Improving data selection for low-resource STT and KWS
2015 IEEE Workshop on Automatic Speech Recognition and Understanding (ASRU)(2015)
摘要
This paper extends recent research on training data selection for speech transcription and keyword spotting system development. Selection techniques were explored in the context of the IARPA-Babel Active Learning (AL) task for 6 languages. Different selection criteria were considered with the goal of improving over a system built using a pre-defined 3-hour training data set. Four variants of the entropy-based criterion were explored: words, triphones, phones as well as the use of HMM-states previously introduced in [4]. The influence of the number of HMM-states was assessed as well as whether automatic or manual reference transcripts were used. The combination of selection criteria was investigated, and a novel multi-stage selection method proposed. This method was also assessed using larger data sets than were permitted in the Babel AL task. Results are reported for the 6 languages. The multi-stage selection was also applied to the surprise language (Swahili) in the NIST OpenKWS 2015 evaluation.
更多查看译文
关键词
data selection,low-resource languages,speech recognition,keyword spotting
AI 理解论文
溯源树
样例
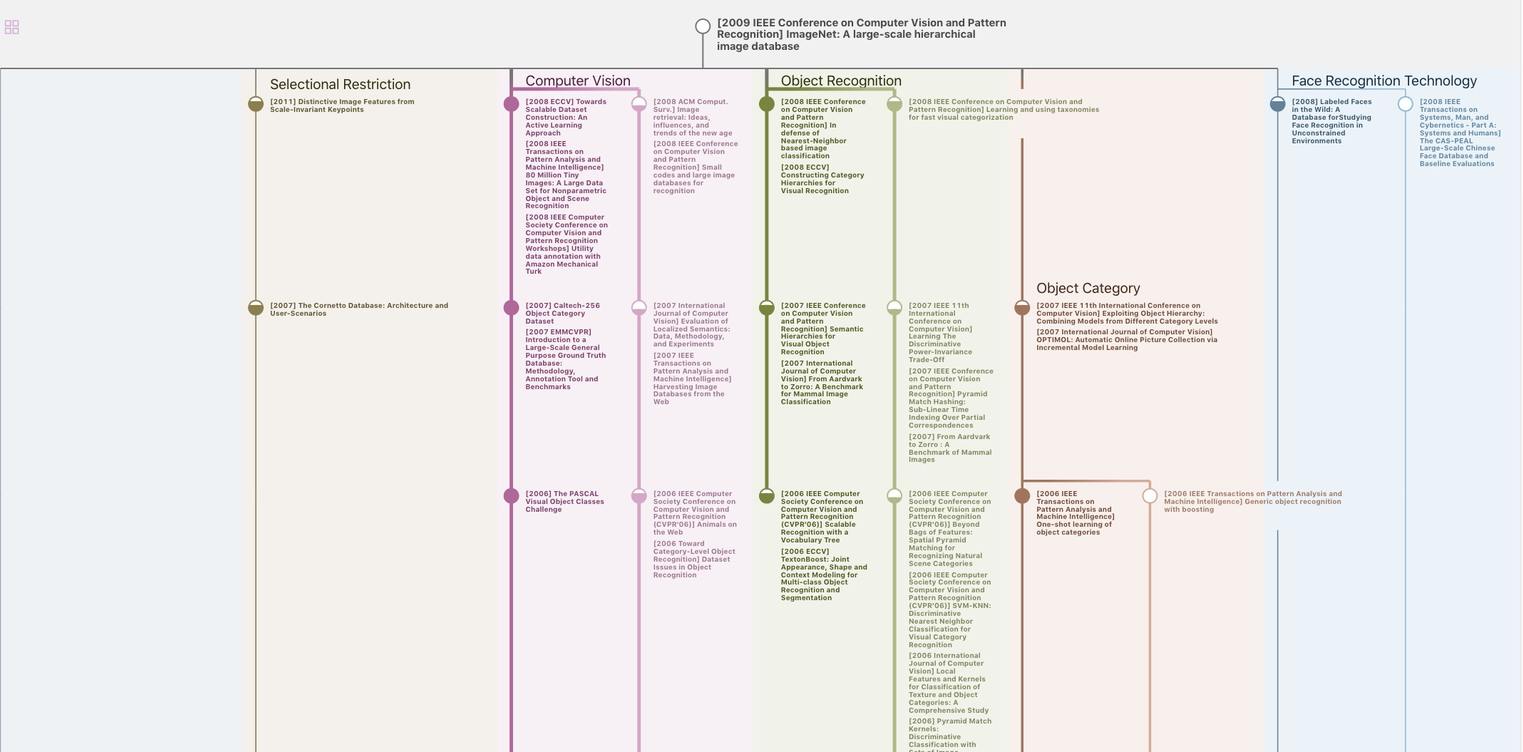
生成溯源树,研究论文发展脉络
Chat Paper
正在生成论文摘要