Towards Solving the Cold Start Transition Problem in Dynamic Recommender Systems.
ICEBE(2015)
摘要
The ability to model the tendency of both user preferences and product attractiveness is vital to the success of recommender systems, which is not covered well in the literature. It is challenging to learn a personalized tendency for recommender systems since users often leave feedback on an item only once and on only one period, i.e. The cold start transition problem. To tackle such a problem, we develop a novel probabilistic personalized and item-wise temporal model without prior assumptions on the structure of the dynamics, which solves the cold start transition problem by collaborative tendencies. The proposed method is evaluated on two benchmark datasets for recommender systems. The experimental results demonstrate that our proposed models significantly outperform a variety of existing methods for the top-k recommendation task.
更多查看译文
关键词
cold start transition problem,dynamic recommender system,user preference,product attractiveness,probabilistic personalized model,item-wise temporal model,collaborative tendency
AI 理解论文
溯源树
样例
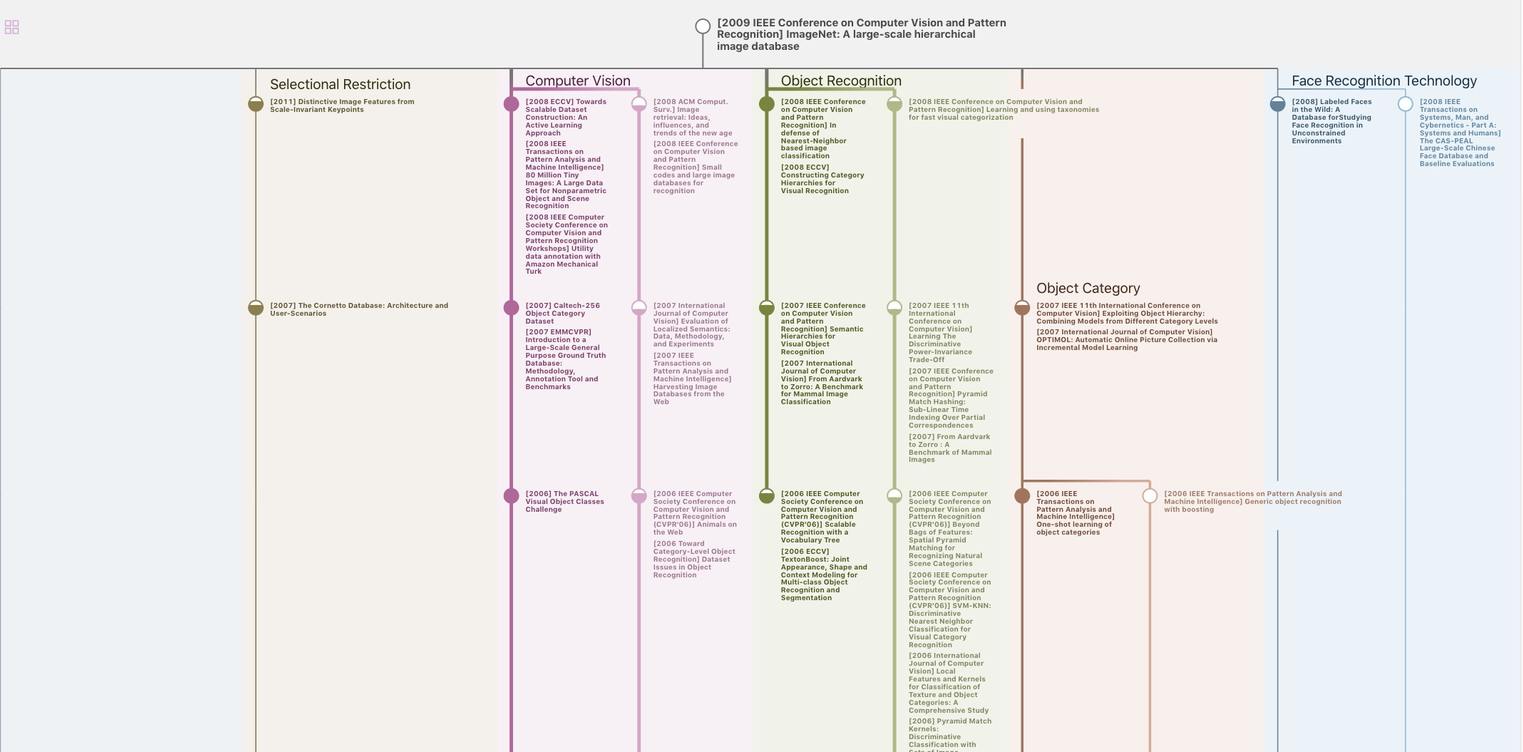
生成溯源树,研究论文发展脉络
Chat Paper
正在生成论文摘要