Drift Detection Using Stream Volatility
ECML/PKDD(2015)
摘要
Current methods in data streams that detect concept drifts in the underlying distribution of data look at the distribution difference using statistical measures based on mean and variance. Existing methods are unable to proactively approximate the probability of a concept drift occurring and predict future drift points. We extend the current drift detection design by proposing the use of historical drift trends to estimate the probability of expecting a drift at different points across the stream, which we term the expected drift probability. We offer empirical evidence that applying our expected drift probability with the state-of-the-art drift detector, ADWIN, we can improve the detection performance of ADWIN by significantly reducing the false positive rate. To the best of our knowledge, this is the first work that investigates this idea. We also show that our overall concept can be easily incorporated back onto incremental classifiers such as VFDT and demonstrate that the performance of the classifier is further improved.
更多查看译文
关键词
Data stream,Drift detection,Stream volatility
AI 理解论文
溯源树
样例
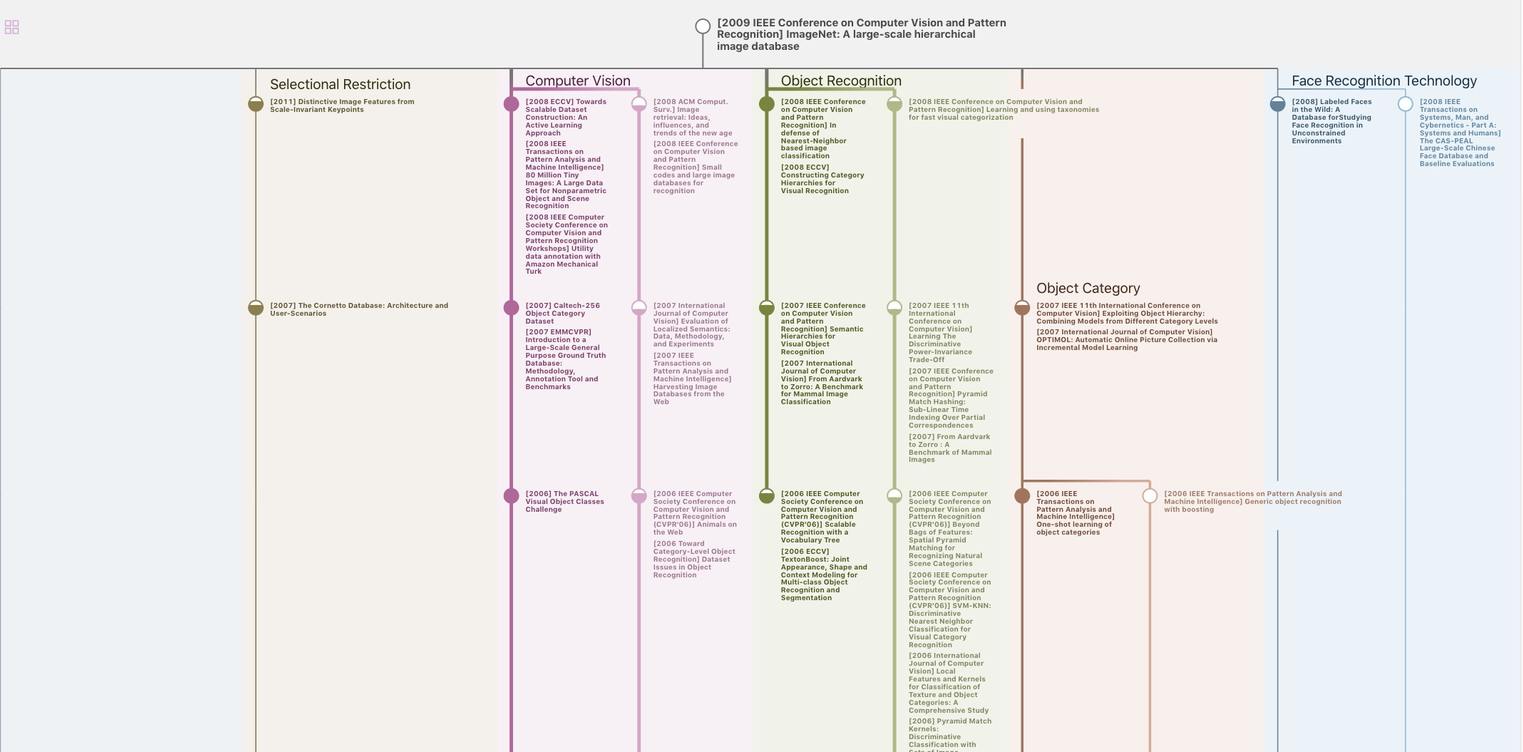
生成溯源树,研究论文发展脉络
Chat Paper
正在生成论文摘要