Probabilistic Inference on Integrity for Access Behavior Based Malware Detection.
RAID(2015)
摘要
Integrity protection has proven an effective way of malware detection and defense. Determining the integrity of subjects programs and objects files and registries plays a fundamental role in integrity protection. However, the large numbers of subjects and objects, and intricate behaviors place burdens on revealing their integrities either manually or by a set of rules. In this paper, we propose a probabilistic model of integrity in modern operating system. Our model builds on two primary security policies, \"no read down\" and \"no write up\", which make connections between observed access behaviors and the inherent integrity ordering between pairs of subjects and objects. We employ a message passing based inference to determine the integrity of subjects and objects under a probabilistic graphical model. Furthermore, by leveraging a statistical classifier, we build an integrity based access behavior model for malware detection. Extensive experimental results on a real-world dataset demonstrate that our model is capable of detecting 7,257 malware samples from 27,840 benign processes at 99.88﾿% true positive rate under 0.1﾿% false positive rate. These results indicate the feasibility of our probabilistic integrity model.
更多查看译文
关键词
Probabilistic graphical model, Integrity protection, Malware
AI 理解论文
溯源树
样例
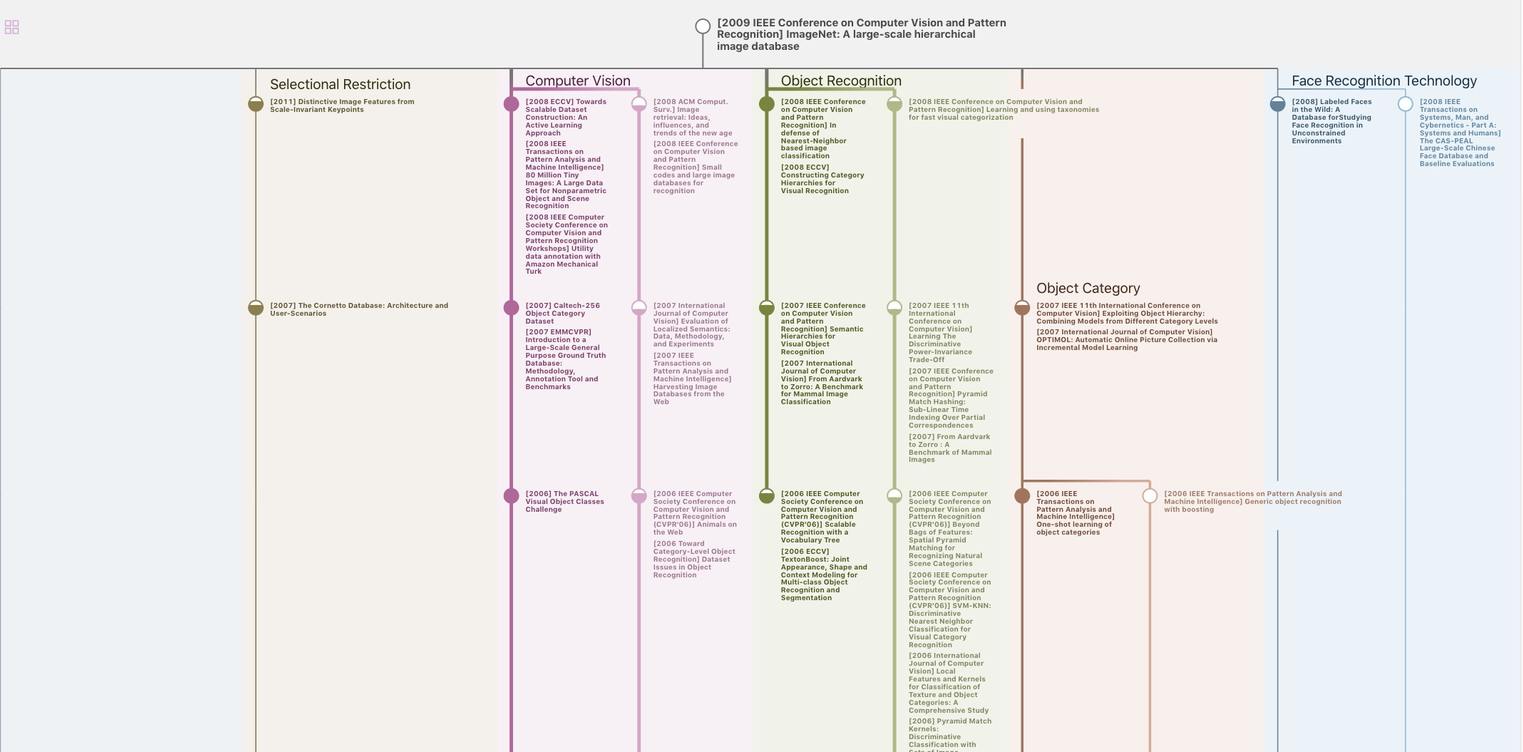
生成溯源树,研究论文发展脉络
Chat Paper
正在生成论文摘要