The Long-Run Behavior Of Continuous Time Bayesian Networks
UAI'15: Proceedings of the Thirty-First Conference on Uncertainty in Artificial Intelligence(2015)
摘要
The continuous time Bayesian network (CTBN) is a temporal model consisting of interdependent continuous time Markov chains (Markov processes). One common analysis performed on Markov processes is determining their long-run behavior, such as their stationary distributions. While the CTBN can be transformed into a single Markov process of all nodes' state combinations, the size is exponential in the number of nodes, making traditional long-run analysis intractable. To address this, we show how to perform "long-run" node marginalization that removes a node's conditional dependence while preserving its long-run behavior. This allows long-run analysis of CTBNs to be performed in a top-down process without dealing with the entire network all at once.
更多查看译文
AI 理解论文
溯源树
样例
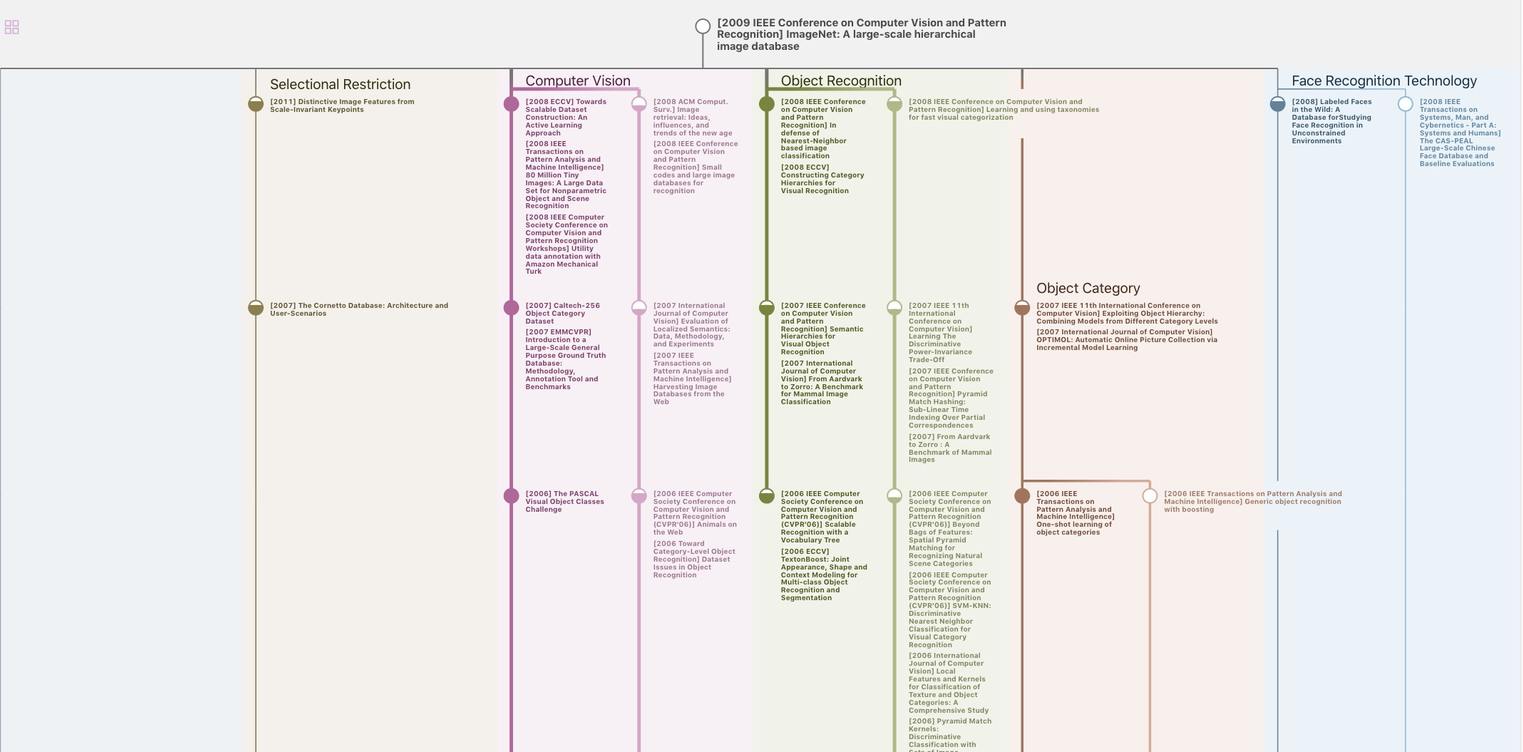
生成溯源树,研究论文发展脉络
Chat Paper
正在生成论文摘要