Reliable seizure prediction from EEG data
2015 International Joint Conference on Neural Networks (IJCNN)(2015)
摘要
There is a growing interest in data-analytic modeling for prediction and/or detection of epileptic seizures from EEG recording of brain activity [1-10]. Even though there is clear evidence that many patients have changes in EEG signal prior to seizures, development of robust seizure prediction methods remains elusive [1]. We argue that the main issue for development of effective EEG-based predictive models is an apparent disconnect between clinical considerations and dataanalytic modeling assumptions. We present an SVM-based system for seizure prediction, where design choices and performance metrics are clearly related to clinical objectives and constraints. This system achieves very accurate prediction of preictal and interictal EEG segments in dogs with naturally occurring epilepsy. However, our empirical results suggest that good prediction performance may be possible only if the training data set has sufficiently many preictal segments, i.e. at least 6-7 seizure episodes.
更多查看译文
关键词
epilepsy,iEEG,patient-specific modeling,predictive data analytics,seizure prediction,SVM classification,unbalanced data
AI 理解论文
溯源树
样例
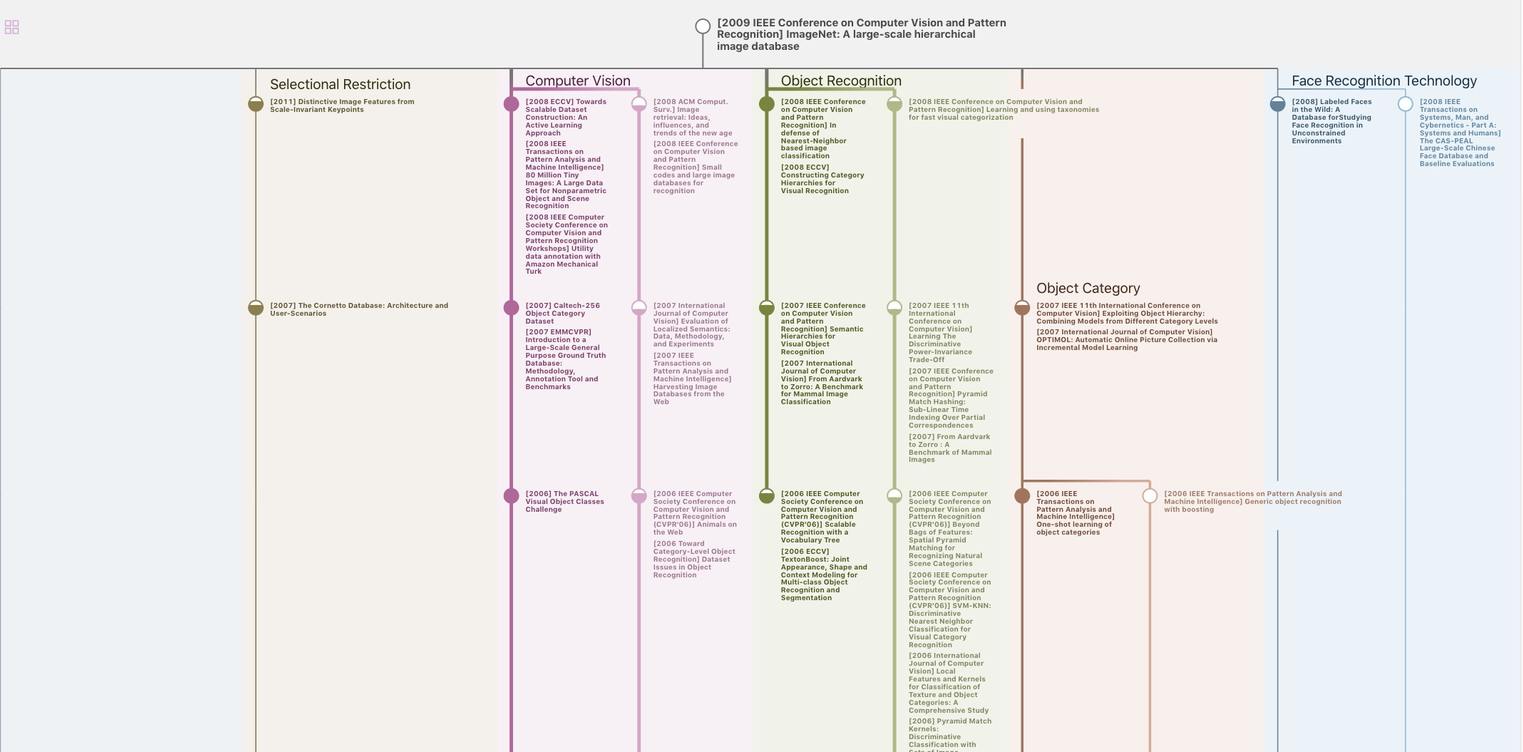
生成溯源树,研究论文发展脉络
Chat Paper
正在生成论文摘要