An Iterative Projective Clustering Method
Procedia Computer Science(2015)
摘要
In this article we offer an algorithm recurrently divides a dataset by search of partitions via one dimensional subspace discovered by means of optimizing of a projected pursuit function. Aiming to assess the model order a resampling technique is employed. For each number of clusters, bounded by a predefined limit, samples from the projected data are drawn and clustered through the EM algorithm. Further, the basis cumulative histogram of the projected data is approximated by means of the GMM histograms constructed using the samples’ partitions. The saturation order of this approximation process, at what time the components’ amount increases, is recognized as the “true” components’ number. Afterward the whole data is clustered and the densest cluster is omitted. The process is repeated while waiting for the true number of clusters equals one. Numerical experiments demonstrate the high ability of the proposed method.
更多查看译文
关键词
Projective Clustering,Projected Pursuit,Image Segmentation
AI 理解论文
溯源树
样例
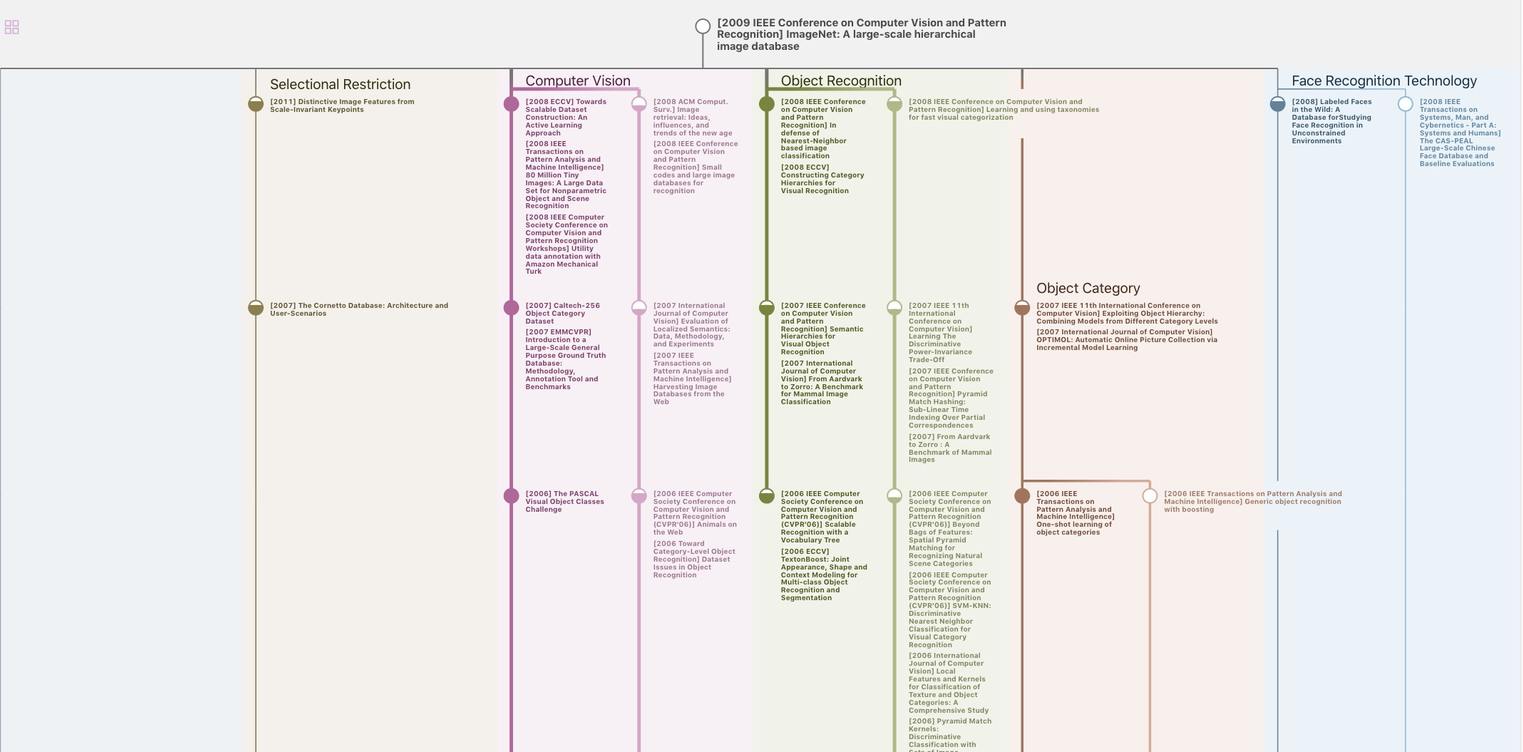
生成溯源树,研究论文发展脉络
Chat Paper
正在生成论文摘要