Probabilistic learning from mislabelled data for multimedia content recognition
2015 IEEE International Conference on Multimedia and Expo (ICME)(2015)
摘要
There have been considerable advances in multimedia recognition recently as powerful computing capabilities and large, representative datasets become ubiquitous. A fundamental assumption of traditional recognition techniques is that the data available for training are accurately labelled. Given the scale and diversity of web data, it takes considerable annotation effort to reduce label noise to acceptable levels. In this work, we propose a novel method to work around this issue by utilizing approximate apriori estimates of the mislabelling probabilities to design a noise-aware learning framework. We demonstrate the proposed framework's effectiveness on several datasets of various modalities and show that it is able to achieve high levels of accuracy even when faced with significant mislabelling in the data.
更多查看译文
关键词
multimedia content recognition,mislabelled data,probabilistic learning
AI 理解论文
溯源树
样例
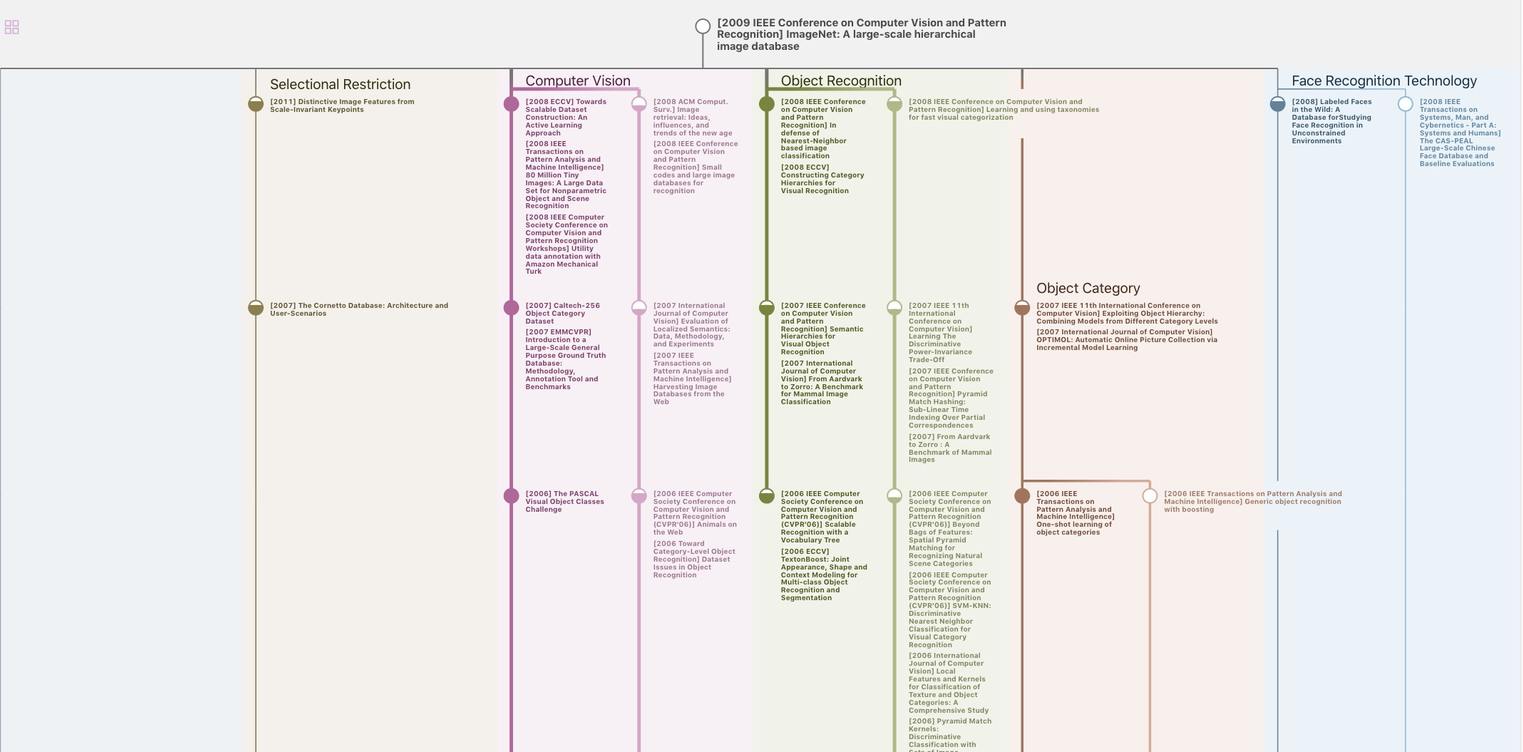
生成溯源树,研究论文发展脉络
Chat Paper
正在生成论文摘要