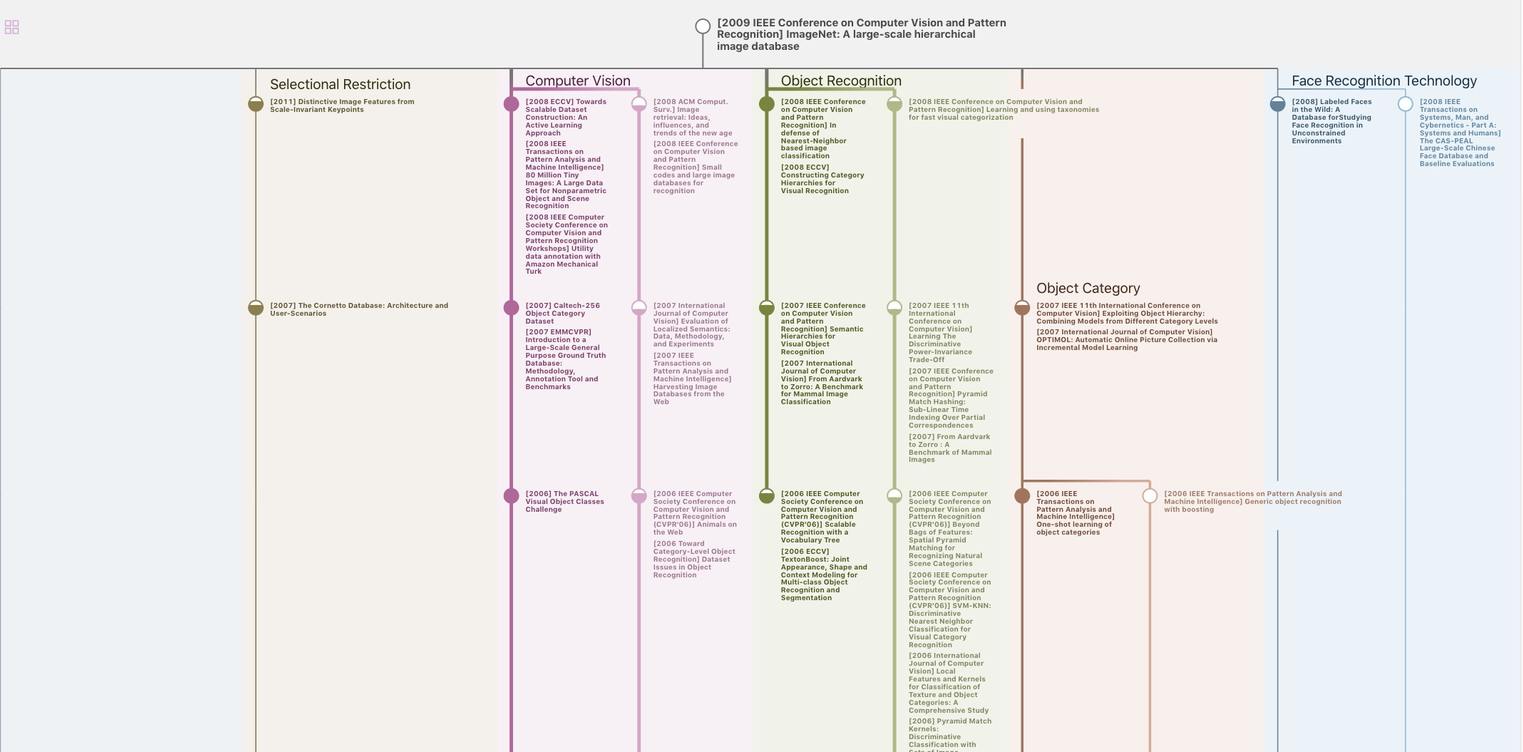
生成溯源树,研究论文发展脉络
Chat Paper
正在生成论文摘要