Stock Price Prediction in Undirected Graphs Using a Structural Support Vector Machine.
WI-IAT(2015)
摘要
Business analytics techniques help mine and analyze business/financial data. For instance, a structural support vector machine (SSVM) can be used to perform classification on complex inputs such as the nodes of a graph structure. We connect collaborating companies in the information technology sector in an undirected graph and use an SSVM to predict positive or negative movement in their stock prices. By using a minimum graph-cutting algorithm to drive the cutting plane optimization problem of the SSVM, an exact solution is achieved in polynomial time. The learned model exploits the associative relationship between the prices of the collaborating companies to outperform the accuracy of a regular SVM. Experiments were conducted using the companies in the Standard and Poor's 500-45 Information Technology Sector index. Trades based on the learned model achieved superior returns in the range of 10% to 17% while tracking the index alone over the same time periods yielded returns in the range of -17% to 9%.
更多查看译文
关键词
Web intelligence, structural support vector machine (SSVM), minimum graph-cuts, graph labeling, finance, stock price prediction
AI 理解论文
溯源树
样例
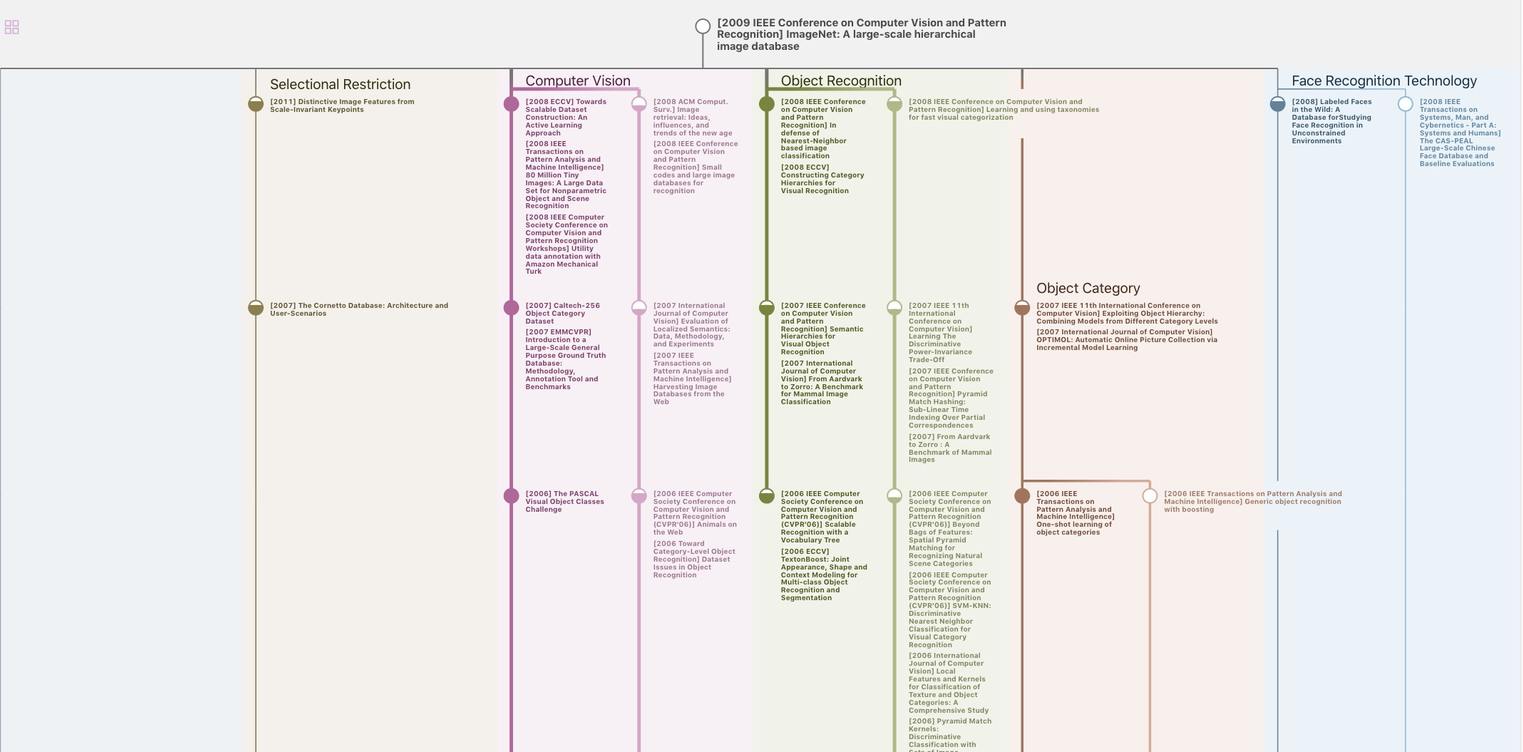
生成溯源树,研究论文发展脉络
Chat Paper
正在生成论文摘要