Non-Linear Prediction With Lstm Recurrent Neural Networks For Acoustic Novelty Detection
2015 International Joint Conference on Neural Networks (IJCNN)(2015)
摘要
Acoustic novelty detection aims at identifying ab-normal/novel acoustic signals which differ from the reference/normal data that the system was trained with. In this paper we present a novel approach based on non-linear predictive denoising autoencoders. In our approach, auditory spectral features of the next short-term frame are predicted from the previous frames by means of Long-Short Term Memory (LSTM) recurrent denoising autoencoders. We show that this yields an effective generative model for audio. The reconstruction error between the input and the output of the autoencoder is used as activation signal to detect novel events. The autoencoder is trained on a public database which contains recordings of typical in-home situations such as talking, watching television, playing and eating. The evaluation was performed on more than 260 different abnormal events. We compare results with state-of-the-art methods and we conclude that our novel approach significantly outperforms existing methods by achieving up to 94.4 % F-Measure.
更多查看译文
关键词
nonlinear prediction,LSTM recurrent neural networks,acoustic novelty detection,abnormal/novel acoustic signals,reference/normal data,nonlinear predictive denoising,long-short term memory,recurrent denoising autoencoders,public database
AI 理解论文
溯源树
样例
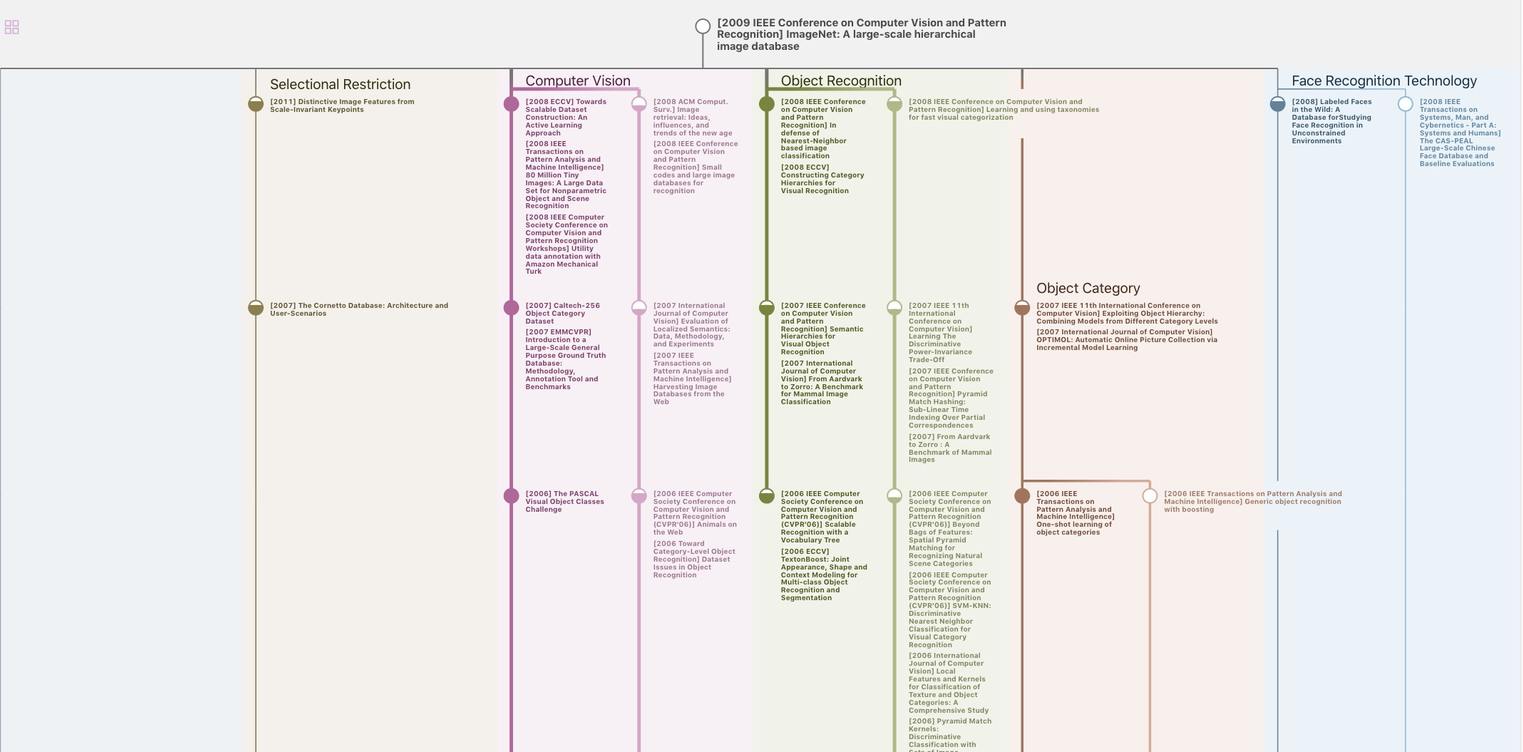
生成溯源树,研究论文发展脉络
Chat Paper
正在生成论文摘要