Causality graph learning on cortical information flow in Parkinson's disease patients during behaviour tests
2015 49TH ASILOMAR CONFERENCE ON SIGNALS, SYSTEMS AND COMPUTERS(2015)
摘要
Electroencephalographs (EEG) signals of the human brains represent electrical activities for a number of channels recorded over a the scalp. The main purpose of this paper is to investigate the interactions and causality of different parts of a brain using EEG signals recorded during a performance subjects of verbal fluency tasks. Subjects who have Parkinson's Disease (PD) have difficulties with mental tasks, such as switching between one behavior task and another. The behavior tasks include motor and phonemic fluency. This method uses verbal generation skills, activating different Broca's areas of the Brodmann's areas (BA44 and BA45). Advanced signal processing techniques are used in order to determine the activated frequency bands in the granger causality for verbal fluency tasks. The graph learning technique for channel strength is used to characterize the complex graph of Granger causality. Also, the support vector machine (SVM) method is used for training a classifier between two subjects with PD and two healthy controls. Neural data from the study was recorded at the Colorado Neurological Institute (CNI).
更多查看译文
关键词
causality graph learning,cortical information flow,Parkinson disease patients,behaviour test,electroencephalograph signal,EEG signal,electrical activities,scalp,brain,verbal fluency task,PD,mental task,motor fluency,phonemic fluency,verbal generation skills,Broca areas,Brodmann areas,BA44,BA45,advanced signal processing technique,activated frequency band,granger causality,channel strength,support vector machine method,SVM method,classifier training,Colorado Neurological Institute,CNI
AI 理解论文
溯源树
样例
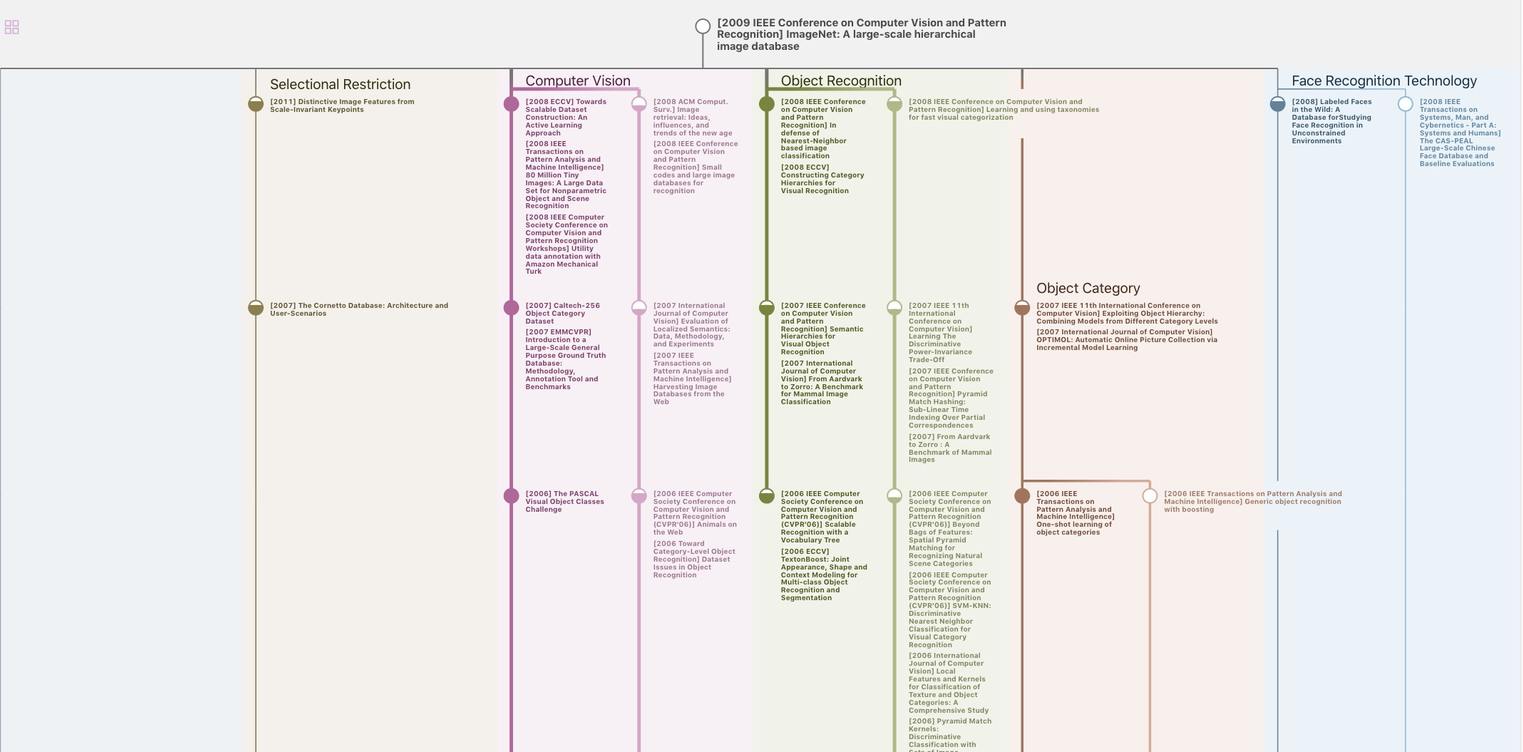
生成溯源树,研究论文发展脉络
Chat Paper
正在生成论文摘要