Two-Level Multi-Task Metric Learning With Application To Multi-Classification
2015 IEEE International Conference on Image Processing (ICIP)(2015)
摘要
Many metric learning approaches neglect that the real world multi-class problems share strong visual similarities, which can be exploited by learning discriminative models. In this paper, a Two-level Multi-task Metric Learning (TMTL) method is presented to learn a distance measure from equivalence constraints. Multiple features are adopted to represent the image information and learn the distance matrices in the first level. Then the task-specific learning paradigm and multi-task voting mechanism make full use of pairwise equivalence labels, which induces knowledge from anonymous pairs to multi-classification. Experiments are conducted on two challenging benchmarks PubFig and OuluVS for face identification and lipreading respectively. The results demonstrate that our method outperforms the recent multi-task learning approaches and multi-class support vector machine.
更多查看译文
关键词
Metric Learning,Multi-task Learning,Face Identification,Lipreading
AI 理解论文
溯源树
样例
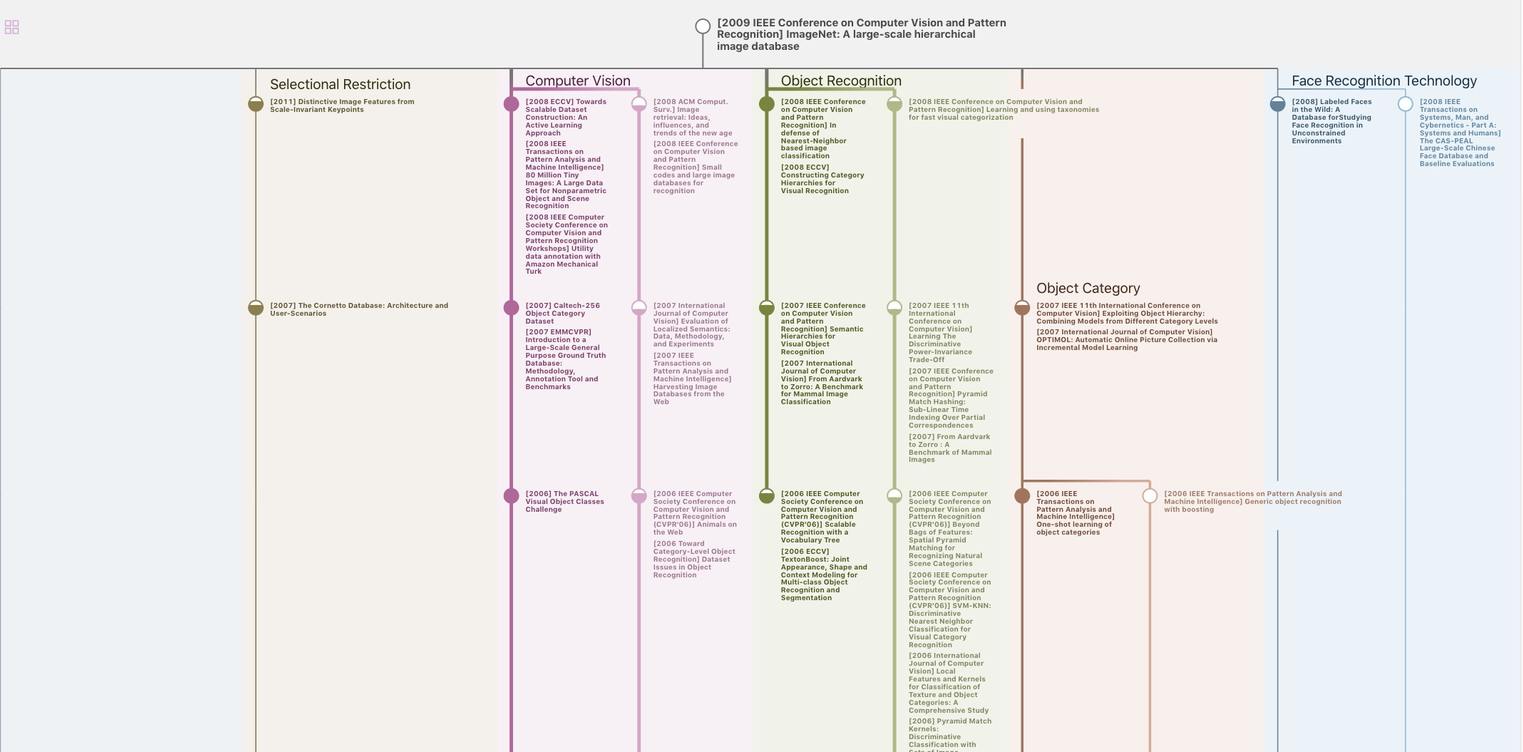
生成溯源树,研究论文发展脉络
Chat Paper
正在生成论文摘要