A Framework for Simplifying Trip Data into Networks via Coupled Matrix Factorization.
SDM(2015)
摘要
Portable devices such as GPS-equipped smart phones and cameras are able to provide detailed spatiotemporal trip event data for each user. Such data can be aggregated over many users to provide large amounts of behavioral data of very fine granularity. Trying to simplify this data into meaningful higher-level insights is challenging for a variety of reasons. In this paper we study the problem of simplifying spatio-temporal trip data and summarizing them into an easily interpretable graph/network. We propose several constrained coupled nonnegative matrix factorization formulations that simultaneously cluster locations and times based on the associated trips, and develop a (block) coordinate descent algorithm to solve them. We empirically evaluate our approach on a real world data set of taxis’ GPS traces and show the advantages of our approach over traditional clustering algorithms.
更多查看译文
AI 理解论文
溯源树
样例
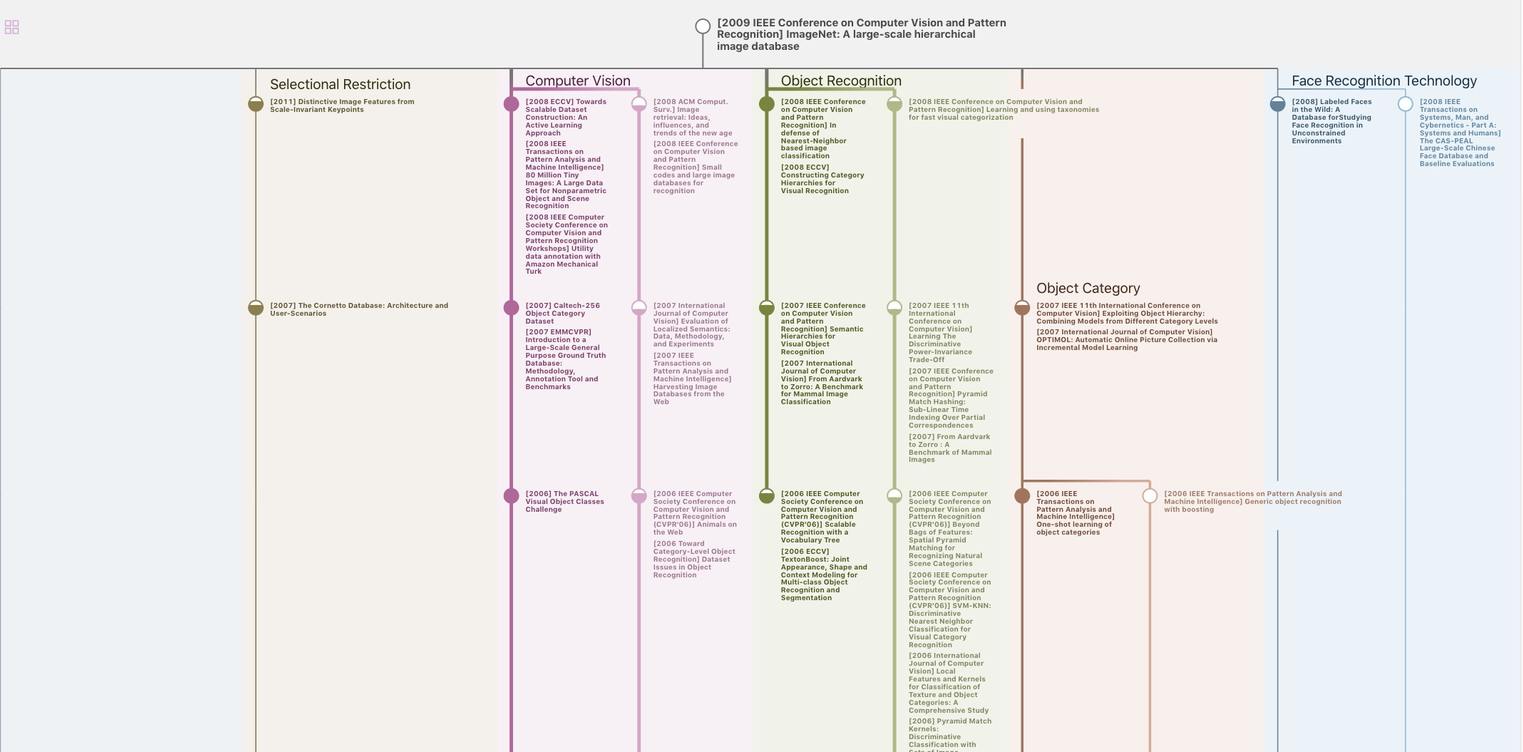
生成溯源树,研究论文发展脉络
Chat Paper
正在生成论文摘要