Efficient Detection of Zero-day Android Malware Using Normalized Bernoulli Naive Bayes
TrustCom/BigDataSE/ISPA(2015)
摘要
According to a recent F-Secure report, 97% of mobile malware is designed for the Android platform which has a growing number of consumers. In order to protect consumers from downloading malicious applications, there should be an effective system of malware classification that can detect previously unseen viruses. In this paper, we present a scalable and highly accurate method for malware classification based on features extracted from Android application package (APK) files. We explored several techniques for tackling independence assumptions in Naive Bayes and proposed Normalized Bernoulli Naive Bayes classifier that resulted in an improved class separation and higher accuracy. We conducted a set of experiments on an up-to-date large dataset of APKs provided by F-Secure and achieved 0.1% false positive rate with overall accuracy of 91%.
更多查看译文
关键词
Malware Classification, Naive Bayes, Security in Android
AI 理解论文
溯源树
样例
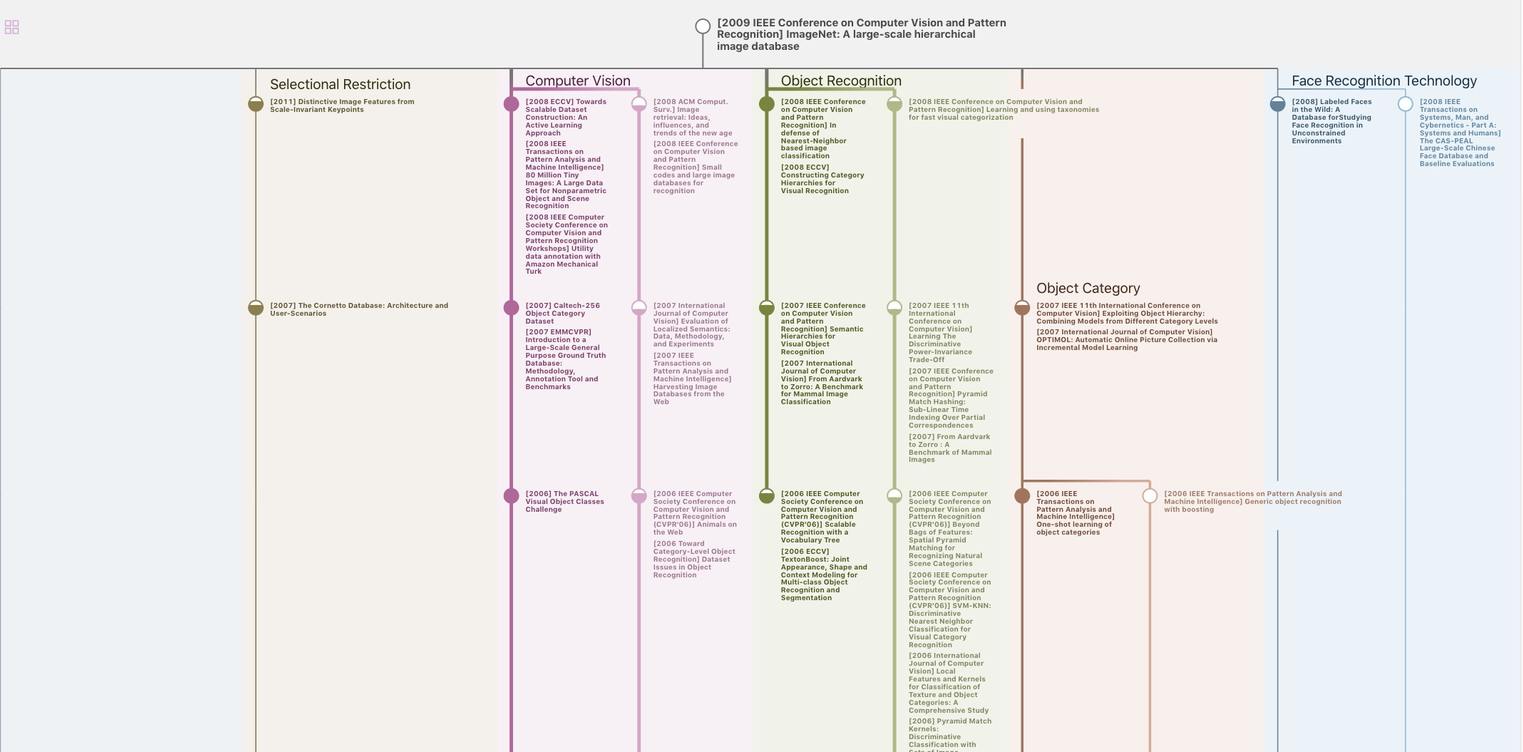
生成溯源树,研究论文发展脉络
Chat Paper
正在生成论文摘要