Collaborative Multi-Output Gaussian Processes
UAI'14: Proceedings of the Thirtieth Conference on Uncertainty in Artificial Intelligence(2014)
摘要
We introduce the collaborative multi-output Gaussian process (GP) model for learning dependent tasks with very large datasets. The model fosters task correlations by mixing sparse processes and sharing multiple sets of inducing points. This facilitates the application of variational inference and the derivation of an evidence lower bound that decomposes across inputs and outputs. We learn all the parameters of the model in a single stochastic optimization framework that scales to a large number of observations per output and a large number of outputs. We demonstrate our approach on a toy problem, two medium-sized datasets and a large dataset. The model achieves superior performance compared to single output learning and previous multi-output GP models, confirming the benefits of correlating sparsity structure of the outputs via the inducing points.
更多查看译文
AI 理解论文
溯源树
样例
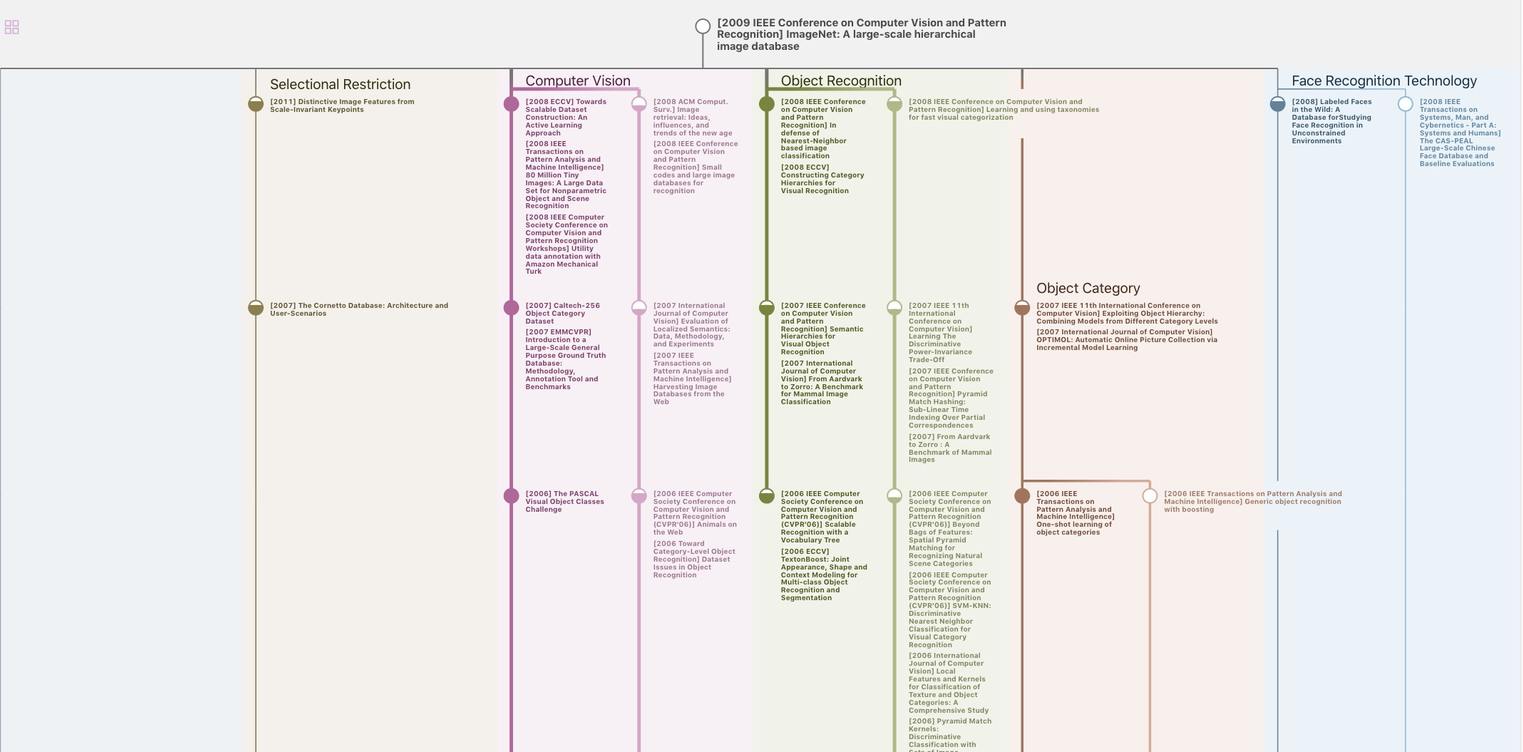
生成溯源树,研究论文发展脉络
Chat Paper
正在生成论文摘要