Input Data Adaptive Learning (IDAL) for Sub-acute Ischemic Stroke Lesion Segmentation.
Brainles@MICCAI(2015)
摘要
In machine learning larger databases are usually associated with higher classification accuracy due to better generalization. This generalization may lead to non-optimal classifiers in some medical applications with highly variable expressions of pathologies. This paper presents a method for learning from a large training base by adaptively selecting optimal training samples for given input data. In this way heterogeneous databases are supported two-fold. First, by being able to deal with sparsely annotated data allows a quick inclusion of new data set and second, by training an input-dependent classifier. The proposed approach is evaluated using the SISS challenge. The proposed algorithm leads to a significant improvement of the classification accuracy.
更多查看译文
关键词
Adaptive learning, Lesion segmentation, Machine learning, Random forest
AI 理解论文
溯源树
样例
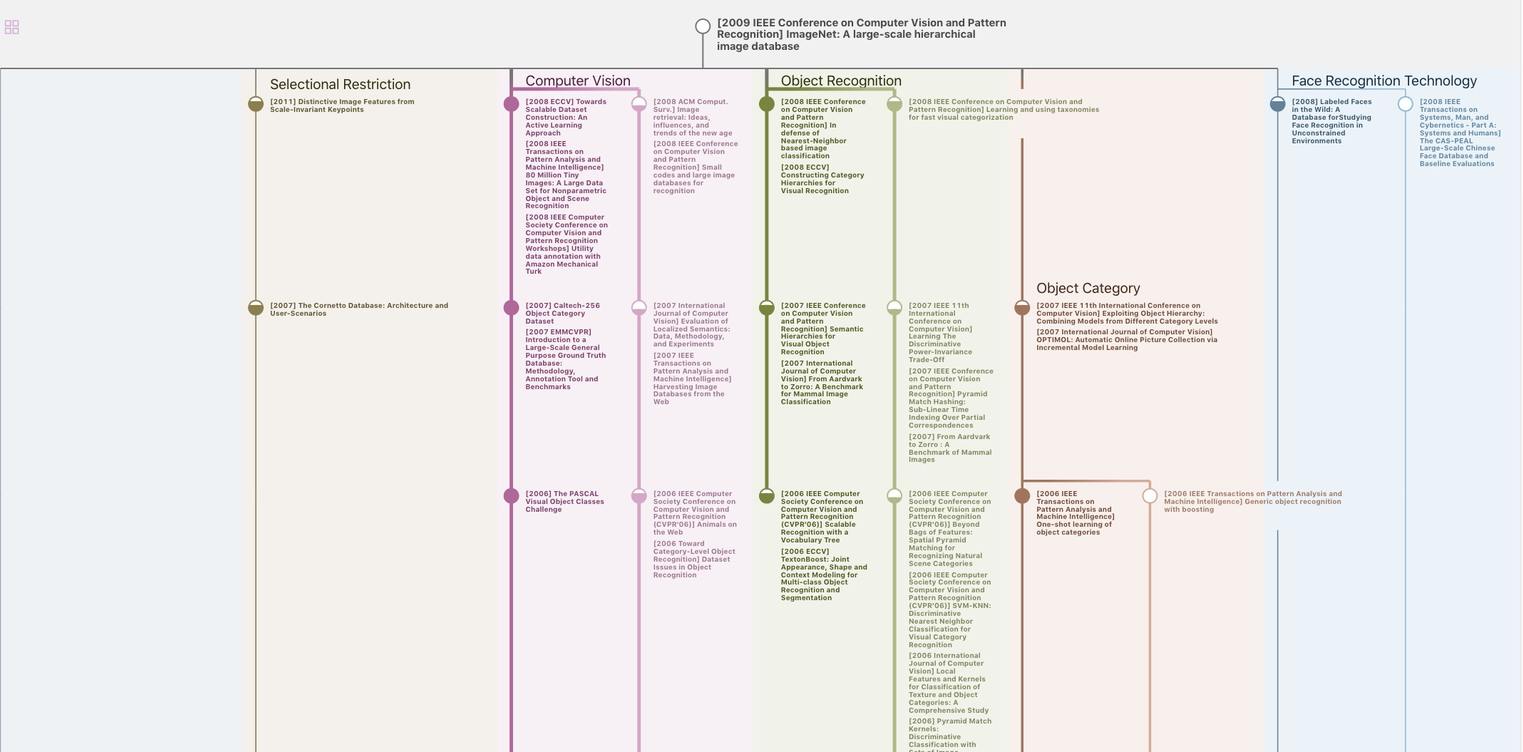
生成溯源树,研究论文发展脉络
Chat Paper
正在生成论文摘要