Privacy-Preserving Mining of Frequent Routes in Cellular Network Data
TrustCom/BigDataSE/ISPA(2015)
摘要
Cellular networks generate a wealth of mobility data that may be applied in numerous application areas such as traffic and transport management, urban planning and crisis management. Due to the sheer size of network data, and since it can contain sensitive information, this potential also comes with great technical challenges with regard to information extraction, data reduction and privacy. In this paper we simultaneously address these three challenges with respect to the specific task of mining frequent routes of terminals and, in extension, people and vehicles. The proposed approach is a pipeline where raw cell data is segmented into sequences that, in turn, are aggregated into groups of similar sequences using a scalable distributed clustering algorithm based on locality-sensitive hashing. Acquired aggregate statistics are then perturbed using an existing differential-privacy framework in order to ensure that sensitive information is not released. This compound approach has been evaluated with respect to cluster quality, data reduction and privacy protection, on coarse empirical call detail record data, as well as on more fine-grained synthetic handover data simulated from measured GPS traces.
更多查看译文
关键词
privacy-preserving mining,frequent route mining,cellular network data,mobility data,information extraction,data reduction,distributed clustering algorithm,locality-sensitive hashing,differential-privacy framework,privacy protection
AI 理解论文
溯源树
样例
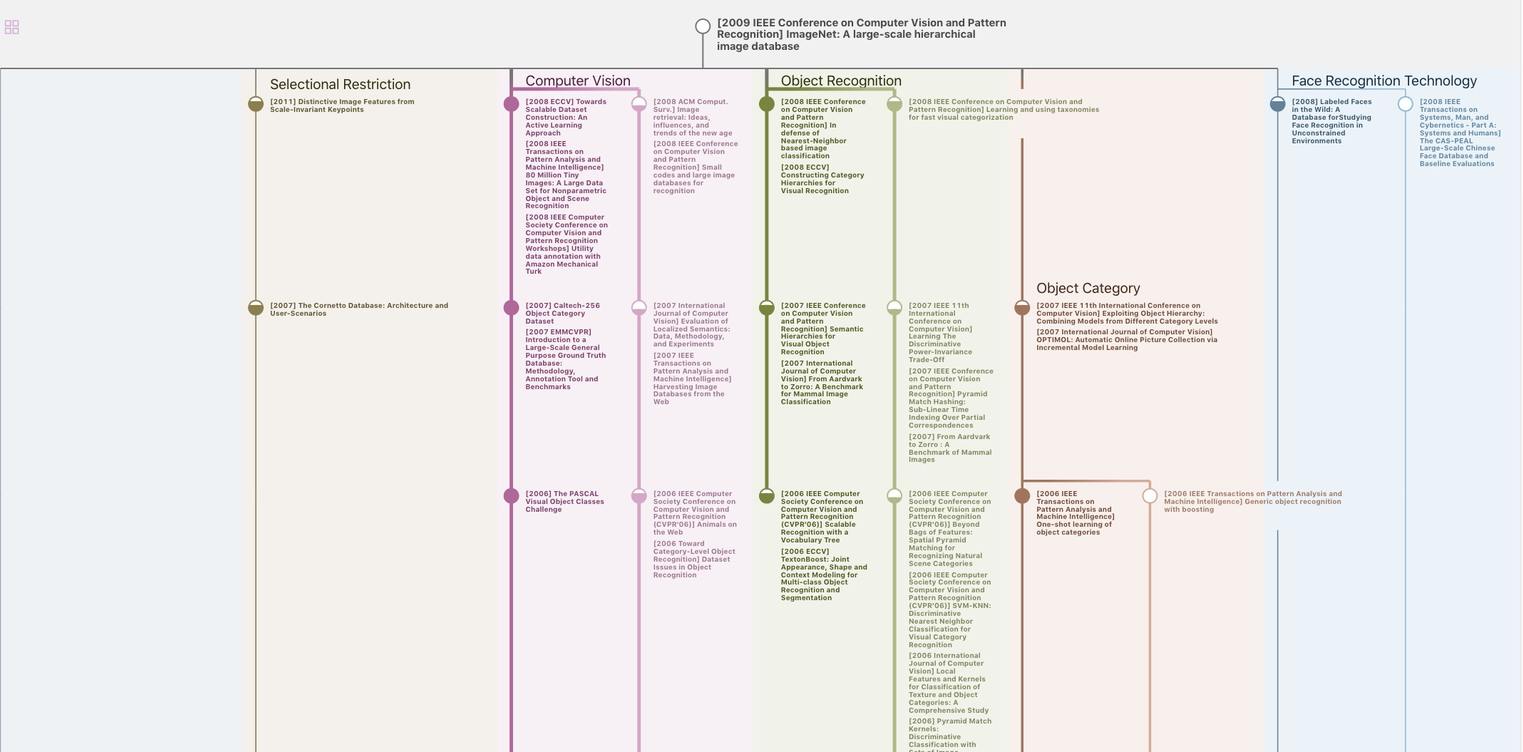
生成溯源树,研究论文发展脉络
Chat Paper
正在生成论文摘要