Parametric Covariance Prediction For Heteroscedastic Noise
2015 IEEE/RSJ International Conference on Intelligent Robots and Systems (IROS)(2015)
摘要
The ubiquitous additive Gaussian noise model is favored in statistical modeling applications for its flexibility and ease of use. Often noise is assumed to be well-represented by a constant covariance, while in reality error characteristics may change predictably. We present an efficient parametric covariance predictor based on the modified Cholesky decomposition that maps from features of the input to covariance matrices. In addition, we discuss fitting the predictor parameters using noise samples with simple regularization techniques. We demonstrate our approach by estimating observation covariances for range-bearing localization with simulated and experimental datasets and show that this results in increased filtering performance compared to traditional covariance adaptation and constant covariance baselines.
更多查看译文
关键词
parametric covariance prediction,heteroscedastic noise,ubiquitous additive Gaussian noise model,statistical modeling,Cholesky decomposition,covariance matrices,regularization technique,range-bearing localization
AI 理解论文
溯源树
样例
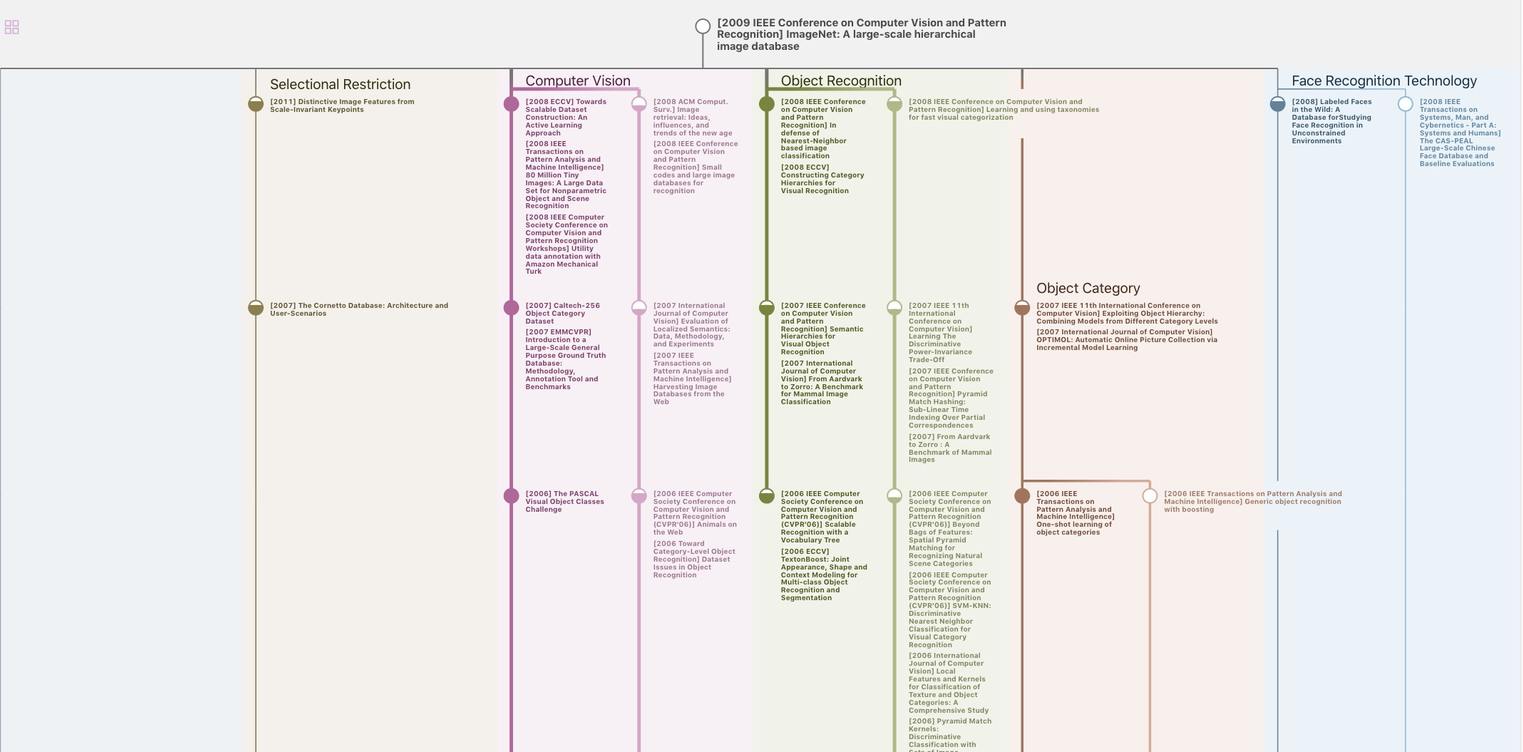
生成溯源树,研究论文发展脉络
Chat Paper
正在生成论文摘要