Experimental evaluation of indoor localization algorithms
2014 International Conference on Indoor Positioning and Indoor Navigation (IPIN)(2014)
摘要
In Radio Frequency (RF)-based indoor localization scenarios, localization algorithms are needed to alleviate the impact of non-line-of-sight and multipath effects on the measurements and thereby estimate the true position precisely. Several resilient lateration algorithms have been proposed in the last couple of years which claim to minimize these effects. However, most of these algorithms were only evaluated using simulations or small static testbeds. We conducted an experiment using 25 anchor nodes and a mobile node installed on top a robotic reference system to collect ranging values. The robot has a localization error of 6.5cm which is an order lower than our range measurement errors. We use this robot to collect range measurements and ground truth positions along a densely grid with approx. 10 cm spacing. The experiment was carried out in a hallway of our office-like building. We collected data on approx. 300 m
2
. First, we examine the influence of the anchor placement and anchor density on the ranging errors we see. Then, we evaluate and analyze the robustness of localization algorithms on our measured data to decide which one works best for a constellation of anchor placement and building. Our results show, that there are significant differences between the simulations published for lateration algorithms and actual experiments in real-world indoor localization scenarios. As we show in this paper, the distance measurement error distribution has a large influence on these algorithms.
更多查看译文
关键词
indoor localization algorithms,radio frequency,RF-based indoor localization scenarios,nonline-of-sight effects,multipath effects,lateration algorithms,anchor nodes,mobile node,robotic reference system,localization error,range measurement errors,ground truth positions,office-like building,anchor placement,anchor density,distance measurement error distribution
AI 理解论文
溯源树
样例
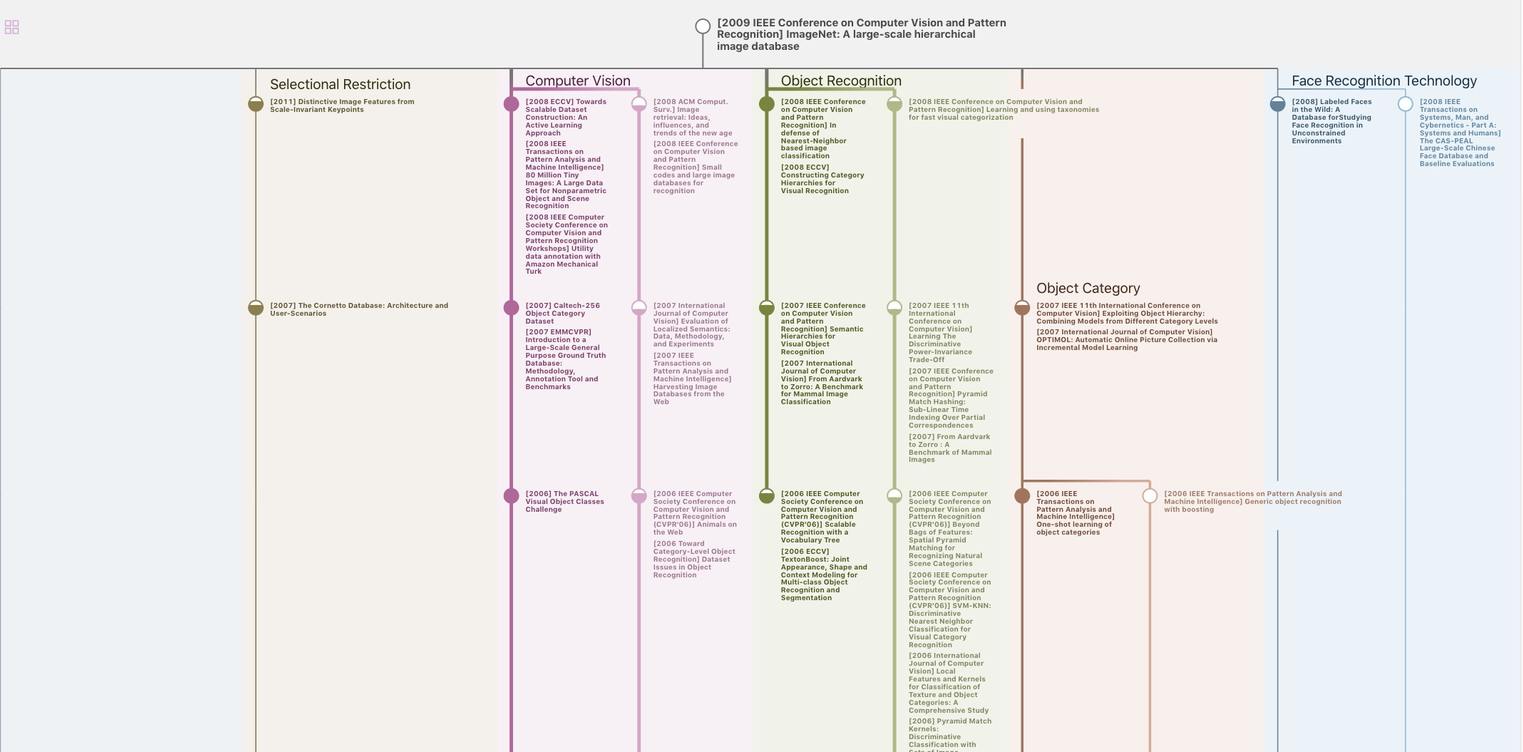
生成溯源树,研究论文发展脉络
Chat Paper
正在生成论文摘要