Unsupervised Network Anomaly Detection In Real-Time On Big Data
Springer eBooks(2015)
摘要
Network anomaly detection relies on intrusion detection systems based on knowledge databases. However, building this knowledge may take time as it requires manual inspection of experts. Actual detection systems are unable to deal with 0-day attack or new user's behavior and in consequence they may fail in correctly detecting intrusions. Unsupervised network anomaly detectors overcome this issue as no previous knowledge is required. In counterpart, these systems may be very slow as they need to learn traffic's pattern in order to acquire the necessary knowledge to detect anomalous flows. To improve speed, these systems are often only exposed to sampled traffic, harmful traffic may then avoid the detector examination. In this paper, we propose to take advantage of new distributed computing framework in order to speed up an Unsupervised Network Anomaly Detector Algorithm, UNADA. The evaluation shows that the execution time can be improved by a factor of 13 allowing UNADA to process large traces of traffic in real time.
更多查看译文
关键词
big data,real-time real-time,detection
AI 理解论文
溯源树
样例
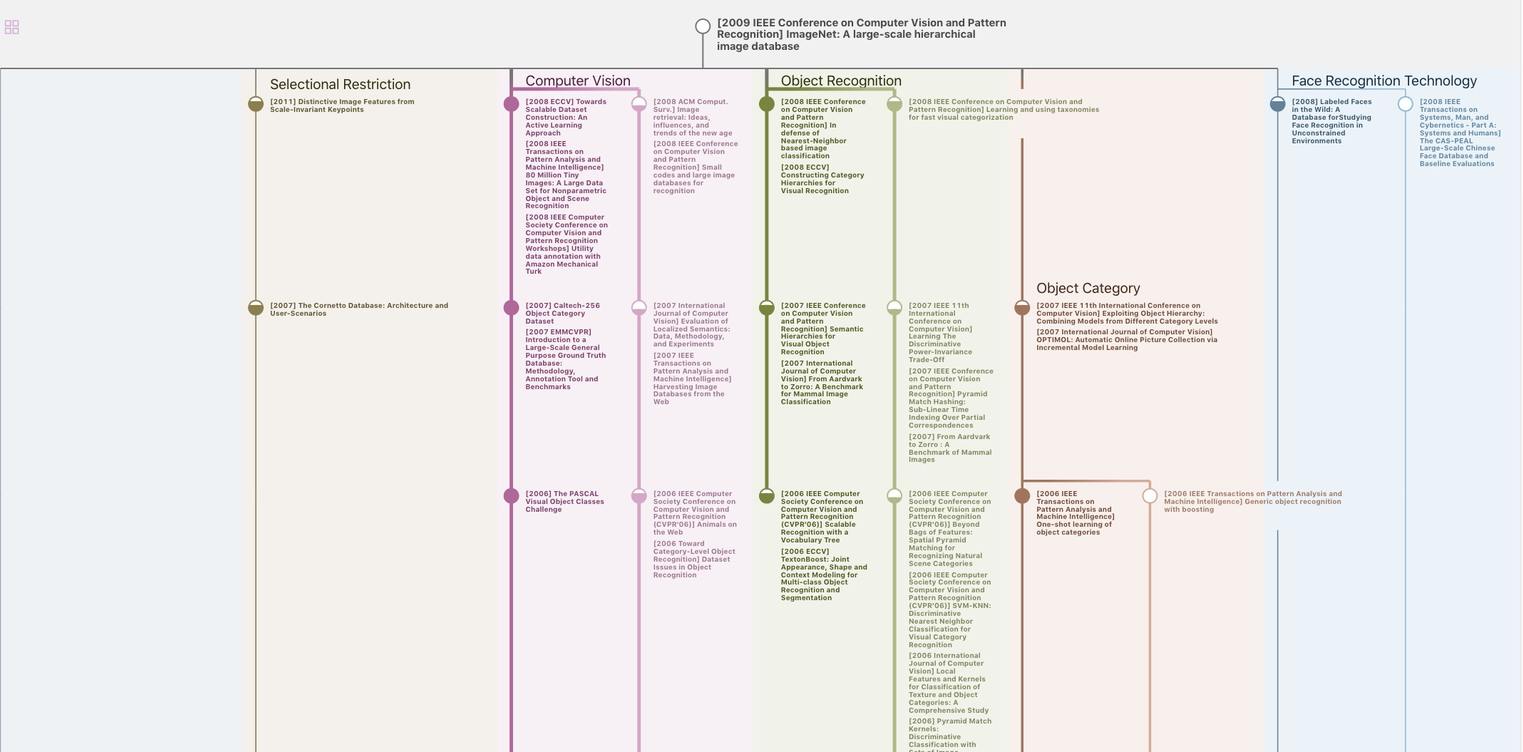
生成溯源树,研究论文发展脉络
Chat Paper
正在生成论文摘要