A mixed model for cross lingual opinion analysis
Communications in Computer and Information Science(2013)
摘要
The performances of machine learning based opinion analysis systems are always puzzled by the insufficient training opinion corpus. Such problem becomes more serious for the resource-poor languages. Thus, the cross-lingual opinion analysis (CLOA) technique, which leverages opinion resources on one (source) language to another (target) language for improving the opinion analysis on target language, attracts more research interests. Currently, the transfer learning based CLOA approach sometimes falls to over fitting on single language resource, while the performance of the co-training based CLOA approach always achieves limited improvement during bi-lingual decision. Target to these problems, in this study, we propose a mixed CLOA model, which estimates the confidence of each monolingual opinion analysis system by using their training errors through bilingual transfer self-training and co-training, respectively. By using the weighted average distances between samples and classification hyper-planes as the confidence, the opinion polarity of testing samples are classified. The evaluations on NLP&CC 2013 CLOA bakeoff dataset show that this approach achieves the best performance, which outperforms transfer learning and co-training based approaches. © Springer-Verlag Berlin Heidelberg 2013.
更多查看译文
关键词
Cross lingual Opinion Analysis,Transfer Self-Training,Co-Training,Mixed Model
AI 理解论文
溯源树
样例
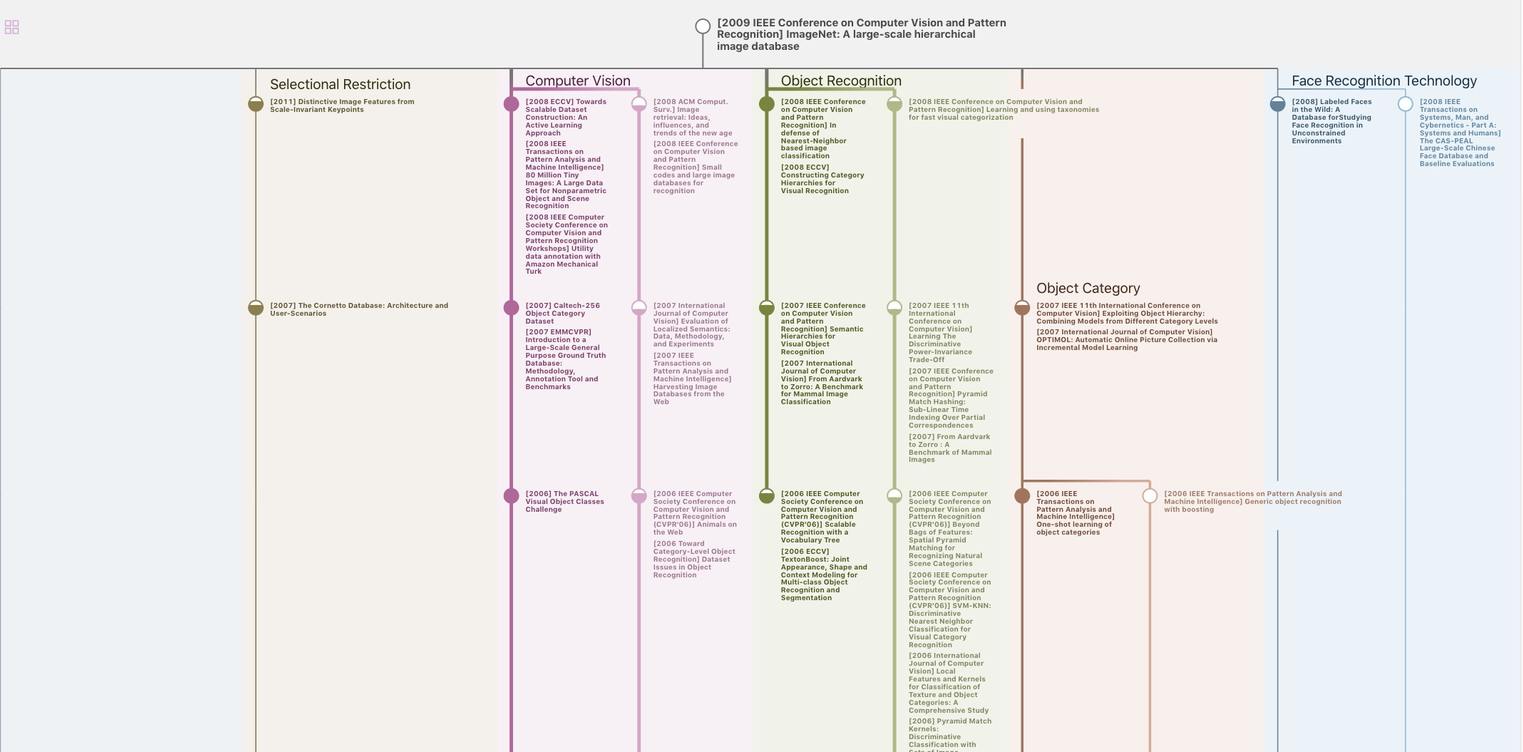
生成溯源树,研究论文发展脉络
Chat Paper
正在生成论文摘要