Large-Margin Determinantal Point Processes.
UAI(2015)
摘要
Determinantal point processes (DPPs) offer a powerful approach to modeling diversity in many applications where the goal is to select a diverse subset from a ground set of items. We study the problem of learning the parameters (i.e., the kernel matrix) of a DPP from labeled training data. In this paper, we develop a novel parameter estimation technique particularly tailored for DPPs based on the principle of large margin separation. In contrast to the state-of-the-art method of maximum likelihood estimation of the DPP parameters, our large-margin loss function explicitly models errors in selecting the target subsets, and it can be customized to trade off different types of errors (precision vs. recall). Extensive empirical studies validate our contributions, including applications on challenging document and video summarization, where flexibility in balancing different errors while training the summarization models is indispensable.
更多查看译文
AI 理解论文
溯源树
样例
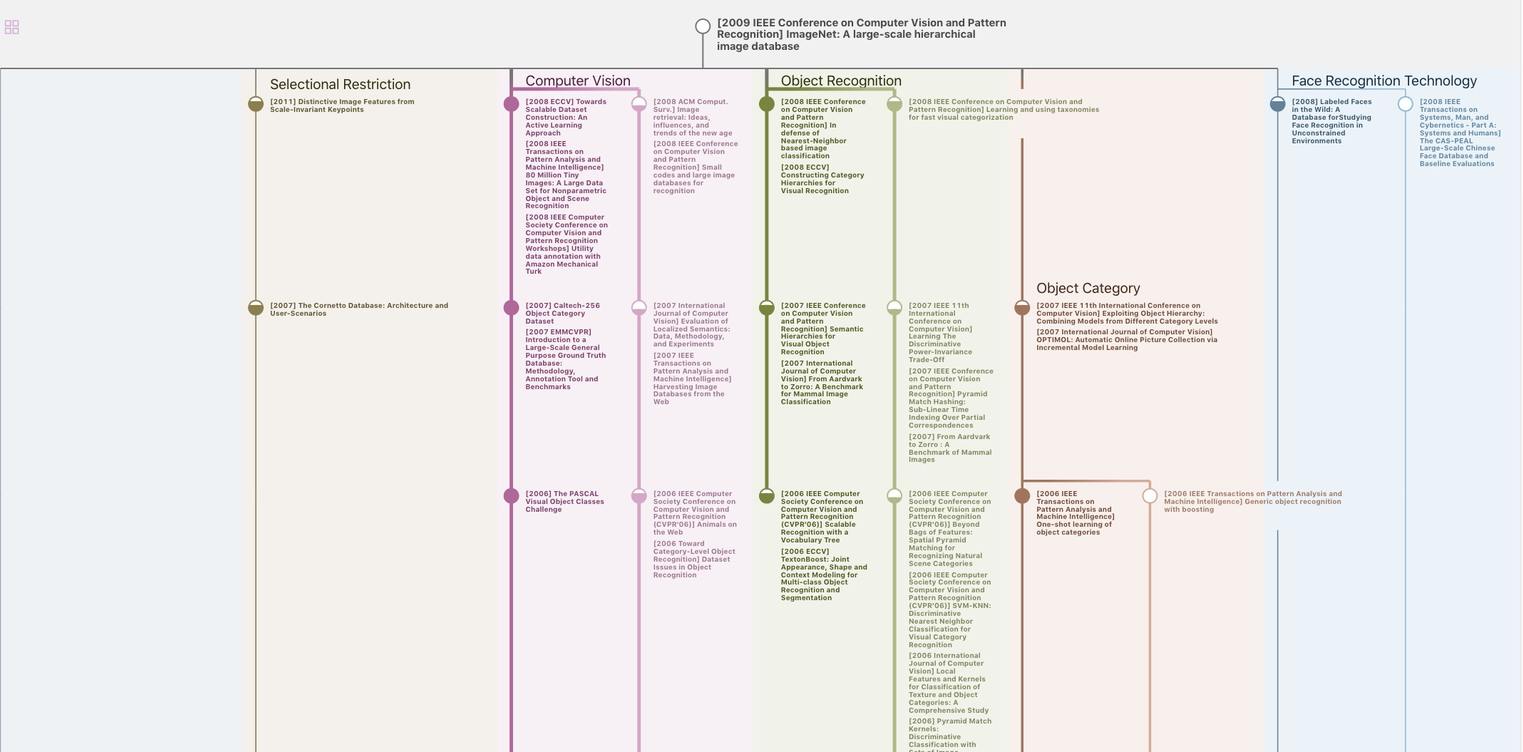
生成溯源树,研究论文发展脉络
Chat Paper
正在生成论文摘要