Adversarial Cost-Sensitive Classification
UAI'15: Proceedings of the Thirty-First Conference on Uncertainty in Artificial Intelligence(2015)
摘要
In many classification settings, mistakes incur different application-dependent penalties based on the predicted and actual class labels. Cost-sensitive classifiers minimizing these penalties are needed. We propose a robust minimax approach for producing classifiers that directly minimize the cost of mistakes as a convex optimization problem. This is in contrast to previous methods that minimize the empirical risk using a convex surrogate for the cost of mistakes, since minimizing the empirical risk of the actual cost-sensitive loss is generally intractable. By treating properties of the training data as uncertain, our approach avoids these computational difficulties. We develop theory and algorithms for our approach and demonstrate its benefits on cost-sensitive classification tasks.
更多查看译文
AI 理解论文
溯源树
样例
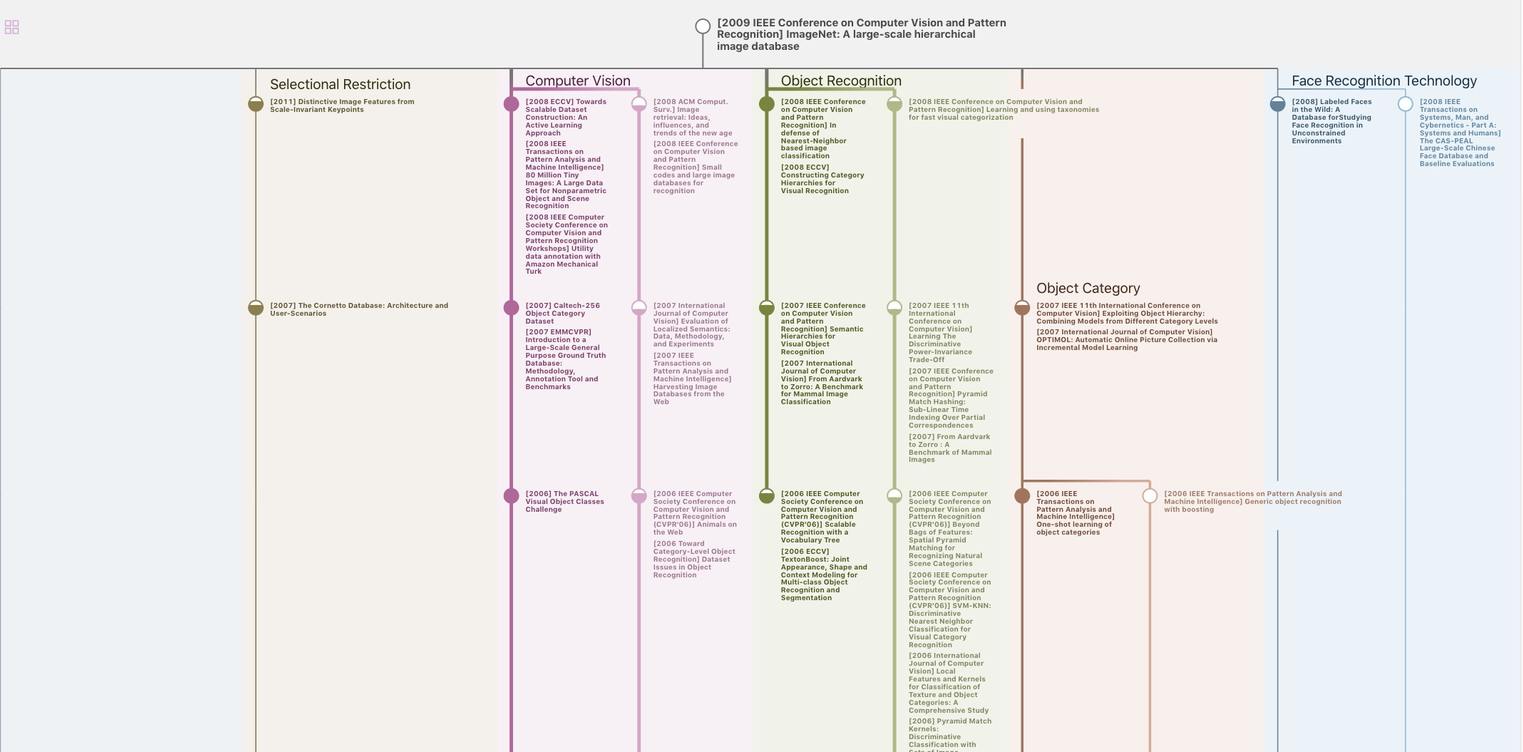
生成溯源树,研究论文发展脉络
Chat Paper
正在生成论文摘要