An Enhanced Support Vector Machine For Faster Time Series Classification
INTELLIGENT INFORMATION AND DATABASE SYSTEMS, ACIIDS 2016, PT I(2016)
摘要
As time series have become a prevalent type of data, various data mining tasks are often performed to extract useful knowledge, including time series classification, a more commonly performed task. Recently, a support vector machine (SVM), one of the most powerful classifiers for general classification tasks, has been applied to time series classification as it has been shown to outperform one nearest neighbor in various domains. Recently, Dynamic Time Warping (DTW) distance measure has been used as a feature for SVM classifier. However, its main drawback is its exceedingly high time complexity in DTW computation, which degrades the SVM's performance. In this paper, we propose an enhanced SVM that utilizes a DTW's fast lower bound function as its feature to mitigate the problem. The experiment results on 47 UCR time series data mining datasets demonstrate that our proposed work can speed up the classification process by a large margin while maintaining high accuracies comparing with the state-of-the-art approach.
更多查看译文
关键词
Classification, Support vector machine (SVM), Dynamic time warping (DTW), Lower bound, LB_keogh
AI 理解论文
溯源树
样例
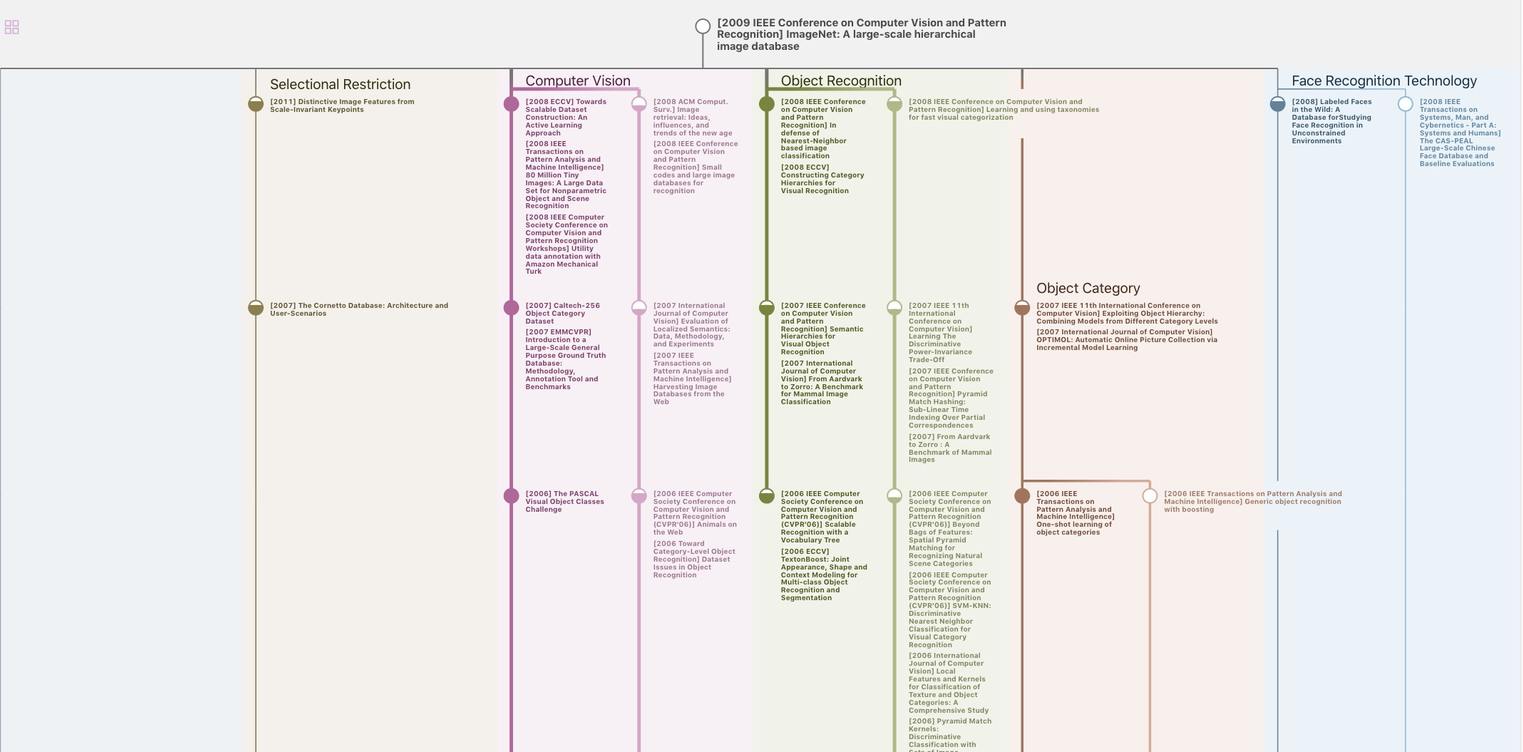
生成溯源树,研究论文发展脉络
Chat Paper
正在生成论文摘要