An Upper Bound On The Error Of Alignment-Based Transfer Learning Between Two Linear, Time-Invariant, Scalar Systems
2015 IEEE/RSJ International Conference on Intelligent Robots and Systems (IROS)(2015)
摘要
Methods from machine learning have successfully been used to improve the performance of control systems in cases when accurate models of the system or the environment are not available. These methods require the use of data generated from physical trials. Transfer Learning (TL) allows for this data to come from a different, similar system. This paper studies a simplified TL scenario with the goal of understanding in which cases a simple, alignment-based transfer of data is possible and beneficial. Two linear, time-invariant (LTI), single-input, single-output systems are tasked to follow the same reference signal. A scalar, LTI transformation is applied to the output from a source system to align with the output from a target system. An upper bound on the 2-norm of the transformation error is derived for a large set of reference signals and is minimized with respect to the transformation scalar. Analysis shows that the minimized error bound is reduced for systems with poles that lie close to each other (that is, for systems with similar response times). This criterion is relaxed for systems with poles that have a larger negative real part (that is, for stable systems with fast response), meaning that poles can be further apart for the same minimized error bound. Additionally, numerical results show that using the reference signal as input to the transformation reduces the minimized bound further.
更多查看译文
关键词
alignment-based transfer learning,linear time-invariant systems,scalar systems,machine learning,control systems,simplified TL scenario,LTI transformation,reference signals,transformation scalar
AI 理解论文
溯源树
样例
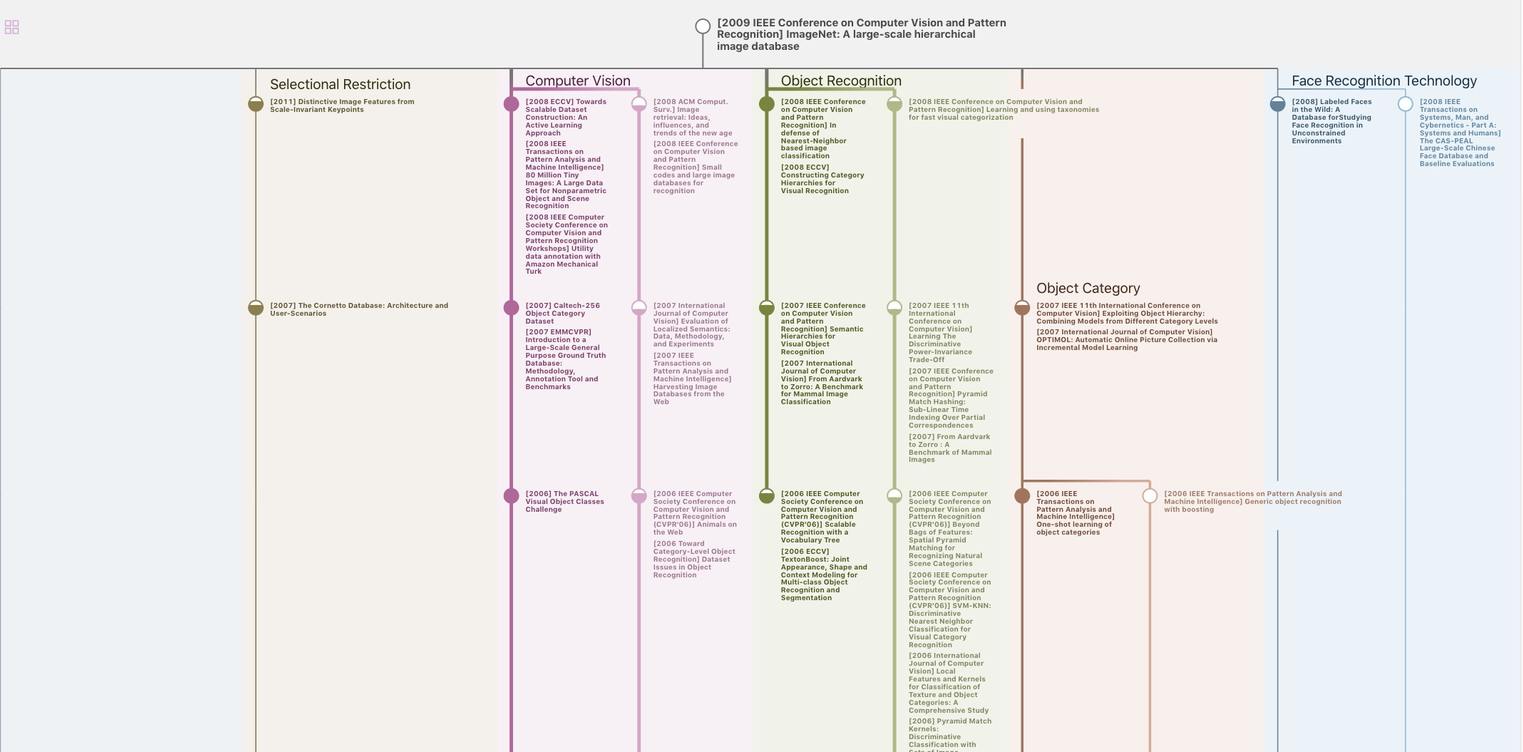
生成溯源树,研究论文发展脉络
Chat Paper
正在生成论文摘要