SGP: Sampling Big Social Network Based on Graph Partition
Proceedings of International Conference on Service Science, ICSS(2016)
摘要
Deriving a representative sample from a big social network is essential for many Internet services that rely on accurate analysis of big social data. A good sampling method for social network should be able to generate small sample networks with similar structures as original big network. In this paper, we propose SGP, a new big social network sampling algorithm based on graph partition. In SGP, original network is firstly partitioned into several sub-networks that will be sampled evenly. This procedure enables SGP to effectively maintain the topological similarity and community structure similarity between the sampled network and its original network. We have evaluated SGP on several well-known data sets. The experimental results show that SGP outperforms six state-of-the-art methods. © 2015 IEEE.
更多查看译文
关键词
sampling algorithms, social networks, graph partition, community structure, topology structure
AI 理解论文
溯源树
样例
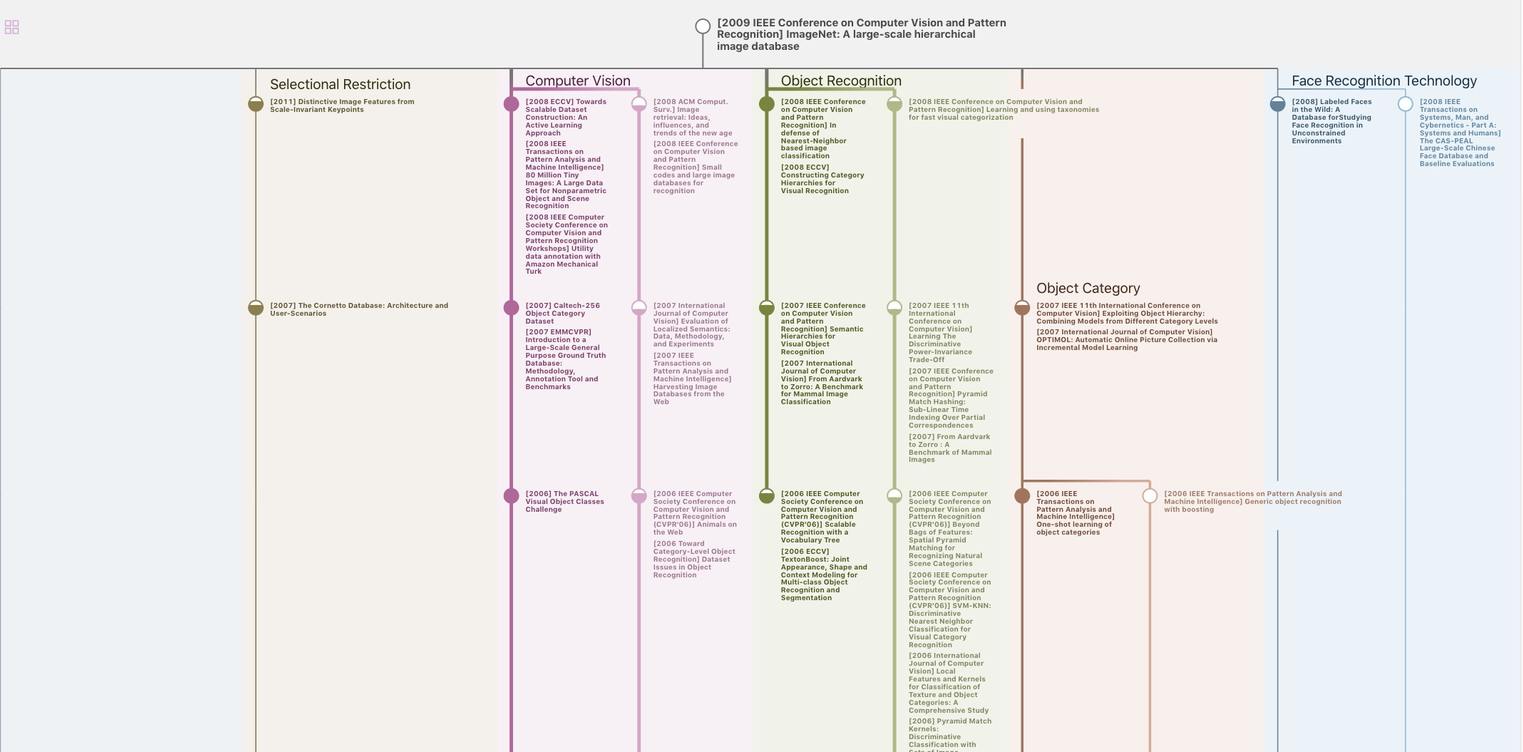
生成溯源树,研究论文发展脉络
Chat Paper
正在生成论文摘要