Hamiltonian Simulation with Nearly Optimal Dependence on all Parameters
IEEE Symposium on Foundations of Computer Science(2015)
摘要
We present an algorithm for sparse Hamiltonian simulation whose complexity is optimal (up to log factors) as a function of all parameters of interest. Previous algorithms had optimal or near-optimal scaling in some parameters at the cost of poor scaling in others. Hamiltonian simulation via a quantum walk has optimal dependence on the sparsity at the expense of poor scaling in the allowed error. In contrast, an approach based on fractional-query simulation provides optimal scaling in the error at the expense of poor scaling in the sparsity. Here we combine the two approaches, achieving the best features of both. By implementing a linear combination of quantum walk steps with coefficients given by Bessel functions, our algorithm's complexity (as measured by the number of queries and 2-qubit gates) is logarithmic in the inverse error, and nearly linear in the product tau of the evolution time, the sparsity, and the magnitude of the largest entry of the Hamiltonian. Our dependence on the error is optimal, and we prove a new lower bound showing that no algorithm can have sub linear dependence on tau.
更多查看译文
关键词
Hamiltonian simulation,quantum algorithms
AI 理解论文
溯源树
样例
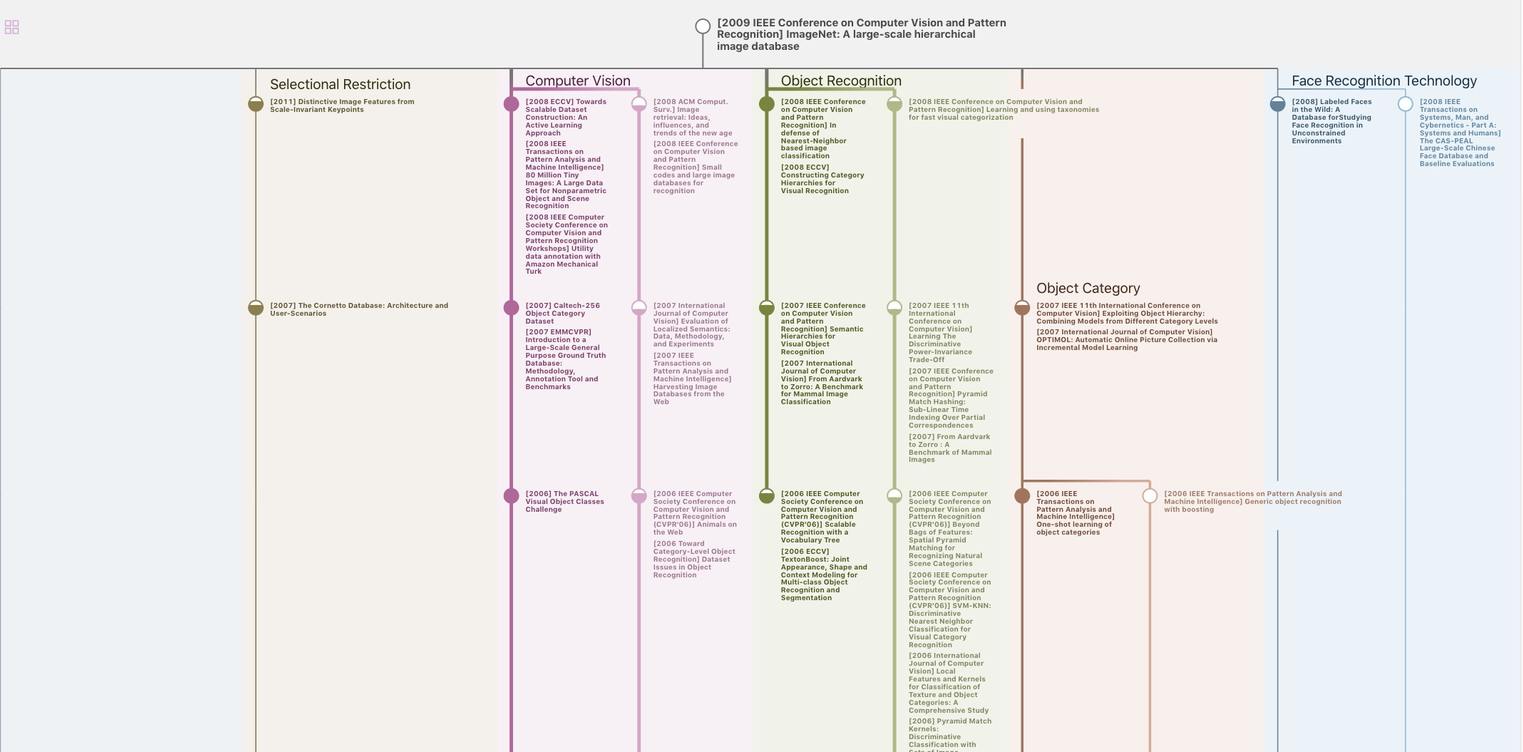
生成溯源树,研究论文发展脉络
Chat Paper
正在生成论文摘要