Surpassing Humans and Computers with JELLYBEAN: Crowd-Vision-Hybrid Counting Algorithms
HCOMP(2015)
摘要
Counting objects is a fundamental image processisng primitive, and has many scientific, health, surveillance, security, and military applications. Existing supervised computer vision techniques typically require large quantities of labeled training data, and even with that, fail to return accurate results in all but the most stylized settings. Using vanilla crowd-sourcing, on the other hand, can lead to significant errors, especially on images with many objects. In this paper, we present our JellyBean suite of algorithms, that combines the best of crowds and computer vision to count objects in images, and uses judicious decomposition of images to greatly improve accuracy at low cost. Our algorithms have several desirable properties: they are , in that they ask as few questions as possible to humans (under certain intuitively reasonable assumptions that we justify in our paper experimentally); they operate under or modes, in that they can either work independent of computer vision algorithms, or work in concert with them, depending on whether the computer vision techniques are available or useful for the given setting; they in practice, returning accurate counts on images that no individual worker or computer vision algorithm can count correctly, while not incurring a high cost.
更多查看译文
AI 理解论文
溯源树
样例
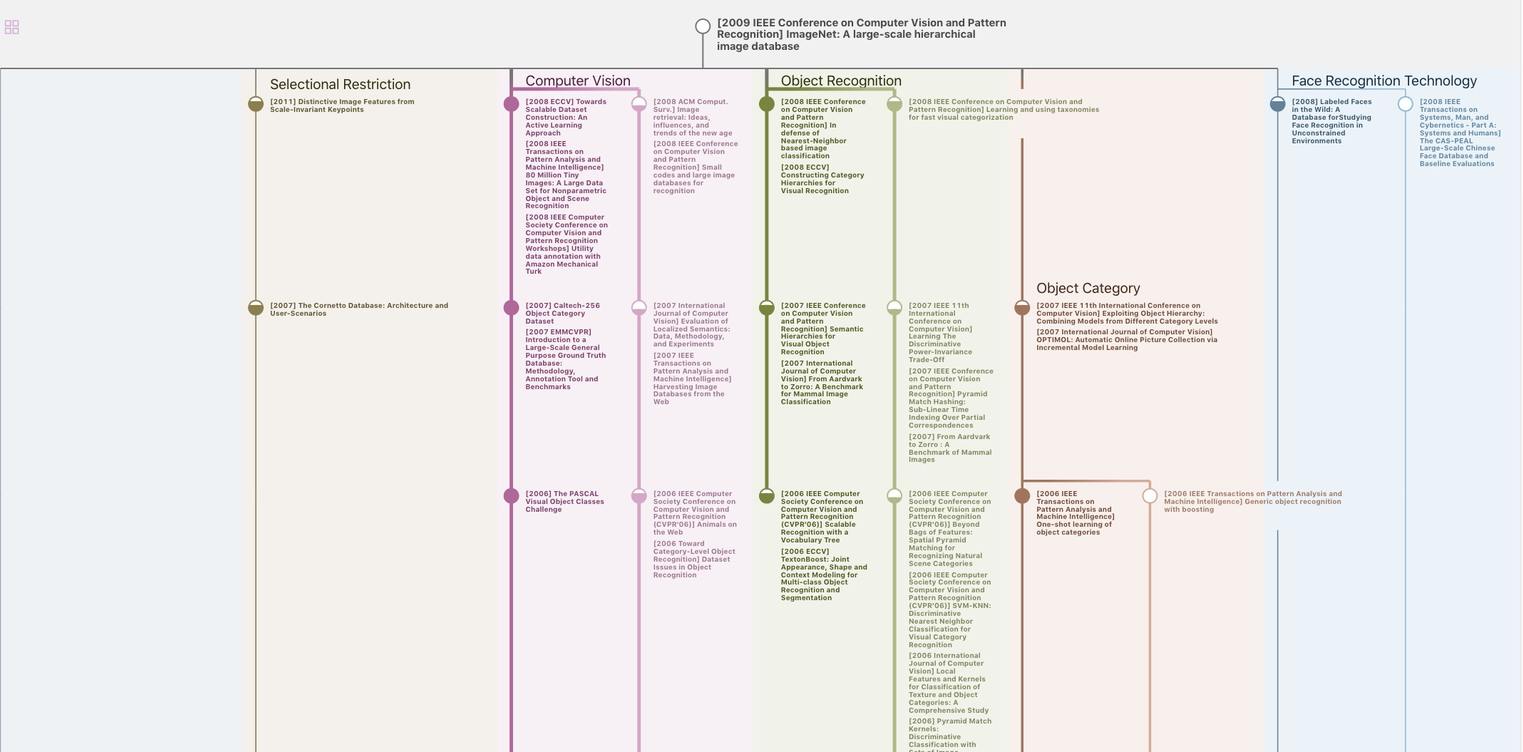
生成溯源树,研究论文发展脉络
Chat Paper
正在生成论文摘要