Combining One-vs-One Decomposition and Ensemble Learning for Multi-class Imbalanced Data.
PROCEEDINGS OF THE 9TH INTERNATIONAL CONFERENCE ON COMPUTER RECOGNITION SYSTEMS, CORES 2015(2016)
摘要
Learning from imbalanced data poses significant challenges for machine learning algorithms, as they need to deal with uneven distribution of examples in the training set. As standard classifiers will be biased toward the majority class there exist a need for specific methods than can overcome this single-class dominance. Most of works concentrated on binary problems, where majority and minority class can be distinguished. But a more challenging problem arises when imbalance is present within multi-class datasets, as relations between classes tend to complicate. One class can be a minority class for some, while a majority for others. In this paper, we propose an efficient method for handling such scenarios that combines the problem decomposition with ensemble learning. According to divide-and-conquer rule, we decompose our multi-class data into a number of binary subproblems using one-versus-one approach. To each simplified task we delegate a ensemble of classifiers dedicated to binary imbalanced problems. Then using a dedicated classifier fusion approach, we reconstruct the original multi-class problem. Experimental analysis backed-up with statistical testing clearly proves that such an approach is superior to state-of-the art ad hoc and decomposition methods used in the literature.
更多查看译文
关键词
Ensemble classifiers,Imbalanced data,Multi-class classification,Pairwise learning,Binary decomposition
AI 理解论文
溯源树
样例
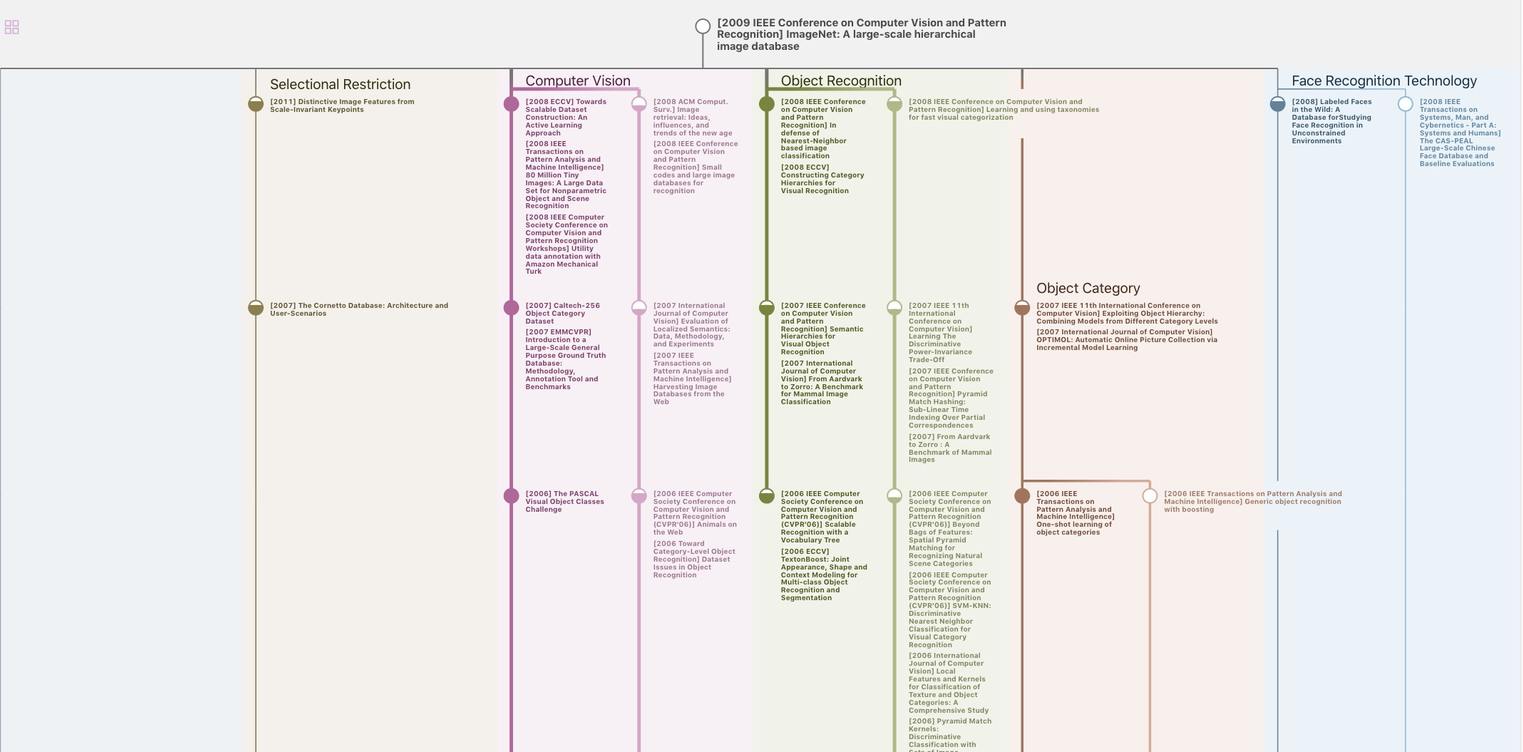
生成溯源树,研究论文发展脉络
Chat Paper
正在生成论文摘要